Machine learning COVID-19 detection from wearables
The Lancet: Digital Health(2023)
摘要
The increasing accessibility of wearable activity-tracking and health-tracking devices has prompted much research into passive diagnostics and screening that could contribute to infrastructure for population health testing and ultimately mitigate potential pandemics. Elevated resting heart rates have been noted to occur alongside fever.1Radin JM Wineinger NE Topol EJ Steinhubl SR Harnessing wearable device data to improve state-level real-time surveillance of influenza-like illness in the USA: a population-based study.Lancet Digit Health. 2020; 2: e85-e93Summary Full Text Full Text PDF PubMed Scopus (170) Google Scholar This finding has enabled researchers to accurately estimate the prevalence of influenza using data from wearable devices alone.1Radin JM Wineinger NE Topol EJ Steinhubl SR Harnessing wearable device data to improve state-level real-time surveillance of influenza-like illness in the USA: a population-based study.Lancet Digit Health. 2020; 2: e85-e93Summary Full Text Full Text PDF PubMed Scopus (170) Google Scholar In the past 3 years, studies show the potential to make individualised predictions of infection. For example, wearable devices have shown promise for population-level tracking of disease prevalence1Radin JM Wineinger NE Topol EJ Steinhubl SR Harnessing wearable device data to improve state-level real-time surveillance of influenza-like illness in the USA: a population-based study.Lancet Digit Health. 2020; 2: e85-e93Summary Full Text Full Text PDF PubMed Scopus (170) Google Scholar, 2Hirten RP Danieletto M Tomalin L et al.Use of physiological data from a wearable device to identify SARS-CoV-2 infection and symptoms and predict COVID-19 diagnosis: observational study.J Med Internet Res. 2021; 23e26107Crossref Scopus (66) Google Scholar and detection before the onset of symptoms.3Mason AE Hecht FM Davis SK et al.Detection of COVID-19 using multimodal data from a wearable device: results from the first TemPredict study.Sci Rep. 2022; 123463Google Scholar, 4Mishra T Wang M Metwally AA et al.Pre-symptomatic detection of COVID-19 from smartwatch data.Nat Biomed Eng. 2020; 4: 1208-1220Crossref PubMed Scopus (214) Google Scholar However, we caution the reader to pay close attention to the design of these studies and the outcomes they estimate. The methods of evaluation proposed in COVID-19 detection studies using machine learning do not replicate a realistic clinical use scenario. Until now, the performance of a one prediction per participant per day model to detect COVID-19 with wearables has not been described. The following criteria must be satisfied before a study can claim reasonable COVID-19 detection performance using data from wearable devices: (1) the latest training data must predate the earliest testing data when data are non-stationary, otherwise the performance is greatly inflated as a result of data leakage; (2) the evaluation period must not be artificially cropped around the event window, otherwise disease incidence is increased to unrealistic levels; (3) the evaluation period must not exclude participants that always test negative, as such exclusion results in a skewed representation of the population at large; and (4) the model must differentiate between COVID-19 and other conditions with similar characteristics—eg, non-COVID-19 influenza-like illness—when claiming to detect COVID-19. Here we show the necessity of