Robotic Control in Adversarial and Sparse Reward Environments: A Robust Goal-Conditioned Reinforcement Learning Approach.
IEEE transactions on artificial intelligence(2024)
摘要
With deep neural networks-based function approximators, reinforcement learning holds the promise of learning complex end-to-end robotic controllers that can map high-dimensional sensory information directly to control policies. However, a common challenge, especially for robotics, is sample-efficient learning from sparse rewards, in which an agent is required to find a long sequence of “correct” actions to achieve a desired outcome. Unfortunately, inevitable perturbations on observations may make this task trickier to solve. Here, this article advances a novel robust goal-conditioned reinforcement learning approach for end-to-end robotic control in adversarial and sparse reward environments. Specifically, a mixed adversarial attack scheme is presented to generate diverse adversarial perturbations on observations by combining white-box and black-box attacks. Meanwhile, a hindsight experience replay technique considering observation perturbations is developed to turn a failed experience into a successful one and generate the policy trajectories perturbed by the mixed adversarial attacks. Additionally, a robust goal-conditioned actor–critic method is proposed to learn goal-conditioned policies and keep the variations of the perturbed policy trajectories within bounds. Finally, the proposed method is evaluated on three tasks with adversarial attacks and sparse reward settings. The results indicate that our scheme can ensure robotic control performance and policy robustness on the adversarial and sparse reward tasks.
更多查看译文
关键词
Adversarial machine learning,end-to-end control,reinforcement learning (RL),robotics,sparse reward
AI 理解论文
溯源树
样例
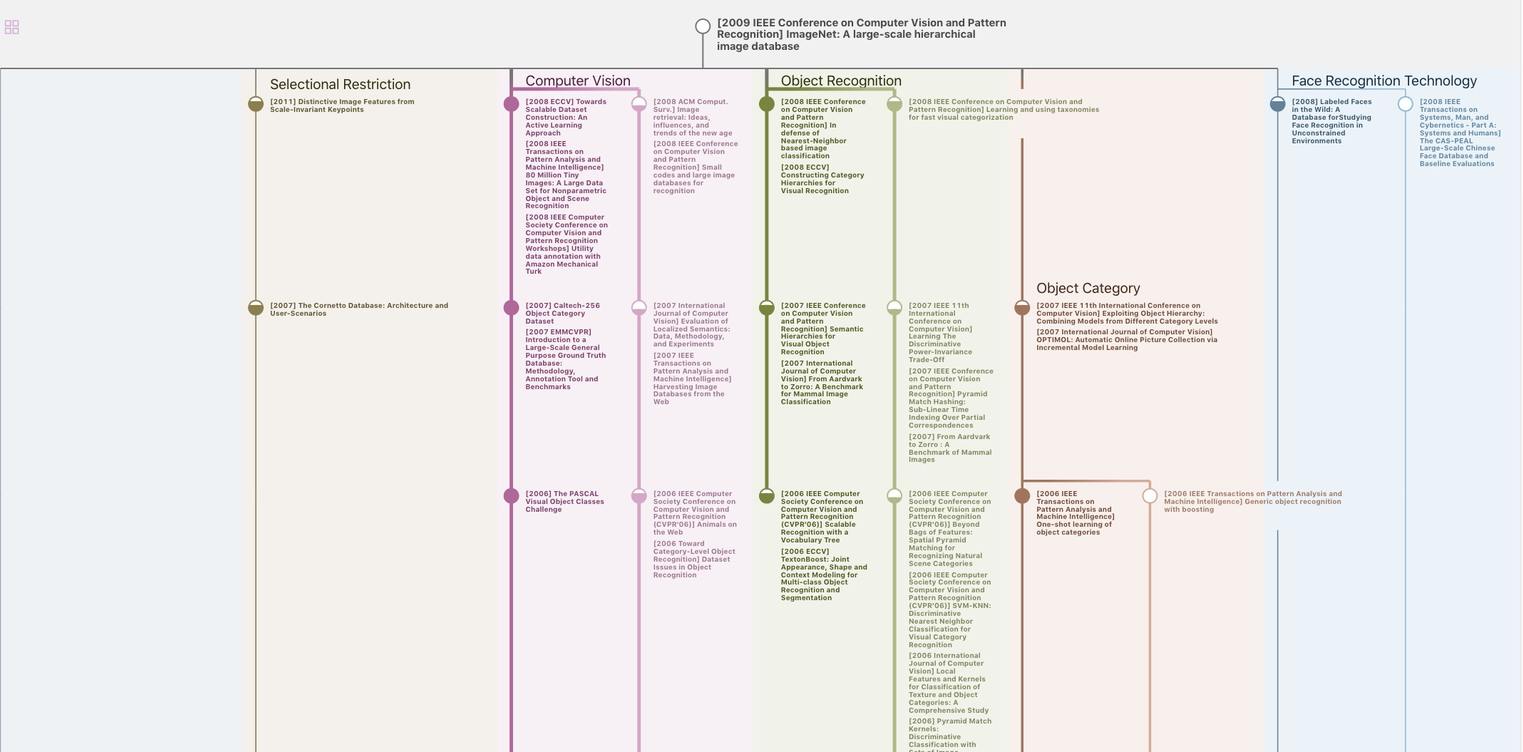
生成溯源树,研究论文发展脉络
Chat Paper
正在生成论文摘要