Nearly tight bounds on the price of explainability for the k-center and the maximum-spacing clustering problems
Theoretical Computer Science(2023)
摘要
The price of explainability for a clustering task can be defined as the unavoidable loss, in terms of the objective function, if we force the final partition to be explainable. Here, we study this price for the k-centers and maximum-spacing clustering problems. We provide nearly tight bounds for a natural model where explainability is achieved via decision trees.
更多查看译文
关键词
Clustering,Decision trees,Explainability
AI 理解论文
溯源树
样例
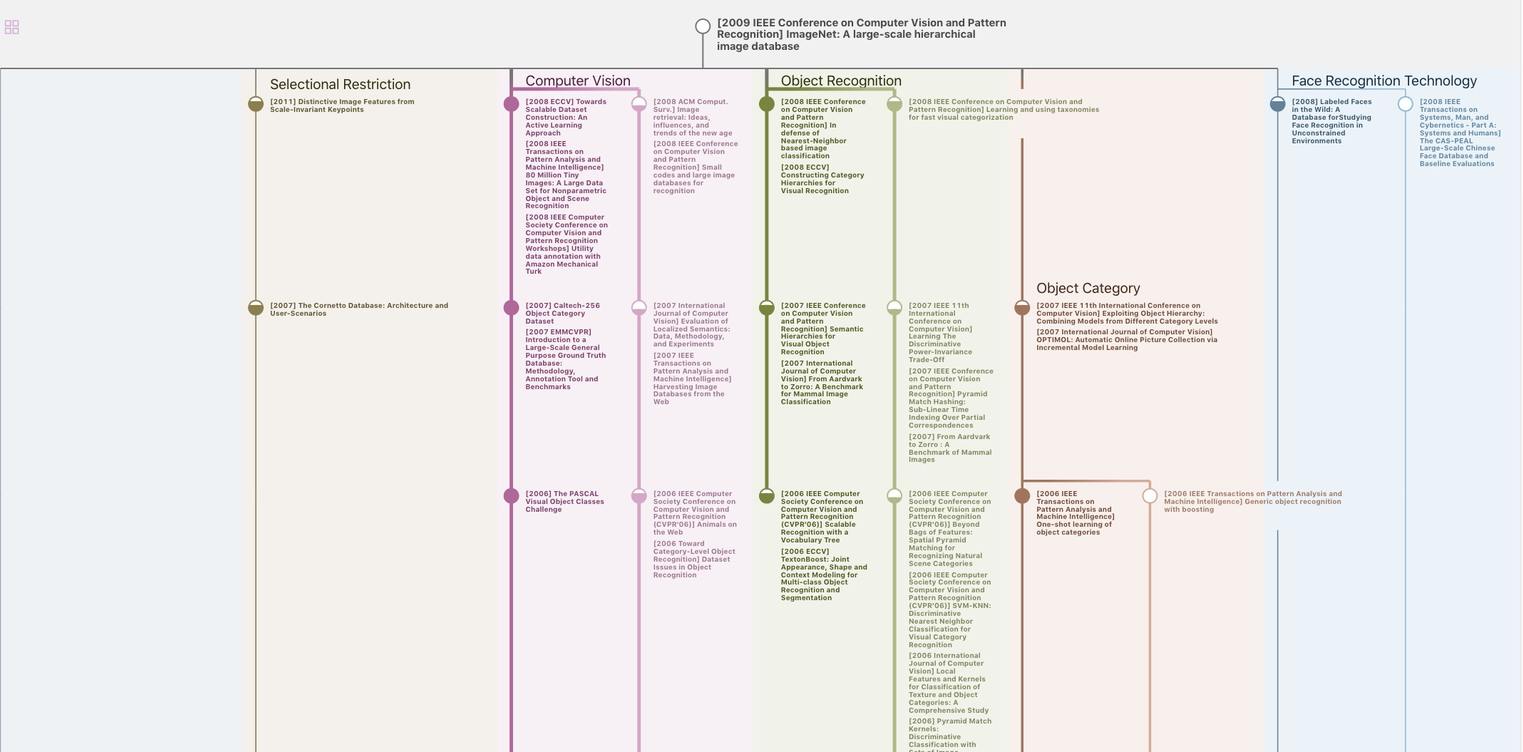
生成溯源树,研究论文发展脉络
Chat Paper
正在生成论文摘要