Bayesian Multi-head Convolutional Neural Networks with Bahdanau Attention for Forecasting Daily Precipitation in Climate Change Monitoring
ECML/PKDD (5)(2023)
摘要
General Circulation Models (GCMs) are established numerical models for simulating multiple climate variables, decades into the future. GCMs produce such simulations at coarse resolution (100 to 600 km), making them inappropriate to monitor climate change at the local regional level. Downscaling approaches are usually adopted to infer the statistical relationship between the coarse simulations of GCMs and local observations and use the relationship to evaluate the simulations at a finer scale. In this paper, we propose a novel deep learning framework for forecasting daily precipitation values via downscaling. Our framework, named Precipitation CNN or PCNN, employs multi-head convolutional neural networks (CNNs) followed by Bahdanau attention blocks and an uncertainty quantification component with Bayesian inference. We apply PCNN to downscale the daily precipitation above the New Jersey portion of the Hackensack-Passaic watershed. Experiments show that PCNN is suitable for this task, reproducing the daily variability of precipitation. Moreover, we produce local-scale precipitation projections for multiple periods into the future (up to year 2100).
更多查看译文
关键词
forecasting daily precipitation,bahdanau attention,climate change,multi-head
AI 理解论文
溯源树
样例
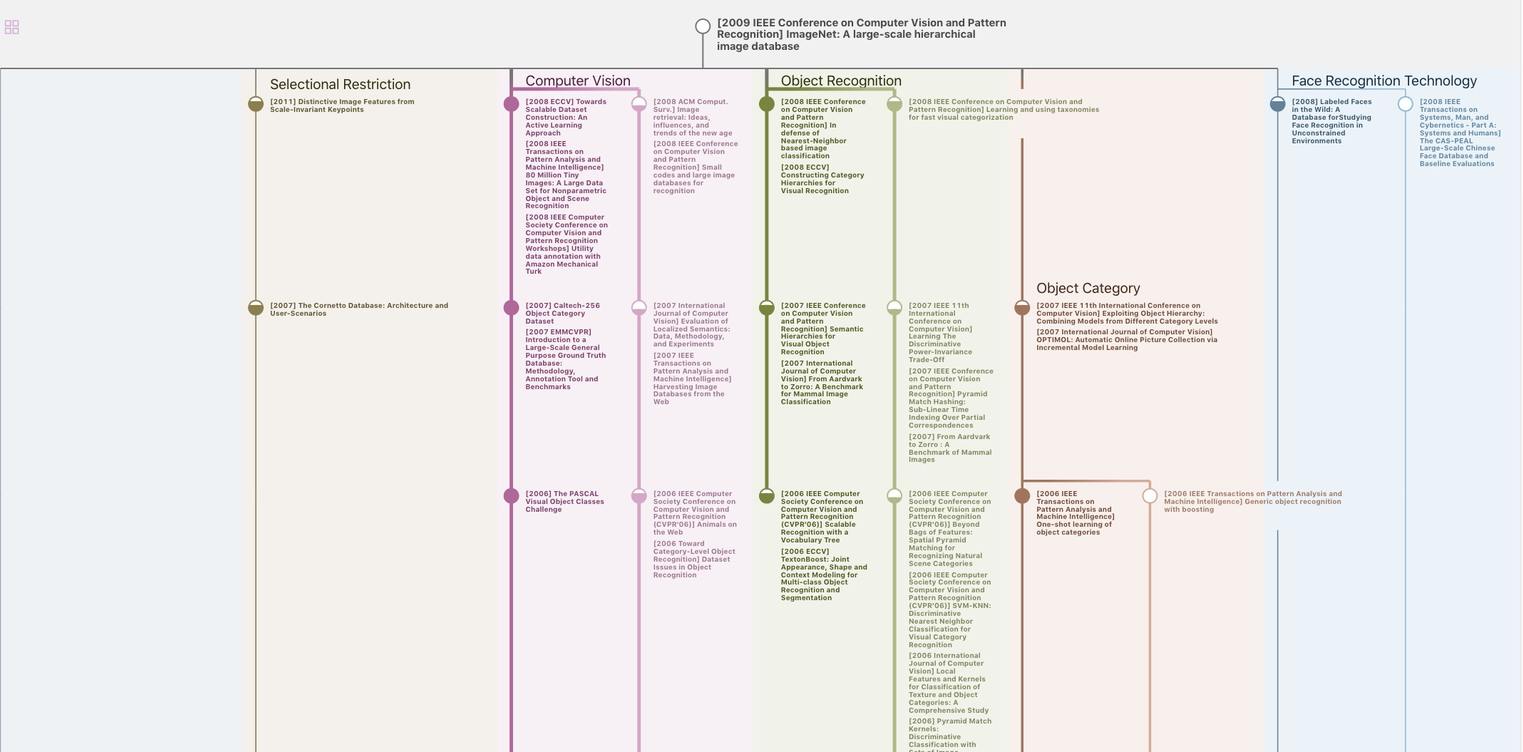
生成溯源树,研究论文发展脉络
Chat Paper
正在生成论文摘要