these design requirements by drawing from literature since the start of the COVID-19 pandemic. Our goal is to contextualise the results reported in the literature to accurately assess and interpret the current state of the art in COVID-19 detection using wearables (table). These four requirements must be satisfied across many applications of artificial intelligence (AI) in health care. If we want ubiquitous adoption of AI, we must ensure that the results generalise from paper to practice.TableDesign decisions made in existing literature when detecting influenza-like illness, such as COVID-19, using wearablesNegative controlsUses prospective test setData not truncated to time-of-onsetDifferentiates between influenza-like illness and COVID-19Radin et al (2020)1Radin JM Wineinger NE Topol EJ Steinhubl SR Harnessing wearable device data to improve state-level real-time surveillance of influenza-like illness in the USA: a population-based study.Lancet Digit Health. 2020; 2: e85-e93Summary Full Text Full Text PDF PubMed Scopus (170) Google ScholarYesNoYesNoMishra et al (2020)4Mishra T Wang M Metwally AA et al.Pre-symptomatic detection of COVID-19 from smartwatch data.Nat Biomed Eng. 2020; 4: 1208-1220Crossref PubMed Scopus (214) Google ScholarYesNoNoNoQuer et al (2021)5Quer G Radin JM Gadaleta M et al.Wearable sensor data and self-reported symptoms for COVID-19 detection.Nat Med. 2021; 27: 73-77Crossref PubMed Scopus (222) Google ScholarYesNoNoYesMiller et al (2020)6Miller DJ Capodilupo JV Lastella M et al.Analyzing changes in respiratory rate to predict the risk of COVID-19 infection.PLoS One. 2020; 15e0243693Crossref Scopus (78) Google ScholarYesYesNoYesNatarajan et al (2020)7Natarajan A Su H-W Heneghan C Assessment of physiological signs associated with COVID-19 measured using wearable devices.NPJ Digit Med. 2020; 3: 156Crossref PubMed Scopus (117) Google ScholarYesNoNoNoMason et al (2022)3Mason AE Hecht FM Davis SK et al.Detection of COVID-19 using multimodal data from a wearable device: results from the first TemPredict study.Sci Rep. 2022; 123463Google ScholarNoNoNoNoHirten et al (2021)2Hirten RP Danieletto M Tomalin L et al.Use of physiological data from a wearable device to identify SARS-CoV-2 infection and symptoms and predict COVID-19 diagnosis: observational study.J Med Internet Res. 2021; 23e26107Crossref Scopus (66) Google ScholarYesYesNoNoShandhi et al (2022)8Shandhi MMH Cho PJ Roghanizad AR et al.A method for intelligent allocation of diagnostic testing by leveraging data from commercial wearable devices: a case study on COVID-19.NPJ Digit Med. 2022; 5: 130Crossref PubMed Scopus (8) Google ScholarNoNoNoYes Open table in a new tab In machine learning it is common to draw training, validation, and testing data in a retrospective study at random; in some cases, the data generation dates might even be obscured for privacy. However, if training and test sets co-occur, some COVID-19 prevalence captured in the training data will have occurred later, at the time of the data collection used in the test set—an issue known as data leakage. This implicit information on the weekly risk of COVID-19 is knowledge that the algorithm does not have access to when used in real time. When information about the current COVID-19 prevalence leaks to the model during testing, the performance is likely to be inflated as the method has seen the future as part of the training data. Data on infectious diseases and other non-stationary targets often violate the assumption of being independent and identically distributed after implementation; a property that is inherent to a randomly sampled test set. To test the hypothesis of inflated performance as a result of data leakage, we used previously published data9Shapiro A Marinsek N Clay I et al.Characterizing COVID-19 and influenza illnesses in the real world via person-generated health data.Patterns (NY). 