Low-frequency Image Deep Steganography: Manipulate the Frequency Distribution to Hide Secrets with Tenacious Robustness
CoRR(2023)
摘要
Image deep steganography (IDS) is a technique that utilizes deep learning to embed a secret image invisibly into a cover image to generate a container image. However, the container images generated by convolutional neural networks (CNNs) are vulnerable to attacks that distort their high-frequency components. To address this problem, we propose a novel method called Low-frequency Image Deep Steganography (LIDS) that allows frequency distribution manipulation in the embedding process. LIDS extracts a feature map from the secret image and adds it to the cover image to yield the container image. The container image is not directly output by the CNNs, and thus, it does not contain high-frequency artifacts. The extracted feature map is regulated by a frequency loss to ensure that its frequency distribution mainly concentrates on the low-frequency domain. To further enhance robustness, an attack layer is inserted to damage the container image. The retrieval network then retrieves a recovered secret image from a damaged container image. Our experiments demonstrate that LIDS outperforms state-of-the-art methods in terms of robustness, while maintaining high fidelity and specificity. By avoiding high-frequency artifacts and manipulating the frequency distribution of the embedded feature map, LIDS achieves improved robustness against attacks that distort the high-frequency components of container images.
更多查看译文
关键词
secrets,low-frequency
AI 理解论文
溯源树
样例
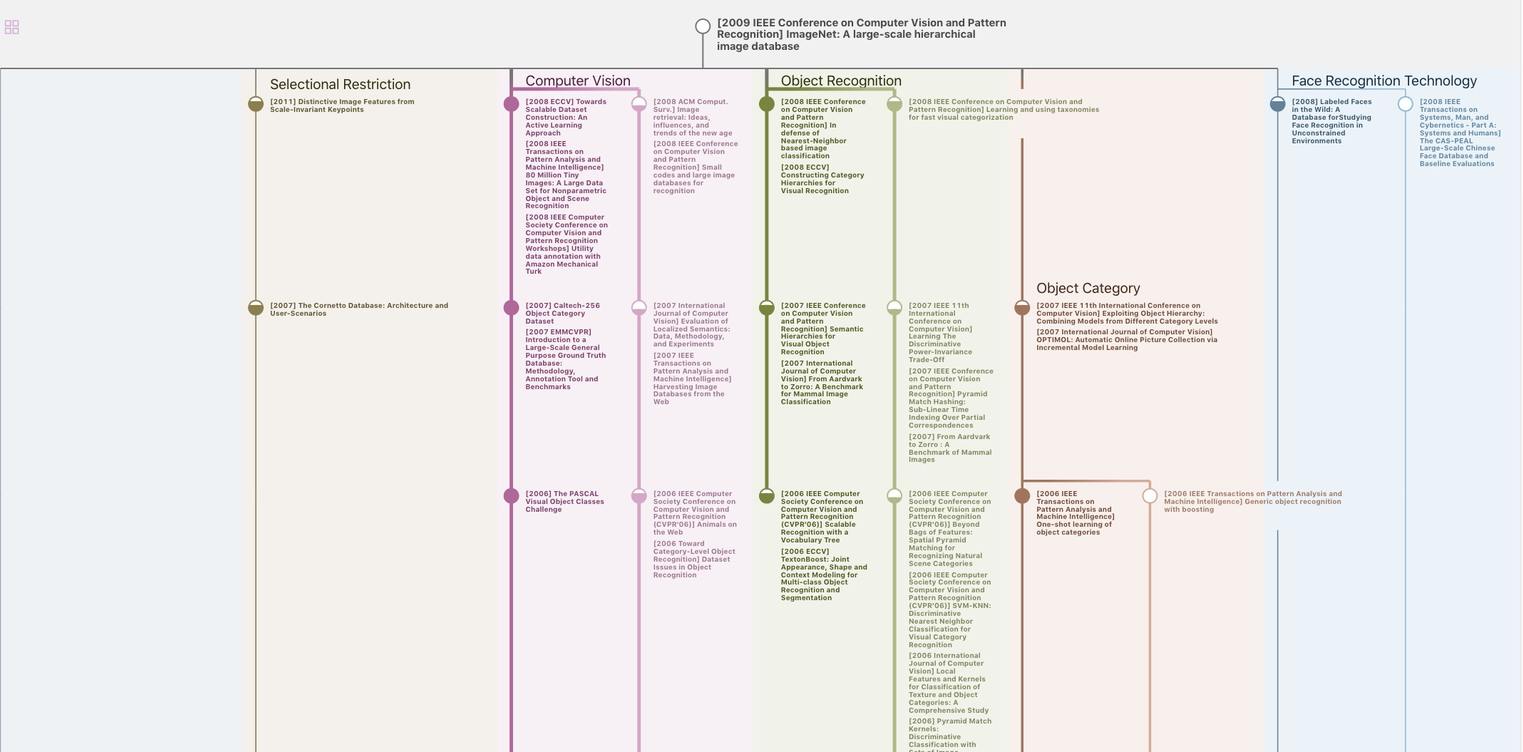
生成溯源树,研究论文发展脉络
Chat Paper
正在生成论文摘要