Efficient algorithms for compression and classification of brain tumor images
Journal of Optics(2023)
摘要
Brain tumor is an abnormal cell population that occurs in the brain. Currently, medical imaging techniques play a vital role in brain tumor diagnosis and classification. Brain tumor classification based on Magnetic Resonance Imaging (MRI) has become a promising research area in the field of medical imaging systems. In the brain image, the size of the tumor may vary from patient to patient along with the minute details of the tumor. It is a difficult task for radiologists to diagnose and classify tumors from numerous images. An efficient algorithm is proposed in this paper for tumor classification based on Deep Learning (DL) models. This paper presents three different Convolutional Neural Network (CNN) models for classification of brain tumors. These models are AlexNet, VGG16, and ResNet50. As brain images need to be stored for a along time for research and medical causes, image compression is an efficient tool for minimizing storage space, and also for allowing the deep analysis of brain images. This study depends on a lossy compression method, namely JPEG2000, for the storage of medical brain images. Classification is applied on the dataset with and without compression to estimate the effect of the compression method on the classification performance. Results of the classification models show that ResNet50 achieves a 99.97% accuracy, then VGG16 reaches a 98.83% accuracy, and finally, AlexNet gives a 92.92% accuracy without compression. The compression process is applied with four different compression ratios of 50, 25, 12.5, and 10%. The reduction in accuracy of classification with compression is small, as ResNet50 gives a 98.56% accuracy, and VGG16 gives a 92.92% accuracy, while AlexNet gives an 83.83% accuracy.
更多查看译文
关键词
Brain tumor,MRI,Deep Learning (DL),AlexNet,VGG16,ResNet50,Image compression
AI 理解论文
溯源树
样例
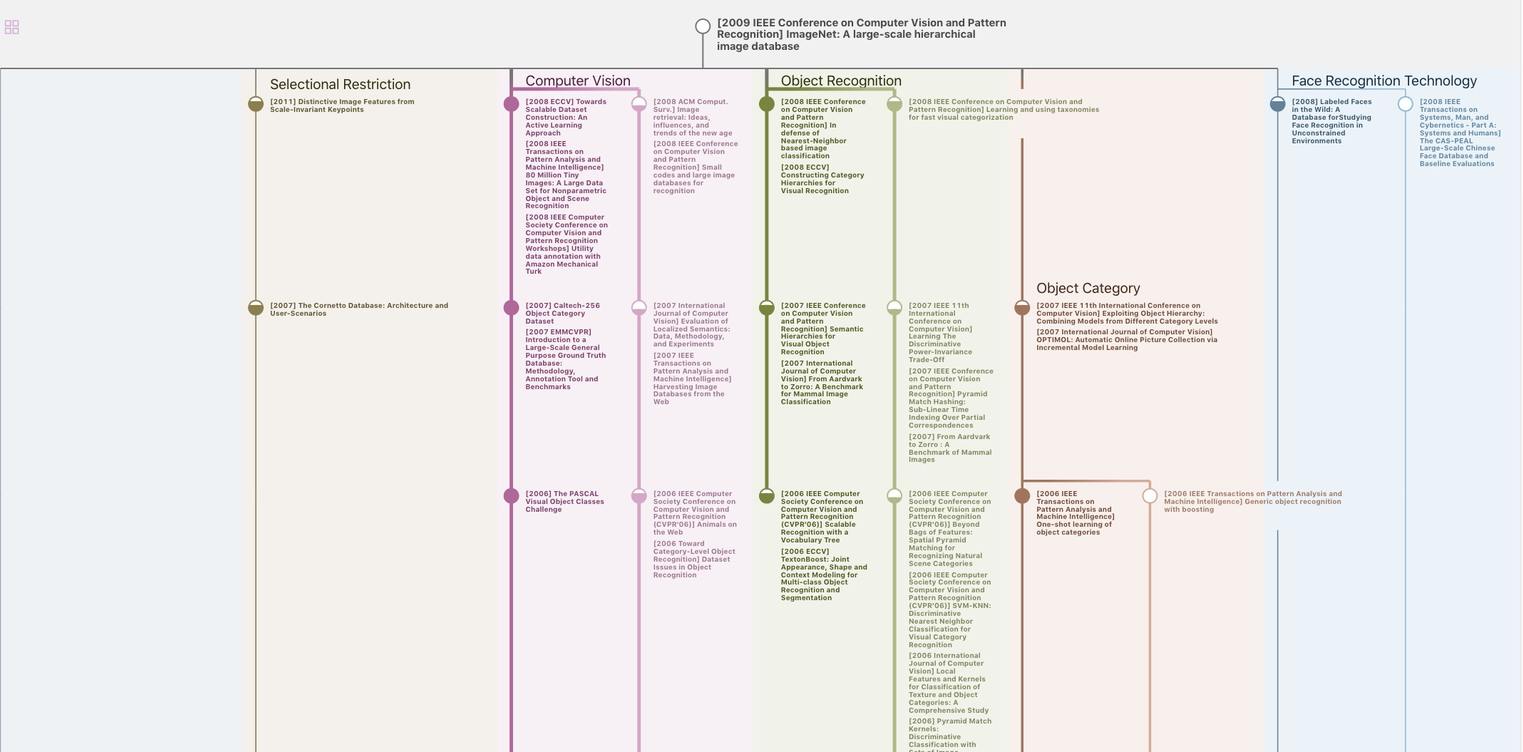
生成溯源树,研究论文发展脉络
Chat Paper
正在生成论文摘要