2020; 2100188PubMed Google Scholar (termed FLUVEY) to train a model that uses 48 features from wearable devices to identify COVID-19 on a daily basis. Data were trained on a random draw of 35% of the participants. An additional 7·5% of the participants were allocated to the validation set, 7·5% to the retrospective test set, and 50% to a simulated deployment test set. The model was retrained weekly with all cumulative data collected for the training participants. The prospective test set encompassed data from a distinct group of participants starting the day after the last retrospective training date for a total of 7 days (tnow+1 to tnow+7). The randomly drawn test set that overlaps the training set in time (but not in participants) typically outperforms the prospective test set. The area under the receiver operating characteristic curve (AUROC) is 0·057 (SD 0·311) lower for the prospective test set than for a randomly drawn test set (appendix p 3). The performance of the model is clearly overestimated when using the retrospective test set, as the model implicitly learns the prevalence of the disease. This hypothesis is supported by the evidence that when the enumerated week-of-year is added as a feature, the AUROC for the retrospective test set outperforms the prospective deployment testing scenario by 0·302 (SD 0·218). This observation holds when the model is tasked with predicting any influenza-like illness, positive instances of which are more frequent in this cohort. Evaluating daily classification performance around a narrow window surrounding symptom onset distorts the perception of performance. False positive calls and true negative calls are vastly under-represented under these circumstances. A trend is shown in the sensitivity of reported findings (appendix p 4).3Mason AE Hecht FM Davis SK et al.Detection of COVID-19 using multimodal data from a wearable device: results from the first TemPredict study.Sci Rep. 2022; 123463Google Scholar, 4Mishra T Wang M Metwally AA et al.Pre-symptomatic detection of COVID-19 from smartwatch data.Nat Biomed Eng. 2020; 4: 1208-1220Crossref PubMed Scopus (214) Google Scholar, 5Quer G Radin JM Gadaleta M et al.Wearable sensor data and self-reported symptoms for COVID-19 detection.Nat Med. 2021; 27: 73-77Crossref PubMed Scopus (222) Google Scholar, 6Miller DJ Capodilupo JV Lastella M et al.Analyzing changes in respiratory rate to predict the risk of COVID-19 infection.PLoS One. 2020; 15e0243693Crossref Scopus (78) Google Scholar, 7Natarajan A Su H-W Heneghan C Assessment of physiological signs associated with COVID-19 measured using wearable devices.NPJ Digit Med. 2020; 3: 156Crossref PubMed Scopus (117) Google Scholar, 10Merrill MA Althoff T Self-supervised pretraining and transfer learning enable flu and COVID-19 predictions in small mobile sensing datasets.arXiv. 