Theoretical bounds on the network community profile from low-rank semi-definite programming
CoRR(2023)
摘要
We study a new connection between a technical measure called $\mu$-conductance that arises in the study of Markov chains for sampling convex bodies and the network community profile that characterizes size-resolved properties of clusters and communities in social and information networks. The idea of $\mu$-conductance is similar to the traditional graph conductance, but disregards sets with small volume. We derive a sequence of optimization problems including a low-rank semi-definite program from which we can derive a lower bound on the optimal $\mu$-conductance value. These ideas give the first theoretically sound bound on the behavior of the network community profile for a wide range of cluster sizes. The algorithm scales up to graphs with hundreds of thousands of nodes and we demonstrate how our framework validates the predicted structures of real-world graphs.
更多查看译文
关键词
network community profile,theoretical bounds,low-rank,semi-definite
AI 理解论文
溯源树
样例
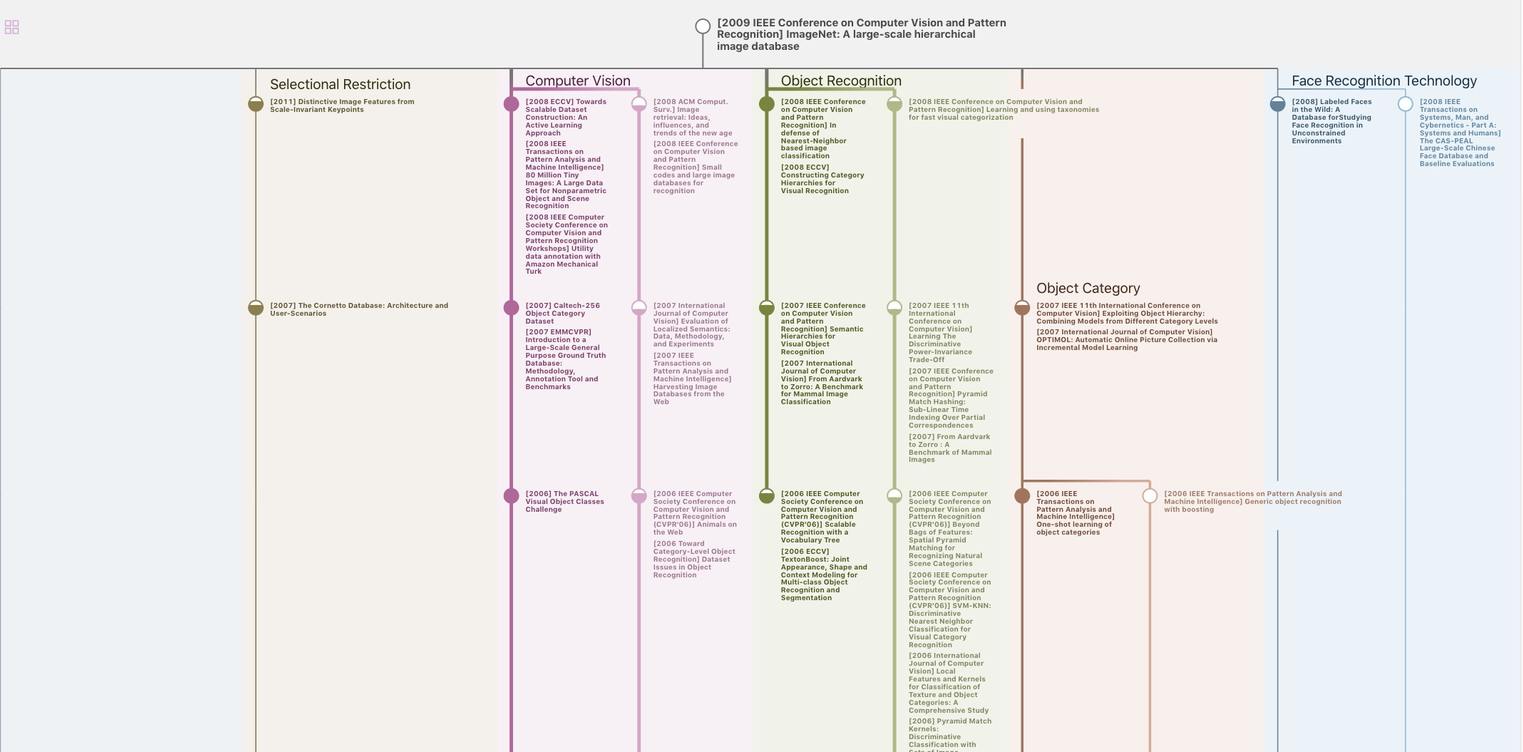
生成溯源树,研究论文发展脉络
Chat Paper
正在生成论文摘要