Author Correction: Synthesize high-dimensional longitudinal electronic health records via hierarchical autoregressive language model
Nature Communications(2023)
摘要
Synthetic electronic health records (EHRs) that are both realistic and privacy-preserving offer alternatives to real EHRs for machine learning (ML) and statistical analysis. However, generating high-fidelity EHR data in its original, high-dimensional form poses challenges for existing methods. We propose Hierarchical Autoregressive Language mOdel (HALO) for generating longitudinal, high-dimensional EHR, which preserve the statistical properties of real EHRs and can train accurate ML models without privacy concerns. HALO generates a probability density function over medical codes, clinical visits, and patient records, allowing for generating realistic EHR data without requiring variable selection or aggregation. Extensive experiments demonstrated that HALO can generate high-fidelity data with high-dimensional disease code probabilities closely mirroring (above 0.9 R2 correlation) real EHR data. HALO also enhances the accuracy of predictive modeling and enables downstream ML models to attain similar accuracy as models trained on genuine data. The paper presents HALO, a Hierarchical Autoregressive Language Model, for generating high-fidelity, longitudinal electronic health records (EHR) data. HALO maintains statistical property, supports machine learning modeling without privacy concerns.
更多查看译文
AI 理解论文
溯源树
样例
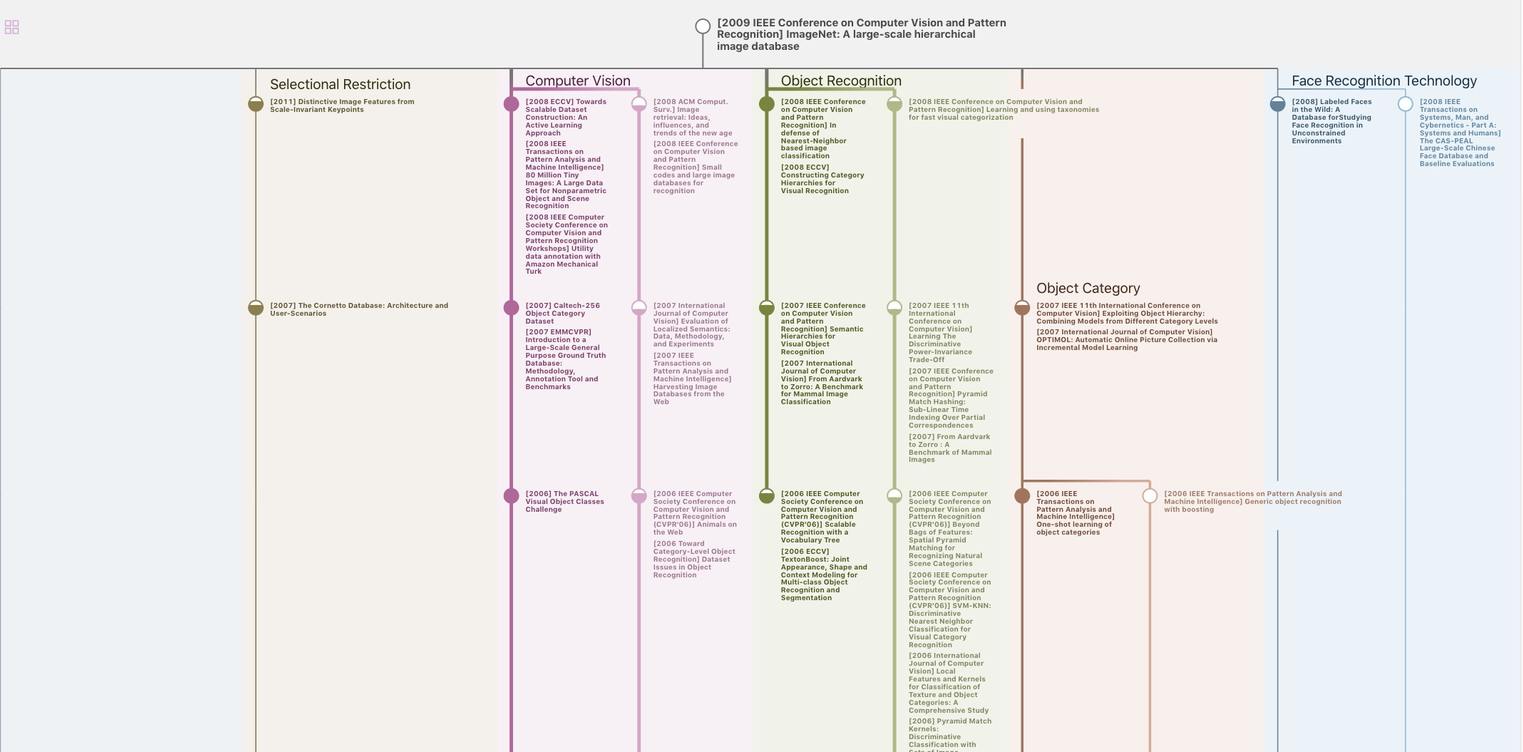
生成溯源树,研究论文发展脉络
Chat Paper
正在生成论文摘要