Autonomous scanning probe microscopy with hypothesis learning: Exploring the physics of domain switching in ferroelectric materials
Patterns(2023)
摘要
Using hypothesis-learning-driven automated scanning probe microscopy (SPM), we explore the bias-induced transformations that underpin the functionality of broad classes of devices and materials from batteries and memristors to ferroelectrics and antiferroelectrics. Optimization and design of these materials require probing the mechanisms of these transformations on the nanometer scale as a function of a broad range of control parameters, leading to experimentally intractable scenarios. Meanwhile, often these behaviors are understood within potentially competing theoretical hypotheses. Here, we develop a hypothesis list covering possible limiting scenarios for domain growth in ferroelectric materials, including thermodynamic, domain-wall pinning, and screening limited. The hypothesis-driven SPM autonomously identifies the mechanisms of bias-induced domain switching, and the results indicate that domain growth is ruled by kinetic control. We note that the hypothesis learning can be broadly used in other automated experiment settings.
更多查看译文
关键词
hypothesis learning,automated experiment,scanning probe microscopy,ferroelectrics
AI 理解论文
溯源树
样例
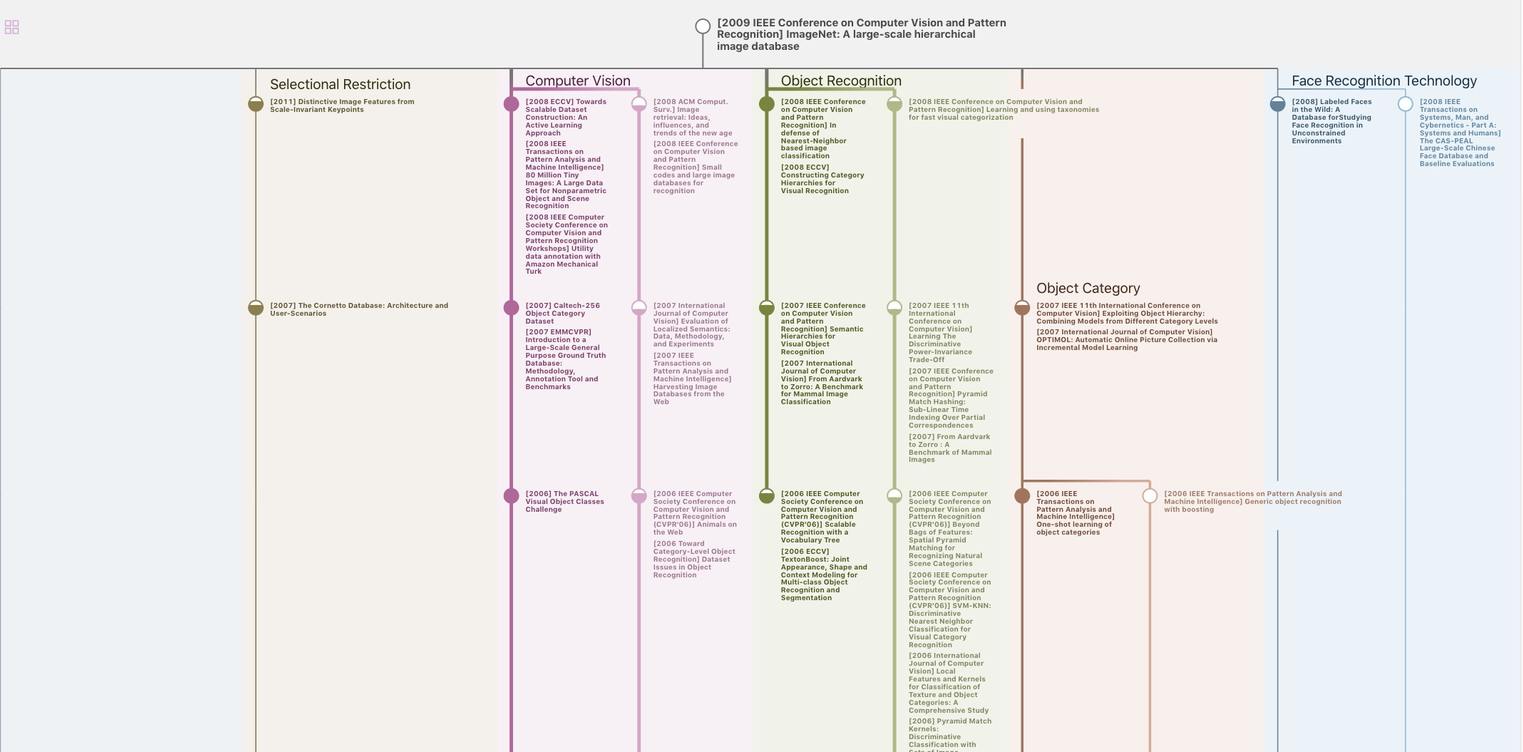
生成溯源树,研究论文发展脉络
Chat Paper
正在生成论文摘要