A Non-parametric Bayesian Approach for Uplift Discretization and Feature Selection
ECML/PKDD (5)(2023)
摘要
Uplift modeling aims to estimate the incremental impact of a treatment, such as a marketing campaign or a drug, on an individual's outcome. Bank or Telecom uplift data often have hundreds to thousands of features. In such situations, detection of irrelevant features is an essential step to reduce computational time and increase model performance. We present a parameter-free feature selection method for uplift modeling founded on a Bayesian approach. We design an automatic feature discretization method for uplift based on a space of discretization models and a prior distribution. From this model space, we define a Bayes optimal evaluation criterion of a discretization model for uplift. We then propose an optimization algorithm that finds near-optimal discretization for estimating uplift in O(n log n) time. Experiments demonstrate the high performances obtained by this new discretization method. Then we describe a parameter-free feature selection method for uplift. Experiments show that the new method both removes irrelevant features and achieves better performances than state of the art methods.
更多查看译文
关键词
Uplift modeling, Feature selection, Discretization, Bayesian methods, Machine learning, Treatment effect estimation
AI 理解论文
溯源树
样例
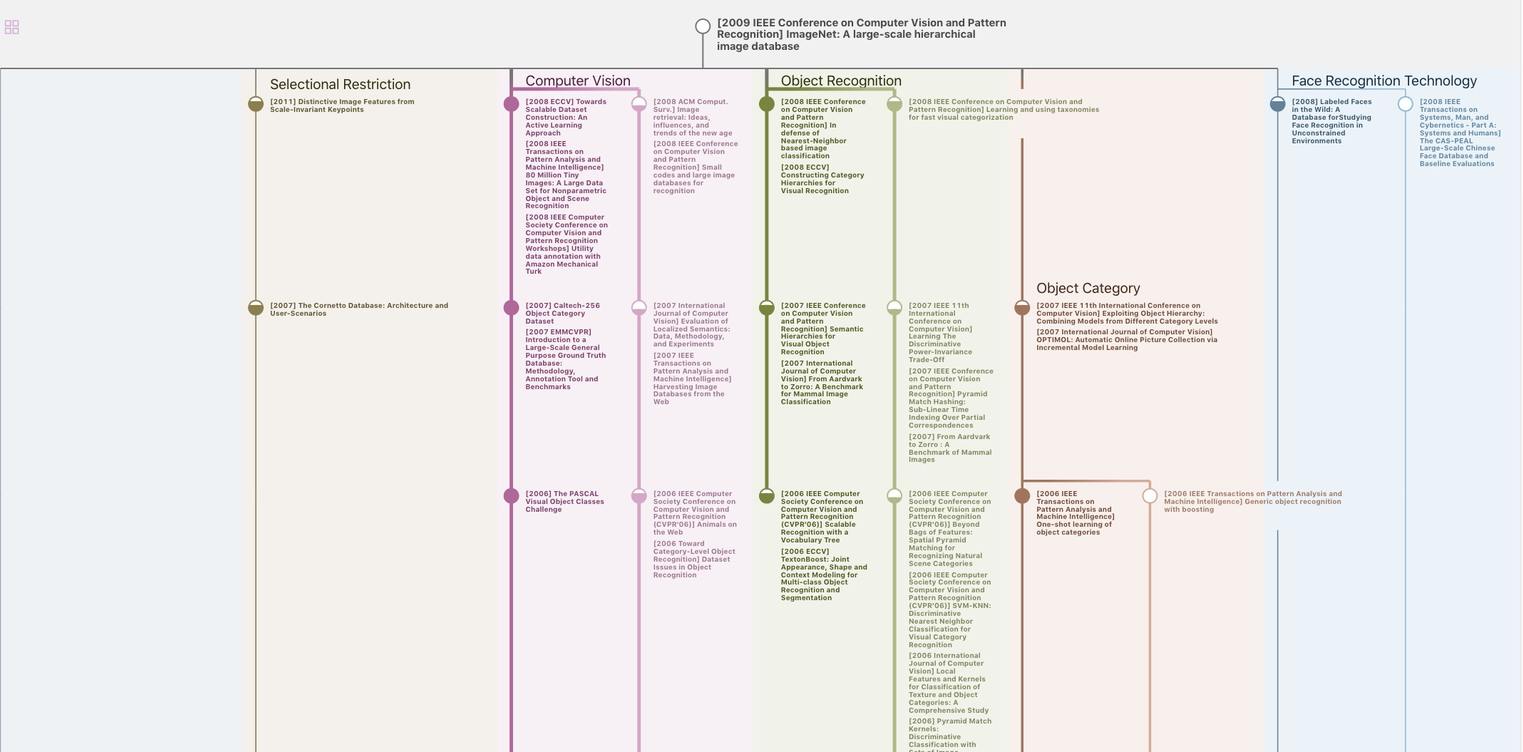
生成溯源树,研究论文发展脉络
Chat Paper
正在生成论文摘要