Addressing the Challenges of Open-World Object Detection
CoRR(2023)
摘要
We address the challenging problem of open world object detection (OWOD), where object detectors must identify objects from known classes while also identifying and continually learning to detect novel objects. Prior work has resulted in detectors that have a relatively low ability to detect novel objects, and a high likelihood of classifying a novel object as one of the known classes. We approach the problem by identifying the three main challenges that OWOD presents and introduce OW-RCNN, an open world object detector that addresses each of these three challenges. OW-RCNN establishes a new state of the art using the open-world evaluation protocol on MS-COCO, showing a drastically increased ability to detect novel objects (16-21% absolute increase in U-Recall), to avoid their misclassification as one of the known classes (up to 52% reduction in A-OSE), and to incrementally learn to detect them while maintaining performance on previously known classes (1-6% absolute increase in mAP).
更多查看译文
关键词
detection,object,challenges,open-world
AI 理解论文
溯源树
样例
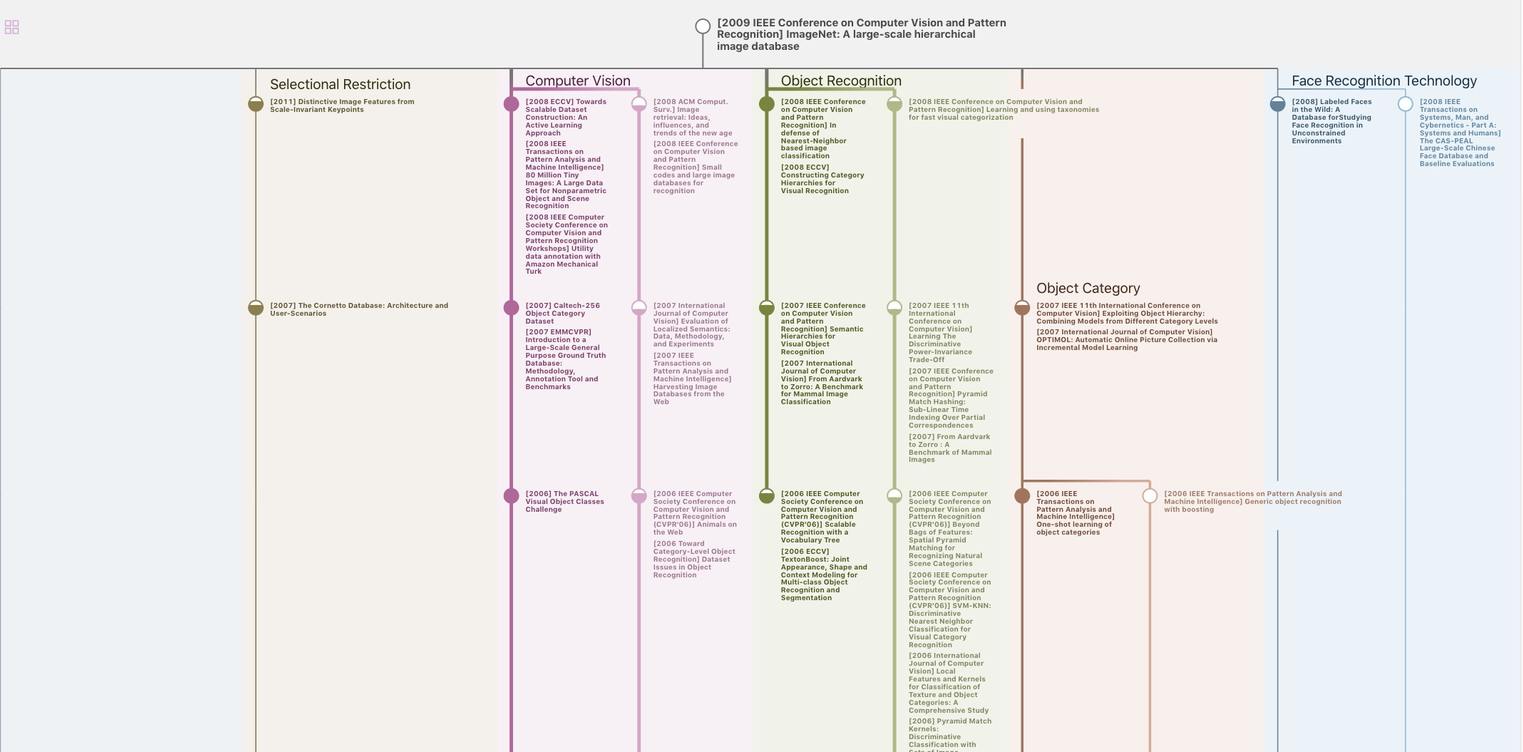
生成溯源树,研究论文发展脉络
Chat Paper
正在生成论文摘要