2022; (published online May 26.)https://doi.org/10.48550/arXiv.2205.13607Google Scholar Model performance tends to improve, irrespective of model capacity or size of dataset, when the class-dependent sampling techniques depart from the disease prevalence. Selectively eliminating hard-to-classify data is reflected by an artificial increase in COVID-19 cases in the test set. Some previous studies3Mason AE Hecht FM Davis SK et al.Detection of COVID-19 using multimodal data from a wearable device: results from the first TemPredict study.Sci Rep. 2022; 123463Google Scholar, 4Mishra T Wang M Metwally AA et al.Pre-symptomatic detection of COVID-19 from smartwatch data.Nat Biomed Eng. 2020; 4: 1208-1220Crossref PubMed Scopus (214) Google Scholar consider positive predictions within extensive windows before the date of the first symptom as true positives, leading to outlying sensitivity. By contrast, we trained an XGBoost model to directly predict COVID and to train an XGBoost influenza-like illness detector, then trained a gated recurrent unit survey model to differentiate between influenza-like illness and COVID-19 symptoms; we refer to this as the combined model. This combined model was re-evaluated—using identical weights but with thresholds redrawn according to the dataset—on an additional, externally collected dataset (denoted C19ex) that enrolled participants regardless of COVID-19 infection. All three of our evaluations have a test set class balance equal to the COVID-19 prevalence in our cohorts. We find that, in these cases of extreme class imbalance, the addition of survey differentiation to distinguish between cases of COVID-19 and cases of non-COVID-19 influenza-like illness is essential for a reasonable sensitivity. However, this increase in sensitivity comes at the expense of not detecting COVID-19 before symptom onset. Previous studies tended to downsample not at random,2Hirten RP Danieletto M Tomalin L et al.Use of physiological data from a wearable device to identify SARS-CoV-2 infection and symptoms and predict COVID-19 diagnosis: observational study.J Med Internet Res. 2021; 23e26107Crossref Scopus (66) Google Scholar, 3Mason AE Hecht FM Davis SK et al.Detection of COVID-19 using multimodal data from a wearable device: results from the first TemPredict study.Sci Rep. 2022; 123463Google Scholar, 4Mishra T Wang M Metwally AA et al.Pre-symptomatic detection of COVID-19 from smartwatch data.Nat Biomed Eng. 2020; 4: 1208-1220Crossref PubMed Scopus (214) Google Scholar, 6Miller DJ Capodilupo JV Lastella M et al.Analyzing changes in respiratory rate to predict the risk of COVID-19 infection.PLoS One. 2020; 15e0243693Crossref Scopus (78) Google Scholar, 7Natarajan A Su H-W Heneghan C Assessment of physiological signs associated with COVID-19 measured using wearable devices.NPJ Digit Med. 2020; 3: 156Crossref PubMed Scopus (117) Google Scholar, 9Shapiro A Marinsek N Clay I et al.Characterizing COVID-19 and influenza illnesses in the real world via person-generated health data.Patterns (NY). 2020; 2100188PubMed Google Scholar either by including only COVID-19-positive individuals or by predicting only on peak symptom days, thereby increasing the ease of the detection task. This increased ease is attested by the increase in AUROC, a prevalence-independent metric, as prevalence increases: simply evaluating our wearable model only on COVID-19-positive participants, when it was trained to detect COVID-19 among all participants with COVID-19 and influenza-like illness, improves the AUROC from 0·51 (SD 0·05) to 0·57 (SD 0·06) (figure). Similarly, distinguishing between COVID-19-positive and COVID-19-negative individuals only on days 0–3 of symptoms increases the AUROC from 