One Thing One Click++: Self-Training for Weakly Supervised 3D Scene Understanding
CoRR(2023)
Abstract
3D scene understanding, e.g., point cloud semantic and instance segmentation, often requires large-scale annotated training data, but clearly, point-wise labels are too tedious to prepare. While some recent methods propose to train a 3D network with small percentages of point labels, we take the approach to an extreme and propose ``One Thing One Click,'' meaning that the annotator only needs to label one point per object. To leverage these extremely sparse labels in network training, we design a novel self-training approach, in which we iteratively conduct the training and label propagation, facilitated by a graph propagation module. Also, we adopt a relation network to generate the per-category prototype to enhance the pseudo label quality and guide the iterative training. Besides, our model can be compatible to 3D instance segmentation equipped with a point-clustering strategy. Experimental results on both ScanNet-v2 and S3DIS show that our self-training approach, with extremely-sparse annotations, outperforms all existing weakly supervised methods for 3D semantic and instance segmentation by a large margin, and our results are also comparable to those of the fully supervised counterparts. Codes and models are available at https://github.com/liuzhengzhe/One-Thing-One-Click.
MoreTranslated text
AI Read Science
Must-Reading Tree
Example
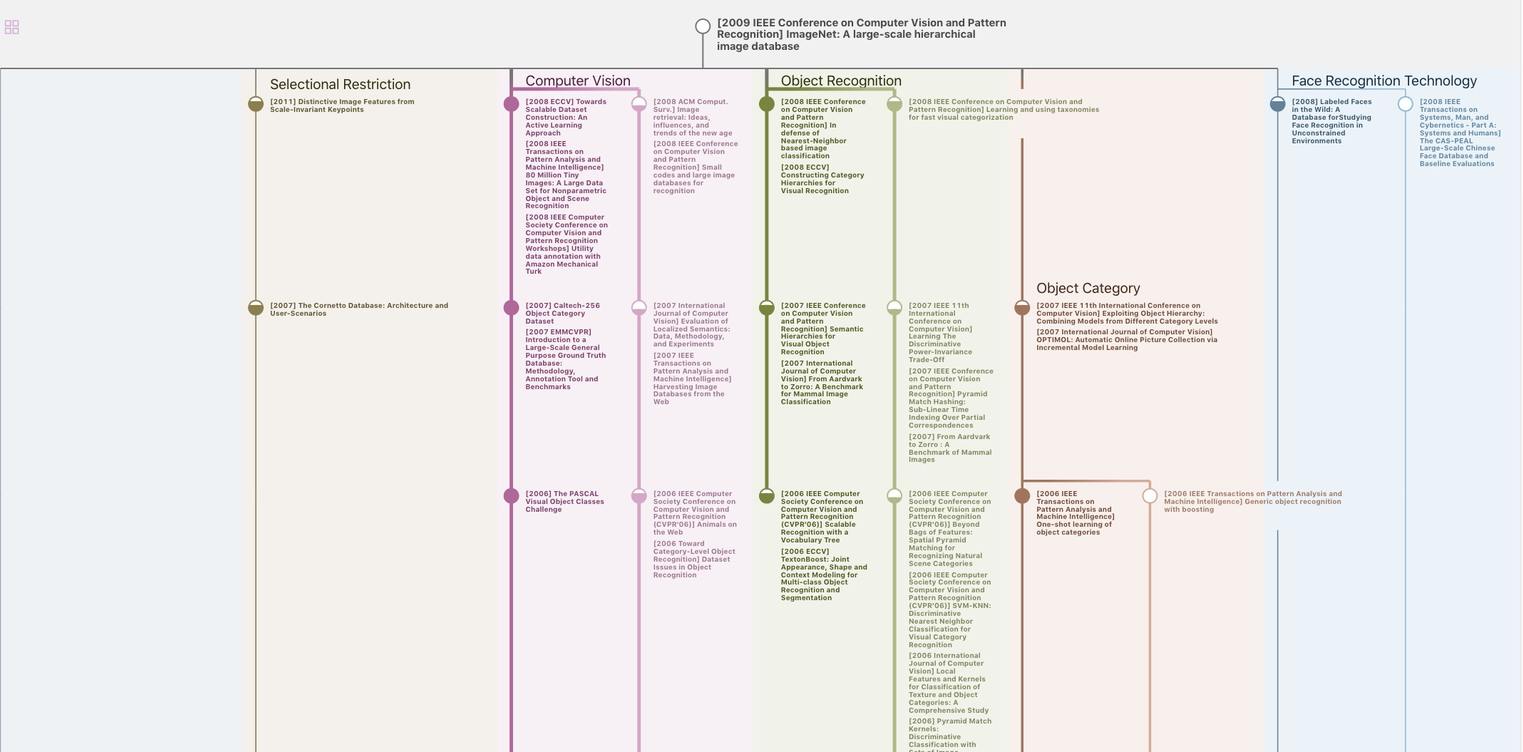
Generate MRT to find the research sequence of this paper
Chat Paper
Summary is being generated by the instructions you defined