Intersection over Union with Smoothing for Bounding Box Regression
ADVANCES IN COMPUTATIONAL INTELLIGENCE, IWANN 2023, PT II(2023)
摘要
We focus on the construction of a loss function for the bounding box regression. The Intersection over Union (IoU) metric is improved to converge faster, to make the surface of the loss function smooth and continuous over the whole searched space, and to reach a more precise approximation of the labels. The main principle is adding a smoothing part to the original IoU, where the smoothing part is given by a linear space with values that increases from the ground truth bounding box to the border of the input image, and thus covers the whole spatial search space. We show the motivation and formalism behind this loss function and experimentally prove that it outperforms IoU, DIoU, CIoU, and SIoU by a large margin. We experimentally show that the proposed loss function is robust with respect to the noise in the dimension of ground truth bounding boxes. The reference implementation is available at https://gitlab.com/irafm-ai/smoothing-iou.
更多查看译文
关键词
Bounding box regression,Intersection over Union,Object detection,Noisy labels
AI 理解论文
溯源树
样例
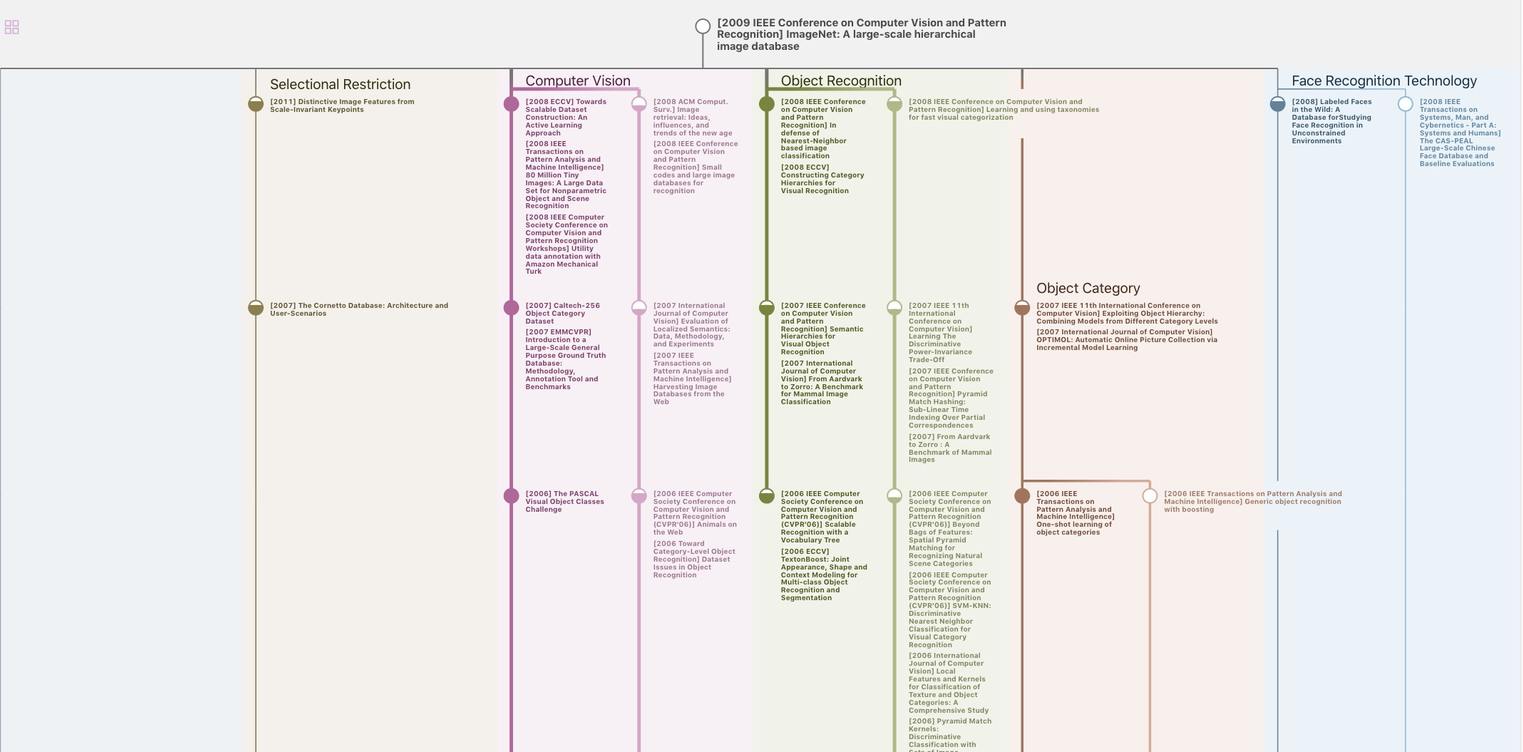
生成溯源树,研究论文发展脉络
Chat Paper
正在生成论文摘要