From Pavlov Conditioning to Hebb Learning.
NEURAL COMPUTATION(2023)
摘要
Hebb's learning traces its origin in Pavlov's classical conditioning; however, while the former has been extensively modeled in the past decades (e.g., by the Hopfield model and countless variations on theme), as for the latter, modeling has remained largely unaddressed so far. Furthermore, a mathematical bridge connecting these two pillars is totally lacking. The main difficulty toward this goal lies in the intrinsically different scales of the information involved: Pavlov's theory is about correlations between concepts that are (dynamically) stored in the synaptic matrix as exemplified by the celebrated experiment starring a dog and a ringing bell; conversely, Hebb's theory is about correlations between pairs of neurons as summarized by the famous statement that neurons that fire together wire together. In this letter, we rely on stochastic process theory to prove that as long as we keep neurons' and synapses' timescales largely split, Pavlov's mechanism spontaneously takes place and ultimately gives rise to synaptic weights that recover the Hebbian kernel.
更多查看译文
关键词
Backpropagation Learning
AI 理解论文
溯源树
样例
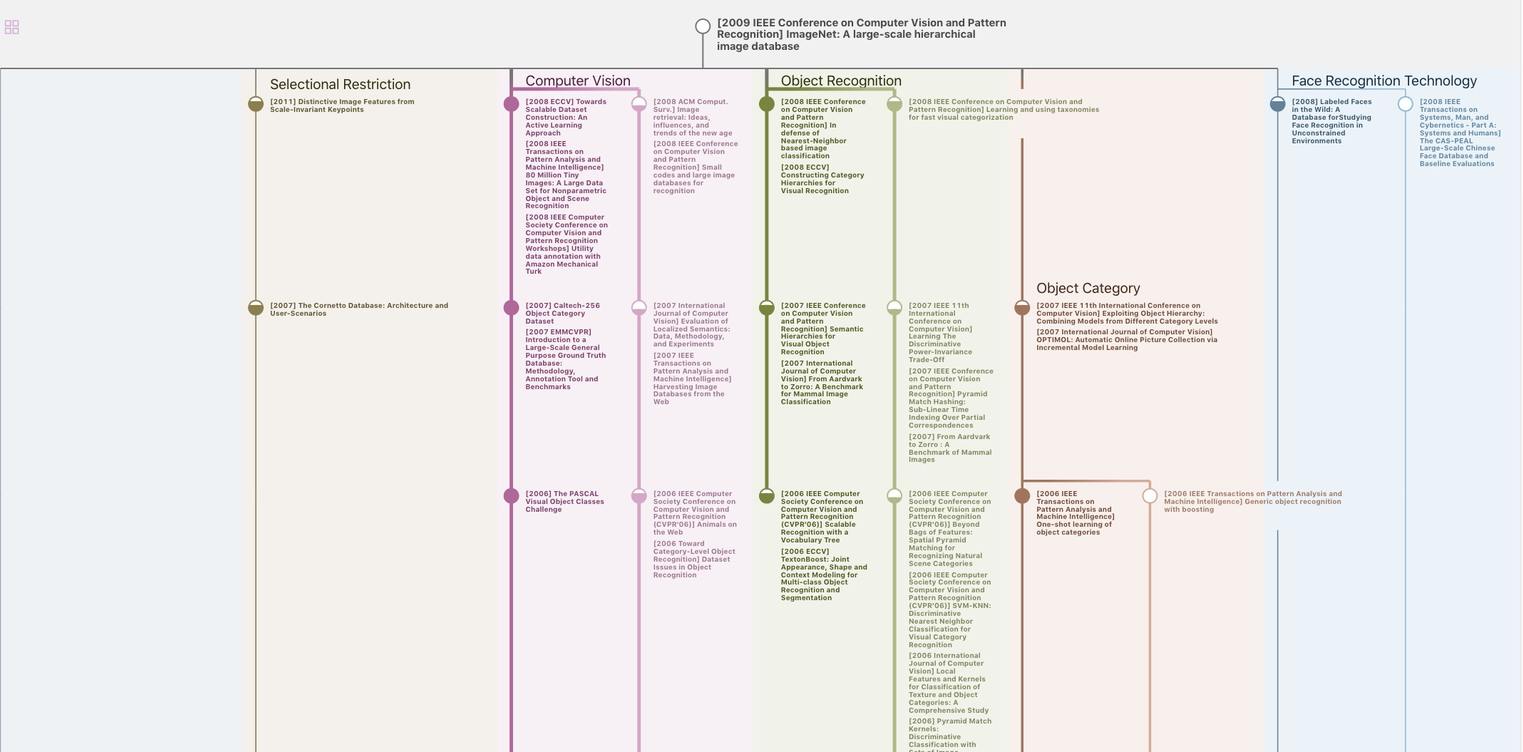
生成溯源树,研究论文发展脉络
Chat Paper
正在生成论文摘要