On-street parking for freight, services, and e-commerce traffic in US cities: A simulation model incorporating demand and duration
Transportation Research Part A: Policy and Practice(2023)
摘要
This paper develops a modeling framework to estimate the on-street parking demand for urban commercial traffic in the US using publicly available data. The commercial traffic includes the freight trip generation (pick-ups and deliveries), service trip attraction (service visits to establishments), and e-commerce deliveries, altogether referred to as freight and service activities (FSA) in this study. The methodology includes a simulation model incorporating FSA demand estimation, probability distributions for FSA duration, and industry sectors at the 2-digit North American Industry Classification System (NAICS) level. The authors validated the model through a case study of the SoHo commercial complex's parking demand in New York City (NYC) (Campbell et al., 2018). In addition, the study analyzes the magnitude of the parking issue in NYC comparing five ZIP codes in Midtown Manhattan, which generate the highest commercial demand in the city. The study extends the analysis to the busiest ZIP codes in six cities in the US (Austin, TX; Columbus, OH; San Jose, CA; Seattle, WA; Washington DC; Boston, MA). The results show that the parking spaces required during peak hours at the denser areas (i.e., ZIP codes in Manhattan) cannot be accommodated by the available on-street parking capacities. The authors tested four demand management initiatives to reduce the peak-hour needs. The off-hours strategy, which indicates 30 % of freight pick-ups and deliveries and 51 % of service visits shifting to the off-hours, is the most effective one, with the potential to reduce daytime parking needs by 30 %. Results also show the need to dedicate curb space for FSA vehicle parking to satisfy time-of-day requirements.
更多查看译文
关键词
Freight and service activity,On-street parking,Duration data,Freight trip generation,Service trip attraction
AI 理解论文
溯源树
样例
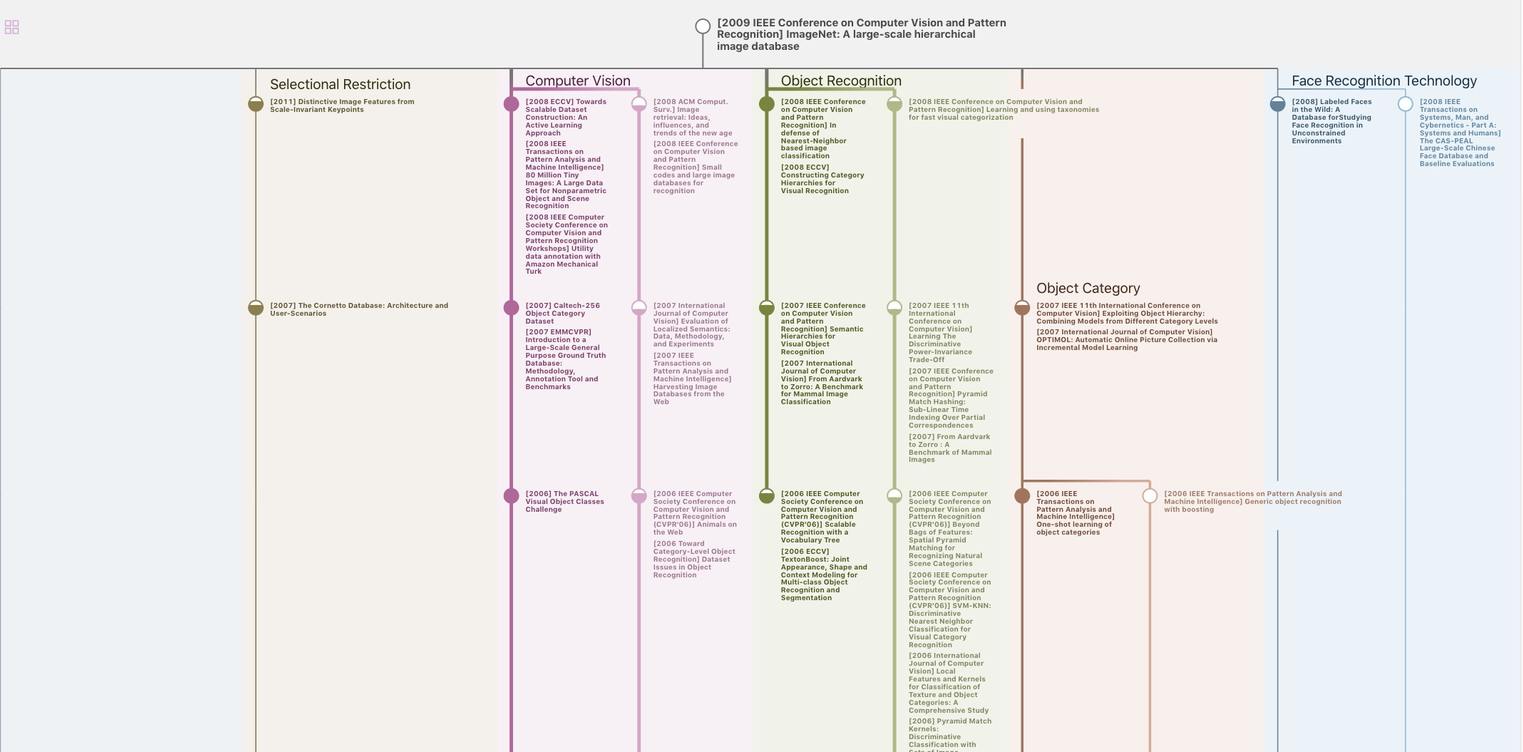
生成溯源树,研究论文发展脉络
Chat Paper
正在生成论文摘要