Exploring Visual Prompts for Whole Slide Image Classification with Multiple Instance Learning
arxiv(2023)
摘要
Multiple instance learning (MIL) has emerged as a popular method for classifying histopathology whole slide images (WSIs). However, existing approaches typically rely on pre-trained models from large natural image datasets, such as ImageNet, to generate instance features, which can be sub-optimal due to the significant differences between natural images and histopathology images that lead to a domain shift. In this paper, we present a novel, simple yet effective method for learning domain-specific knowledge transformation from pre-trained models to histopathology images. Our approach entails using a prompt component to assist the pre-trained model in discerning differences between the pre-trained dataset and the target histopathology dataset, resulting in improved performance of MIL models. We validate our method on two publicly available datasets, Camelyon16 and TCGA-NSCLC. Extensive experimental results demonstrate the significant performance improvement of our method for different MIL models and backbones. Upon publication of this paper, we will release the source code for our method.
更多查看译文
关键词
whole slide image classification,visual prompts,learning
AI 理解论文
溯源树
样例
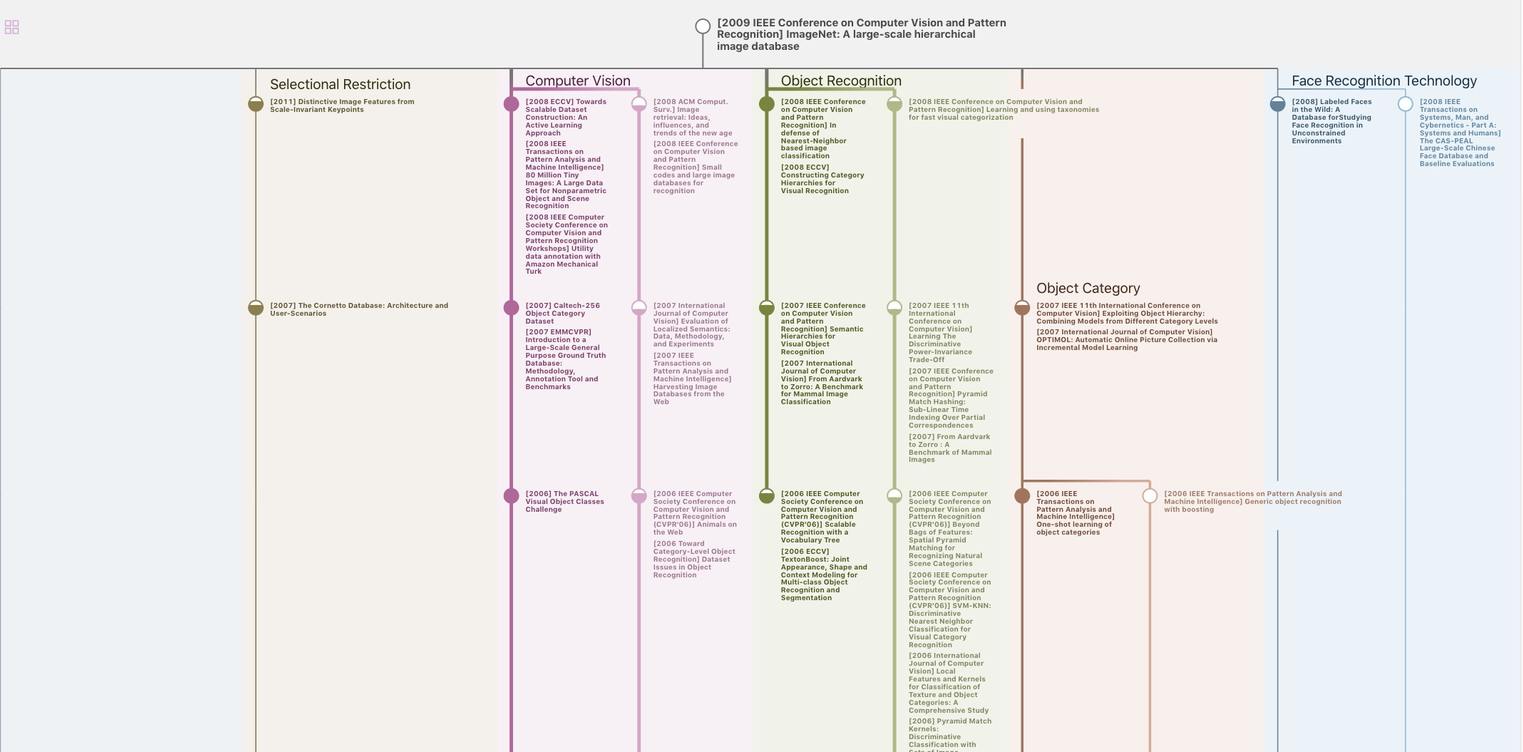
生成溯源树,研究论文发展脉络
Chat Paper
正在生成论文摘要