Salient Span Masking for Temporal Understanding
17TH CONFERENCE OF THE EUROPEAN CHAPTER OF THE ASSOCIATION FOR COMPUTATIONAL LINGUISTICS, EACL 2023(2023)
Abstract
Salient Span Masking (SSM) has shown itself to be an effective strategy to improve closedbook question answering performance. SSM extends general masked language model pretraining by creating additional unsupervised training sentences that mask a single entity or date span, thus oversampling factual information. Despite the success of this paradigm, the span types and sampling strategies are relatively arbitrary and not widely studied for other tasks. Thus, we investigate SSM from the perspective of temporal tasks, where learning a good representation of various temporal expressions is important. To that end, we introduce Temporal Span Masking (TSM) intermediate training. First, we find that SSM alone improves the downstream performance on three temporal tasks by an avg. +5.8 points. Further, we are able to achieve additional improvements (avg. +0.29 points) by adding the TSM task. These comprise the new best reported results on the targeted tasks. Our analysis suggests that the effectiveness of SSM stems from the sentences chosen in the training data rather than the mask choice: sentences with entities frequently also contain temporal expressions. Nonetheless, the additional targeted spans of TSM can still improve performance, especially in a zero-shot context.
MoreTranslated text
Key words
understanding
AI Read Science
Must-Reading Tree
Example
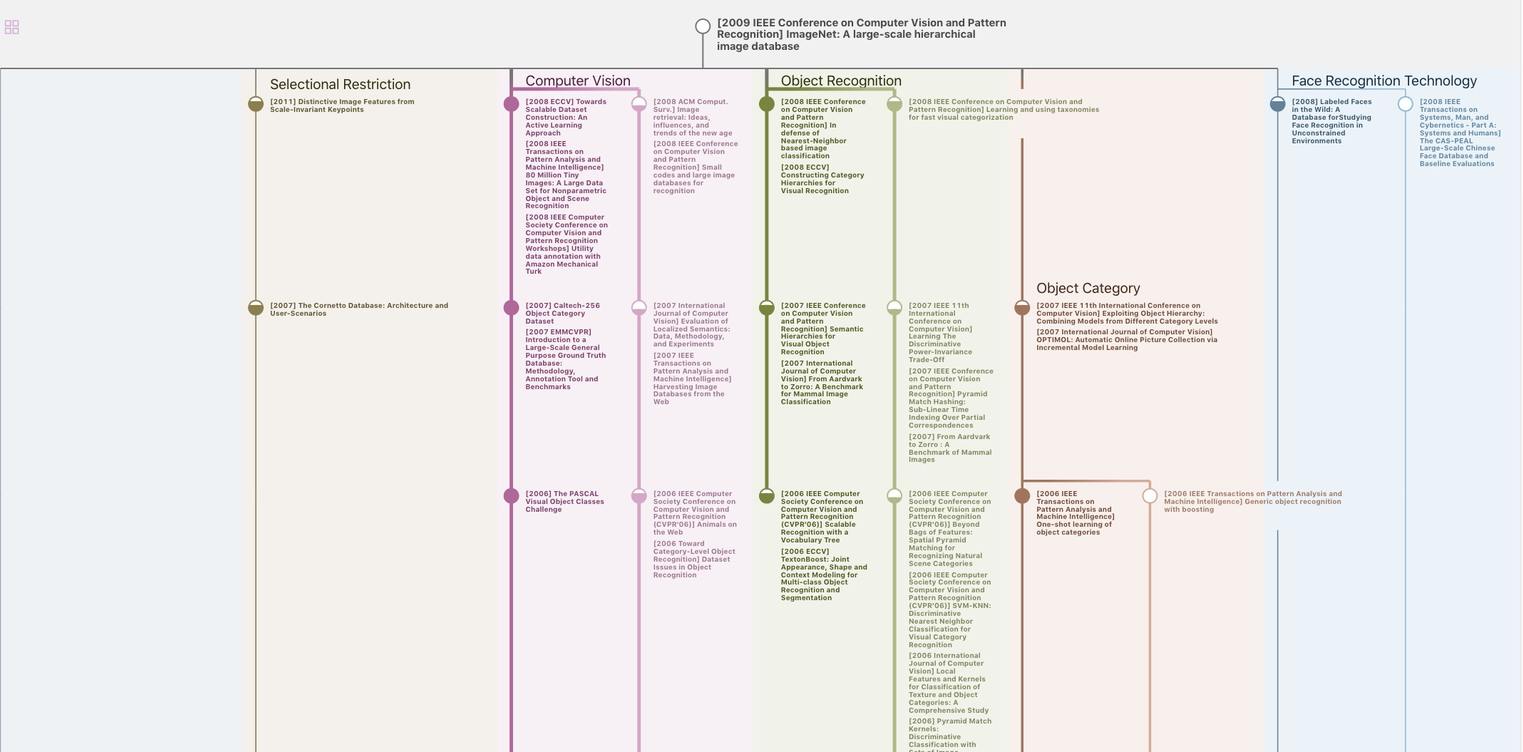
Generate MRT to find the research sequence of this paper
Chat Paper
Summary is being generated by the instructions you defined