pFedDef: Characterizing evasion attack transferability in federated learning
Software Impacts(2023)
摘要
Federated learning jointly trains a model across multiple clients, leveraging information from all of them. However, client models are vulnerable to attacks during training and testing. We introduce the pFedDef library, which analyzes and addresses the issue of adversarial clients performing internal evasion attacks at test time to deceive other clients. pFedDef characterizes the transferability of internal evasion attacks for different learning methods and analyzes the trade-off between model accuracy and robustness to these attacks. We show that personalized federated adversarial training increases relative robustness by 60% compared to federated adversarial training and performs well even under limited system resources.
更多查看译文
关键词
Evasion attacks,Federated learning,Adversarial training,Personalized learning
AI 理解论文
溯源树
样例
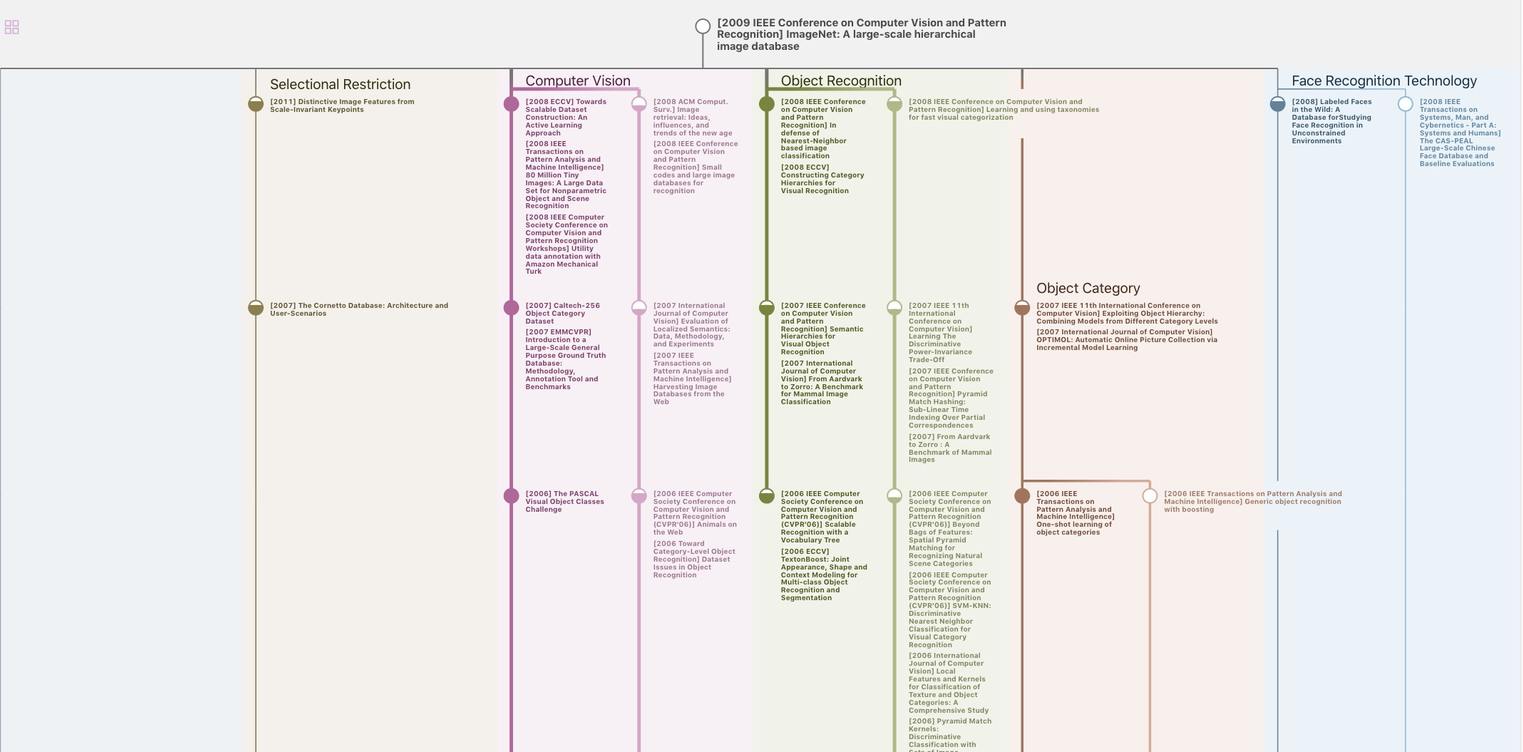
生成溯源树,研究论文发展脉络
Chat Paper
正在生成论文摘要