Robotic Packaging Optimization with Reinforcement Learning
CoRR(2023)
摘要
Intelligent manufacturing is becoming increasingly important due to the growing demand for maximizing productivity and flexibility while minimizing waste and lead times. This work investigates automated secondary robotic food packaging solutions that transfer food products from the conveyor belt into containers. A major problem in these solutions is varying product supply which can cause drastic productivity drops. Conventional rule-based approaches, used to address this issue, are often inadequate, leading to violation of the industry's requirements. Reinforcement learning, on the other hand, has the potential of solving this problem by learning responsive and predictive policy, based on experience. However, it is challenging to utilize it in highly complex control schemes. In this paper, we propose a reinforcement learning framework, designed to optimize the conveyor belt speed while minimizing interference with the rest of the control system. When tested on real-world data, the framework exceeds the performance requirements (99.8% packed products) and maintains quality (100% filled boxes). Compared to the existing solution, our proposed framework improves productivity, has smoother control, and reduces computation time.
更多查看译文
关键词
robotic packaging optimization,reinforcement learning
AI 理解论文
溯源树
样例
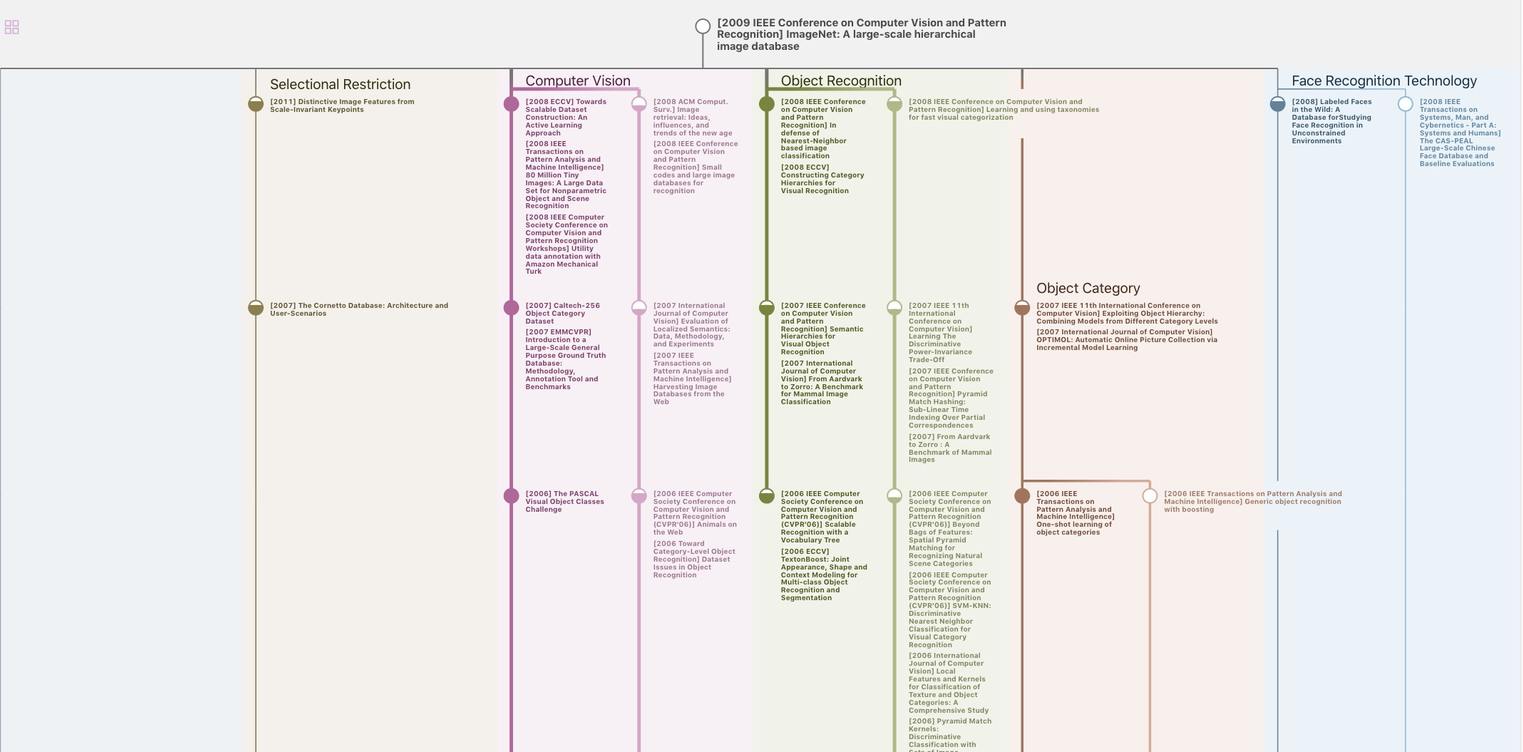
生成溯源树,研究论文发展脉络
Chat Paper
正在生成论文摘要