0·51 (SD 0·05) to 0·65 (SD 0·11). Readers should be aware of how positive labels are defined: do they include asymptomatic and presymptomatic individuals and those with non-COVID-19 influenza-like illness, or do they include only individuals with symptomatic COVID-19? The additional effect of false positives and true negatives in the cohort will influence how scores are perceived, especially the positive predictive value (precision). Other illnesses are simultaneously present in these datasets, and not all symptoms are related to COVID-19. Surveys have been successful in differentiating non-COVID-19 influenza-like illness from COVID-19;12Callahan A Steinberg E Fries JA et al.Estimating the efficacy of symptom-based screening for COVID-19.NPJ Digit Med. 2020; 3: 95Crossref PubMed Scopus (25) Google Scholar, 13Menni C Valdes AM Freidin MB et al.Real-time tracking of self-reported symptoms to predict potential COVID-19.Nat Med. 2020; 26: 1037-1040Crossref PubMed Scopus (857) Google Scholar however, they require the presence of symptoms that can occur over multiple days, leading to uncontained transmission.14He X Lau EHY Wu P et al.Temporal dynamics in viral shedding and transmissibility of COVID-19.Nat Med. 2020; 26: 672-675Crossref PubMed Scopus (2719) Google Scholar Continuous monitoring could enable earlier detection, but might not be able to differentiate between different illnesses. Whereas individuals use PCR testing and rapid antigen testing to establish the source of their symptoms, wearables would be better suited to predict whether symptoms will arise irrespective of illness. Previous work has shown that a model trained to detect the onset of influenza using wearables can transfer to COVID-19 detection without fine-tuning, achieving an AUROC of 0·68.10Merrill MA Althoff T Self-supervised pretraining and transfer learning enable flu and COVID-19 predictions in small mobile sensing datasets.arXiv. 2022; (published online May 26.)https://doi.org/10.48550/arXiv.2205.13607Google Scholar This finding implies that the manifestations of influenza and COVID-19 overlap in terms of the distribution of data from wearable devices. Models that claim to predict COVID-19 symptoms might be better classed as models that can predict respiratory virus illness. Future model designs should be tested on cohorts that do not artificially inflate the prevalence of COVID-19-positive days in the dataset. Models should be tested on prospective data, as a shifting target is inevitable with infectious diseases. Researchers should avoid making the claim of COVID-19 classification without explicitly being able to differentiate between cases of influenza-like illness and COVID-19. We encourage researchers to communicate their intended context of use—ie, what decision will the model facilitate—and evaluate their algorithms under that presumption. Doing so will inform study design choices and set realistic expectations for model performance during use. LF is a co-founder of Evidation Health, a company that powers research studies with person-generated health data. This work was funded by the National Institutes of Health, the National Cancer Institute, and the National Institute of Biomedical Imaging and Bioengineering award N. 75N91020C00034. AG is also funded by the Varma Family Chair and CIFAR AI Chair. Download .pdf (.36 MB) Help with pdf files Supplementary appendix
更多查看译文
AI 理解论文
溯源树
样例
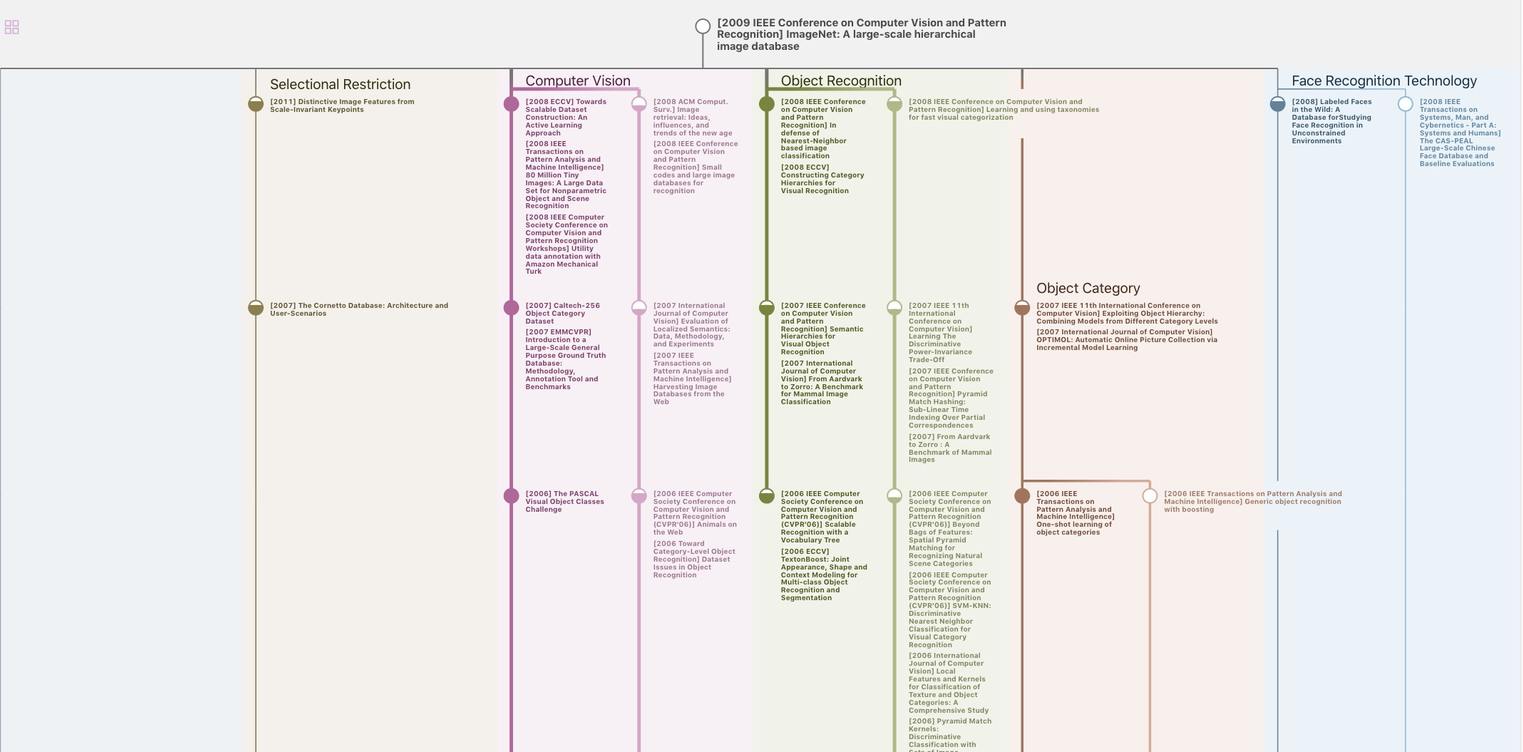
生成溯源树,研究论文发展脉络
Chat Paper
正在生成论文摘要