S3-CIMA: Supervised spatial single-cell image analysis for the identification of disease-associated cell type compositions in tissue
biorxiv(2023)
摘要
The spatial organization of various cell types within the tissue microenvironment is a key element for the formation of physiological and pathological processes, including cancer and autoimmune diseases. Here, we present S3-CIMA, a weakly supervised convolutional neural network model that enables the detection of disease-specific microenvironment compositions from high-dimensional proteomic imaging data. We demonstrate the utility of this approach by determining cancer outcome- and cellular signaling-specific spatial cell state compositions in highly multiplexed fluorescence microscopy data of the tumor microenvironment in colorectal cancer. Moreover, we use S3-CIMA to identify disease onset-specific changes of the pancreatic tissue microenvironment in type 1 diabetes using imaging mass cytometry data. We evaluated S3-CIMA as a powerful tool to discover novel disease-associated spatial cellular interactions from currently available and future spatial biology datasets.
### Competing Interest Statement
MC is a co-founder and holds stock of Scailyte AG. This work is independent of this status. CMS. is a scientific advisor to, has stock options in, and has received research funding from Enable Medicine, Inc., all outside of this work. The other authors declare no conflicts of interest.
更多查看译文
AI 理解论文
溯源树
样例
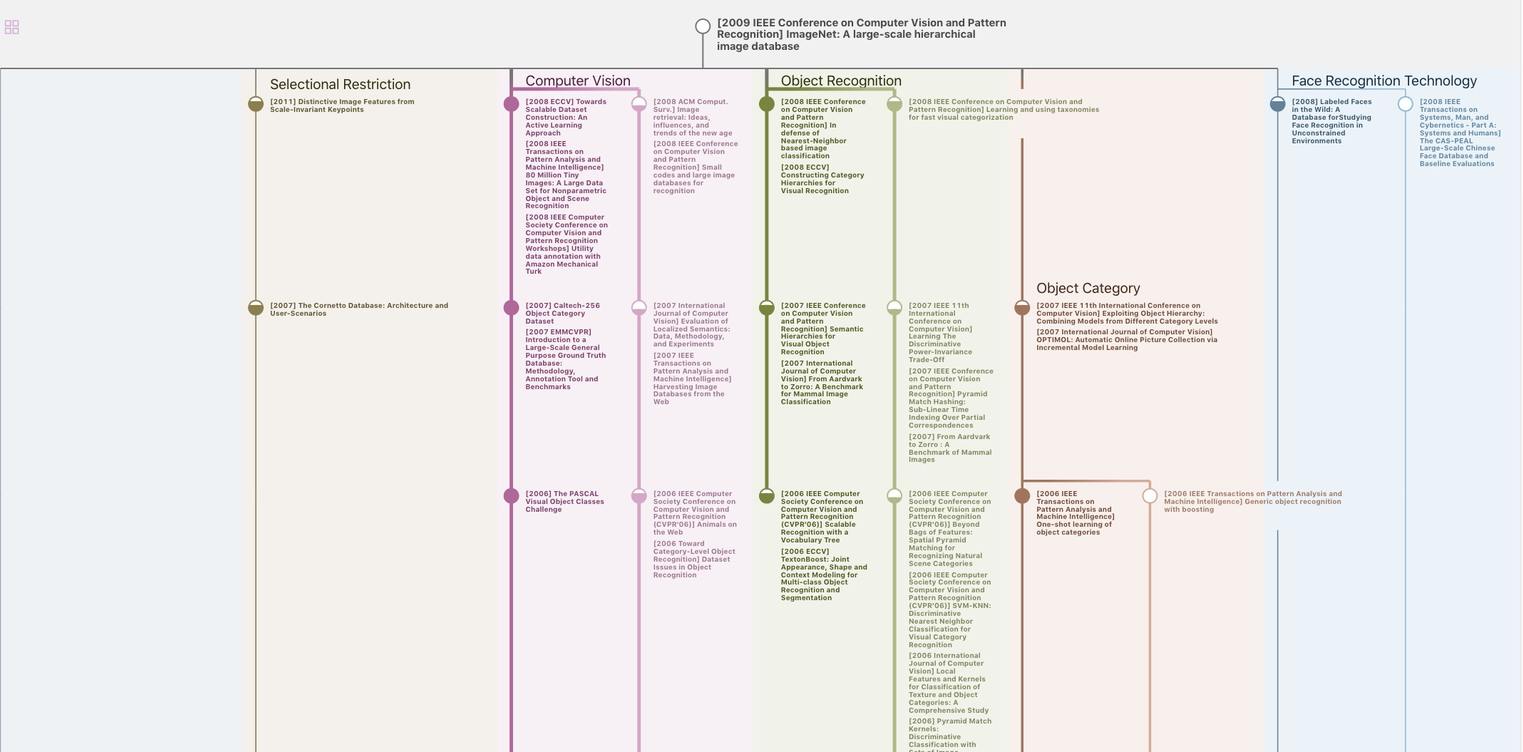
生成溯源树,研究论文发展脉络
Chat Paper
正在生成论文摘要