Vehicle Routing Problem with Value Iteration Network
Advanced Network Technologies and Intelligent Computing(2023)
摘要
Multi Vehicle routing to service consumers in dynamic and unpredictable surrounding such as congested urban areas is a difficult operation that needs robust and flexible planning. Value iteration networks hold promise for planning in vehicle routing problem. Conventional approaches aren’t usually constructed for real-life settings, and they are too slow to be useful in real-time. In comparison, Vehicle Routing Problem with Value Iteration Network (VRP-VIN) offers a neural network model based on graphs that can execute multi-agent routing in a highly dispersed but connected graph with constantly fluctuating traffic conditions using learned value iteration. Furthermore, the model’s communication module allows vehicles to work better in a cooperative manner online and can easily adapt to changes. A virtual environment is constructed to simulate real-world mapping by self-driving vehicles with uncertain traffic circumstances and minimal edge coverage. This method beats standard solutions based on overall cost and run-time. Experiments show that the model achieves a total cost difference of 3% when compared with a state-of-art solver having global information. Also, after being trained with only 2 agents on networks with 25 nodes, can easily generalize to a scenario having additional agents (or nodes).
更多查看译文
关键词
Reinforcement learning,Vehicle Routing Problem,Value iteration networks,Graph attention layer,Multi-agent communication
AI 理解论文
溯源树
样例
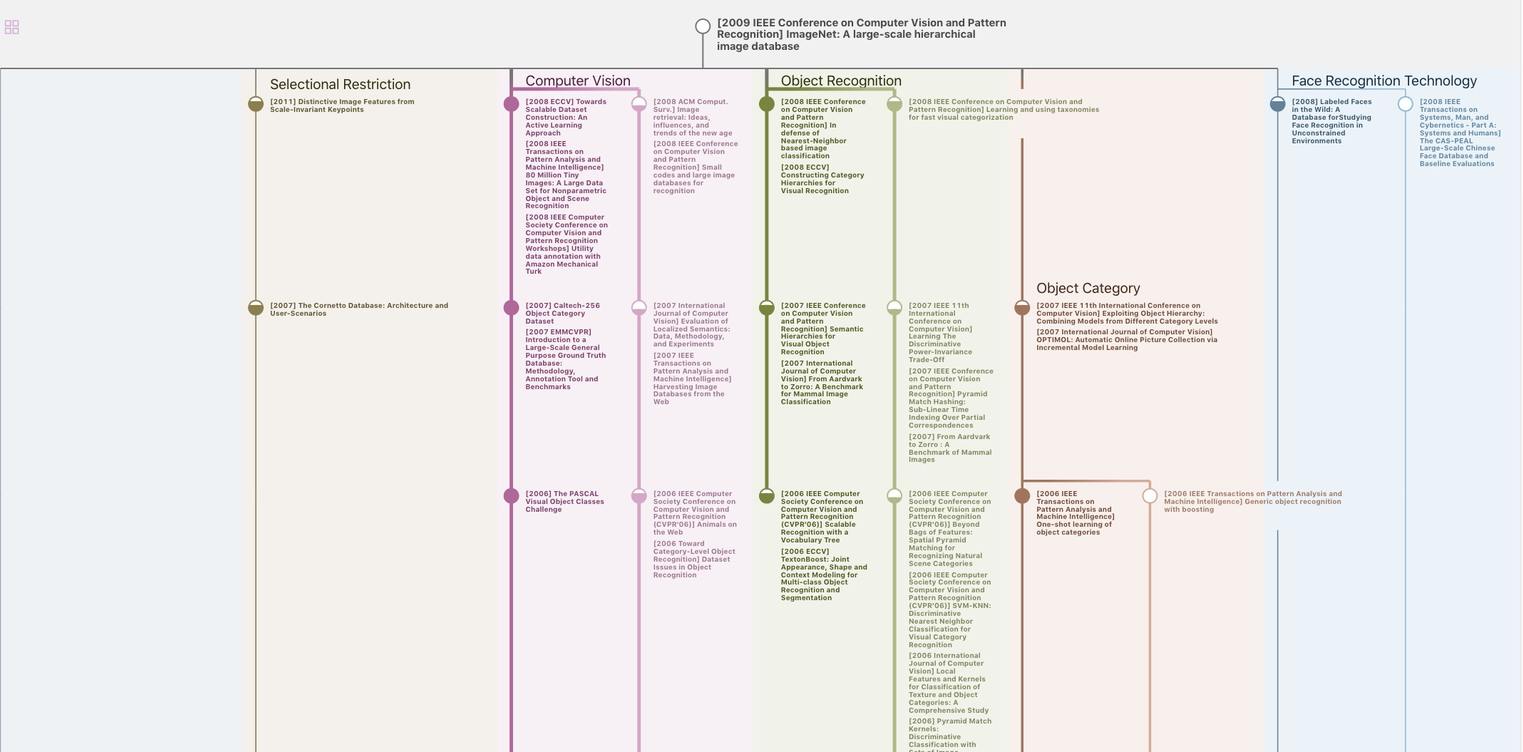
生成溯源树,研究论文发展脉络
Chat Paper
正在生成论文摘要