Spatio-Temporal Pixel-Level Contrastive Learning-Based Source-Free Domain Adaptation for Video Semantic Segmentation
CVPR 2023(2023)
摘要
Unsupervised Domain Adaptation (UDA) of semantic segmentation transfers labeled source knowledge to an unlabeled target domain by relying on accessing both the source and target data. However, the access to source data is often restricted or infeasible in real-world scenarios. Under the source data restrictive circumstances, UDA is less practical. To address this, recent works have explored solutions under the Source-Free Domain Adaptation (SFDA) setup, which aims to adapt a source-trained model to the target domain without accessing source data. Still, existing SFDA approaches use only image-level information for adaptation, making them sub-optimal in video applications. This paper studies SFDA for Video Semantic Segmentation (VSS), where temporal information is leveraged to address video adaptation. Specifically, we propose Spatio-Temporal Pixel-Level (STPL) contrastive learning, a novel method that takes full advantage of spatio-temporal information to tackle the absence of source data better. STPL explicitly learns semantic correlations among pixels in the spatio-temporal space, providing strong self-supervision for adaptation to the unlabeled target domain. Extensive experiments show that STPL achieves state-of-the-art performance on VSS benchmarks compared to current UDA and SFDA approaches. Code is available at: https://github.com/shaoyuanlo/STPL
更多查看译文
关键词
video semantic segmentation,domain adaptation,spatio-temporal,pixel-level,learning-based,source-free
AI 理解论文
溯源树
样例
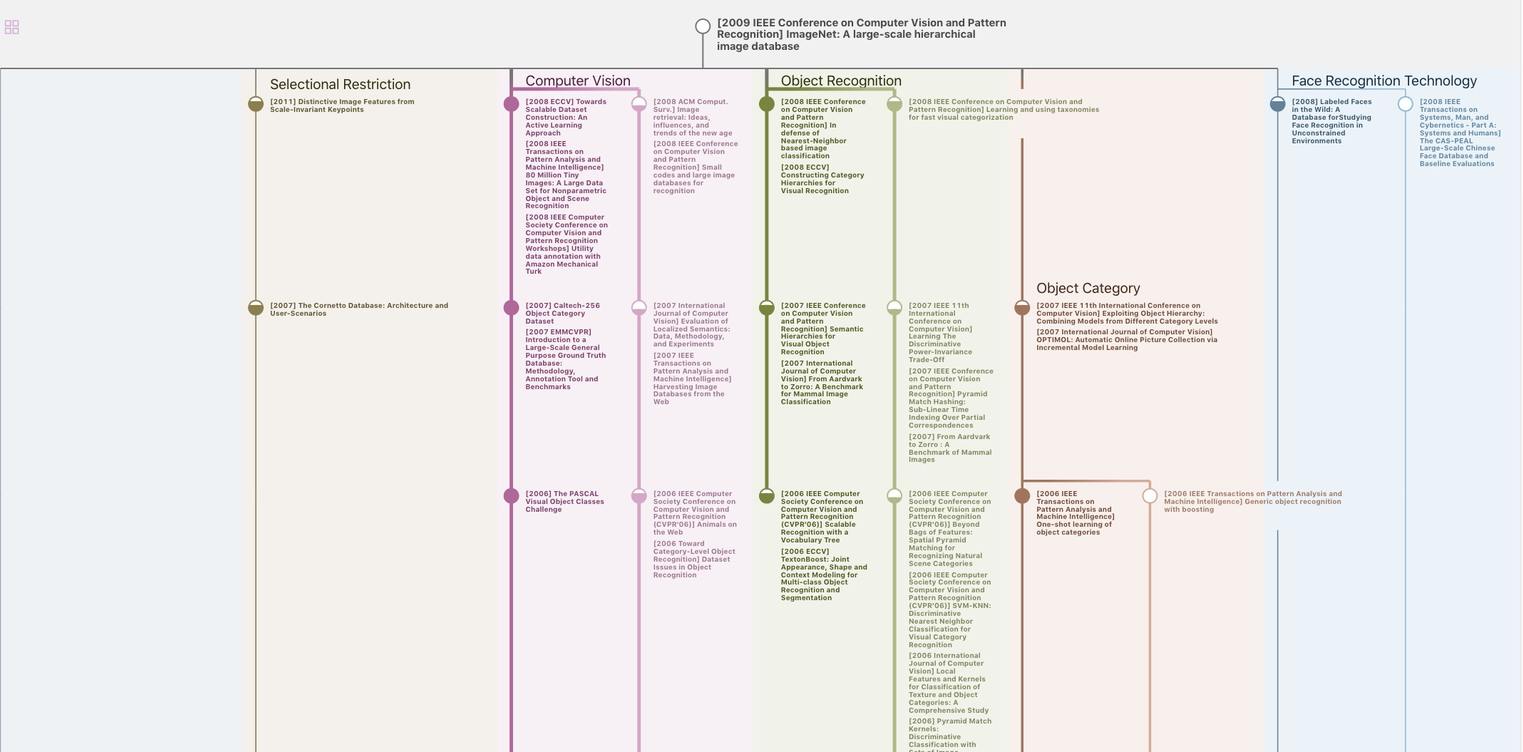
生成溯源树,研究论文发展脉络
Chat Paper
正在生成论文摘要