A deep learning approach for rapid detection of soil liquefaction using time–frequency images
Soil Dynamics and Earthquake Engineering(2023)
摘要
Soil liquefaction – a common hazard during earthquakes – can cause severe damage to infrastructure systems. The excessive pore water pressure induced by underground shaking can result in a sudden loss of soil stiffness and strength, which renders the soil–water mixture to behave temporarily as “liquid”. Identifying liquefaction events and extracting their time of initiation and duration from recorded data are critical capabilities for validating and improving predictive models, and ultimately, effectively mitigating this hazard. The current approach is to conduct on-site investigations or simplified statistical analyses, which are generally time-consuming and bear high uncertainties, except for the most obvious cases. In this study, we utilize a convolutional neural network (CNN), a deep learning method, to predict both the occurrence of a liquefaction event and its triggering time (if it occurred). For this purpose, first, more than one million high-fidelity nonlinear site response simulations with different soil profiles and ground motions are performed. The surface acceleration time-histories are then converted into time–frequency distribution images, which are fed into a CNN as the training inputs. The trained CNN exhibits high prediction accuracy for both training and validation sets, another suite of independent site response simulations, as well as real-life earthquake data.
更多查看译文
关键词
Liquefaction,Triggering,Deep learning,Convolutional neural network,Time–frequency distribution
AI 理解论文
溯源树
样例
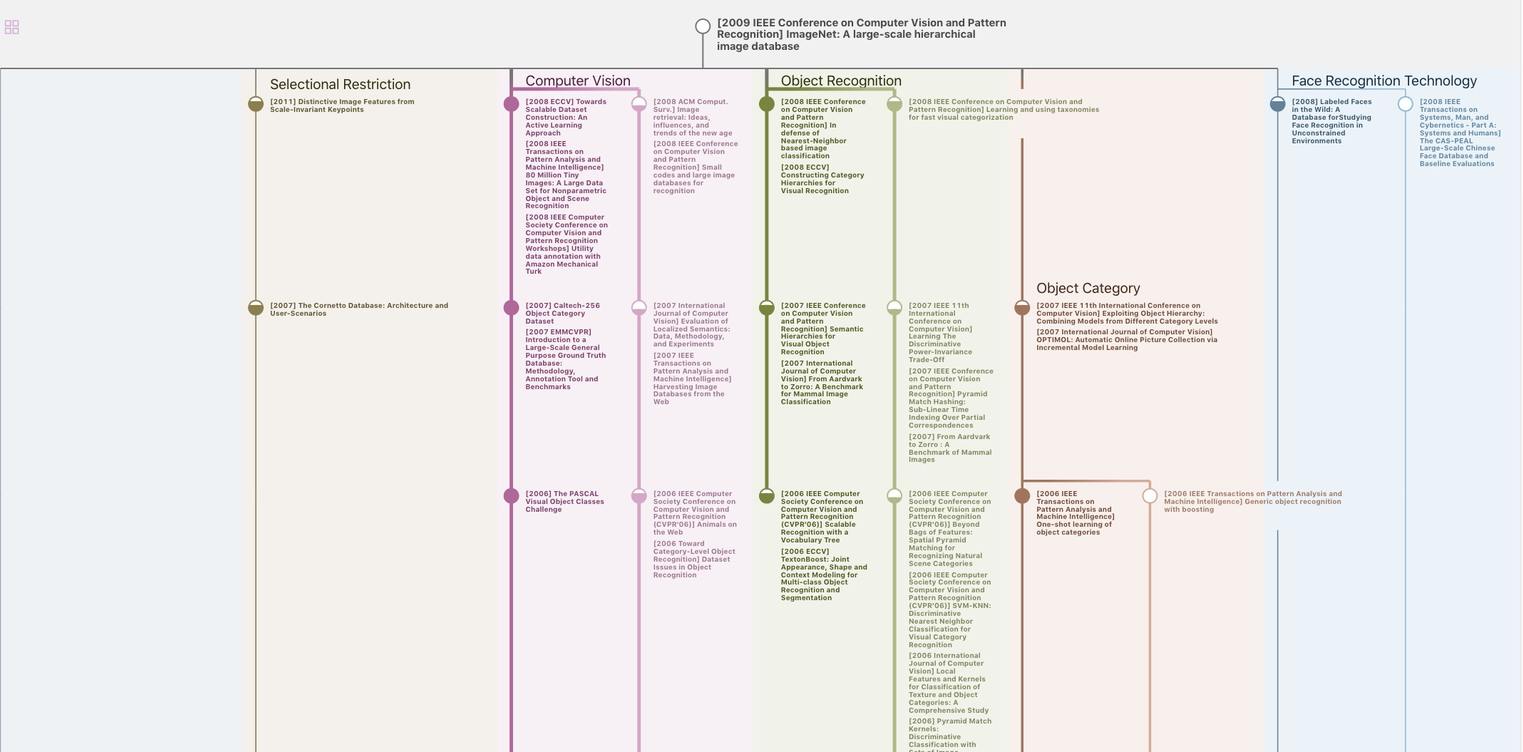
生成溯源树,研究论文发展脉络
Chat Paper
正在生成论文摘要