Edge-free but Structure-aware: Prototype-Guided Knowledge Distillation from GNNs to MLPs
CoRR(2023)
摘要
Distilling high-accuracy Graph Neural Networks~(GNNs) to low-latency multilayer perceptrons~(MLPs) on graph tasks has become a hot research topic. However, MLPs rely exclusively on the node features and fail to capture the graph structural information. Previous methods address this issue by processing graph edges into extra inputs for MLPs, but such graph structures may be unavailable for various scenarios. To this end, we propose a Prototype-Guided Knowledge Distillation~(PGKD) method, which does not require graph edges~(edge-free) yet learns structure-aware MLPs. Specifically, we analyze the graph structural information in GNN teachers, and distill such information from GNNs to MLPs via prototypes in an edge-free setting. Experimental results on popular graph benchmarks demonstrate the effectiveness and robustness of the proposed PGKD.
更多查看译文
关键词
knowledge distillation
AI 理解论文
溯源树
样例
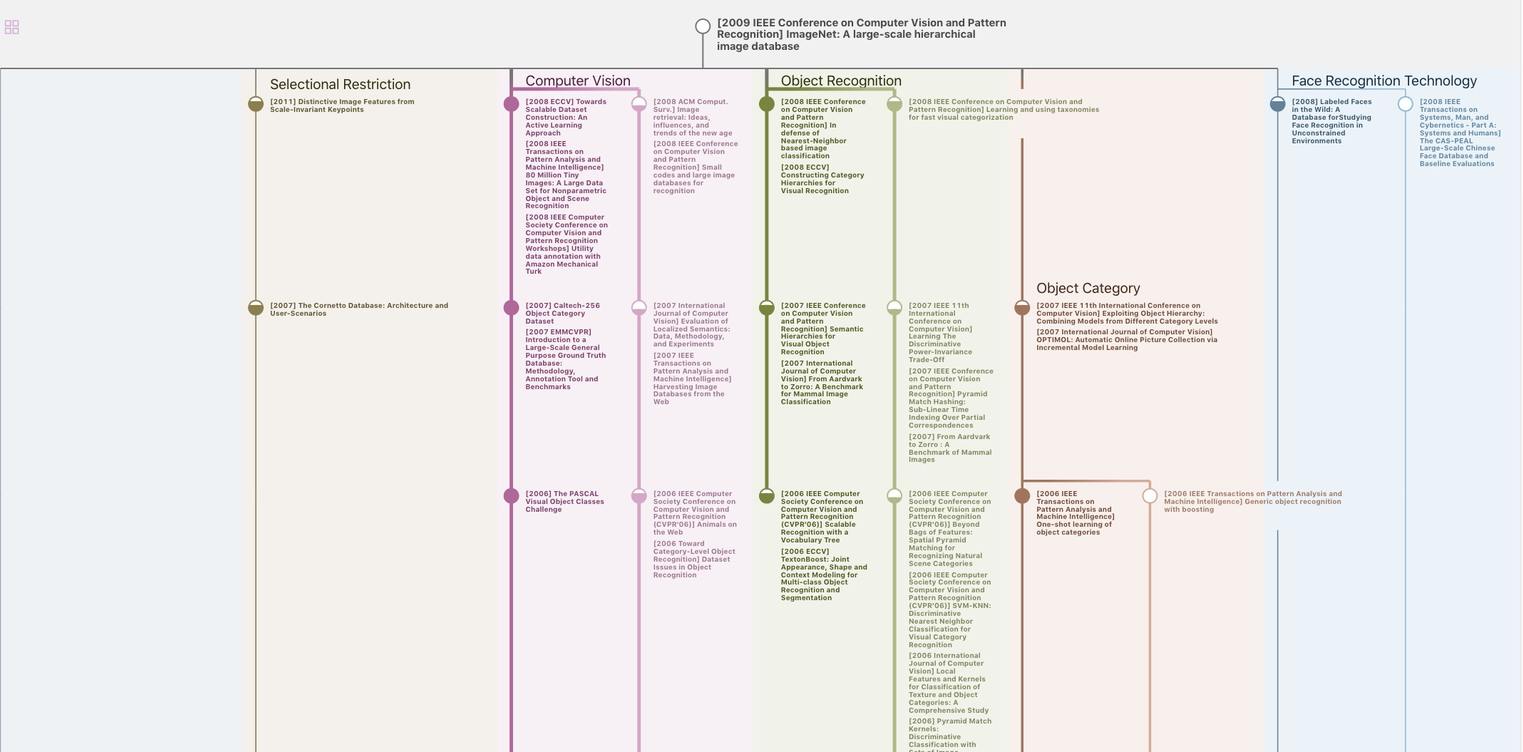
生成溯源树,研究论文发展脉络
Chat Paper
正在生成论文摘要