Single-subject Multi-contrast MRI Super-resolution via Implicit Neural Representations
MEDICAL IMAGE COMPUTING AND COMPUTER ASSISTED INTERVENTION, MICCAI 2023, PT VIII(2023)
摘要
Clinical routine and retrospective cohorts commonly include multi-parametric Magnetic Resonance Imaging; however, they are mostly acquired in different anisotropic 2D views due to signal-to-noise-ratio and scan-time constraints. Thus acquired views suffer from poor out-of-plane resolution and affect downstream volumetric image analysis that typically requires isotropic 3D scans. Combining different views of multi-contrast scans into high-resolution isotropic 3D scans is challenging due to the lack of a large training cohort, which calls for a subject-specific framework. This work proposes a novel solution to this problem leveraging Implicit Neural Representations (INR). Our proposed INR jointly learns two different contrasts of complementary views in a continuous spatial function and benefits from exchanging anatomical information between them. Trained within minutes on a single commodity GPU, our model provides realistic super-resolution across different pairs of contrasts in our experiments with three datasets. Using Mutual Information (MI) as a metric, we find that our model converges to an optimum MI amongst sequences, achieving anatomically faithful reconstruction. (Code is available at: https://github.com/jqmcginnis/multi_contrast_inr/).
更多查看译文
关键词
Multi-contrast Super-resolution,Implicit Neural Representations,Mutual Information
AI 理解论文
溯源树
样例
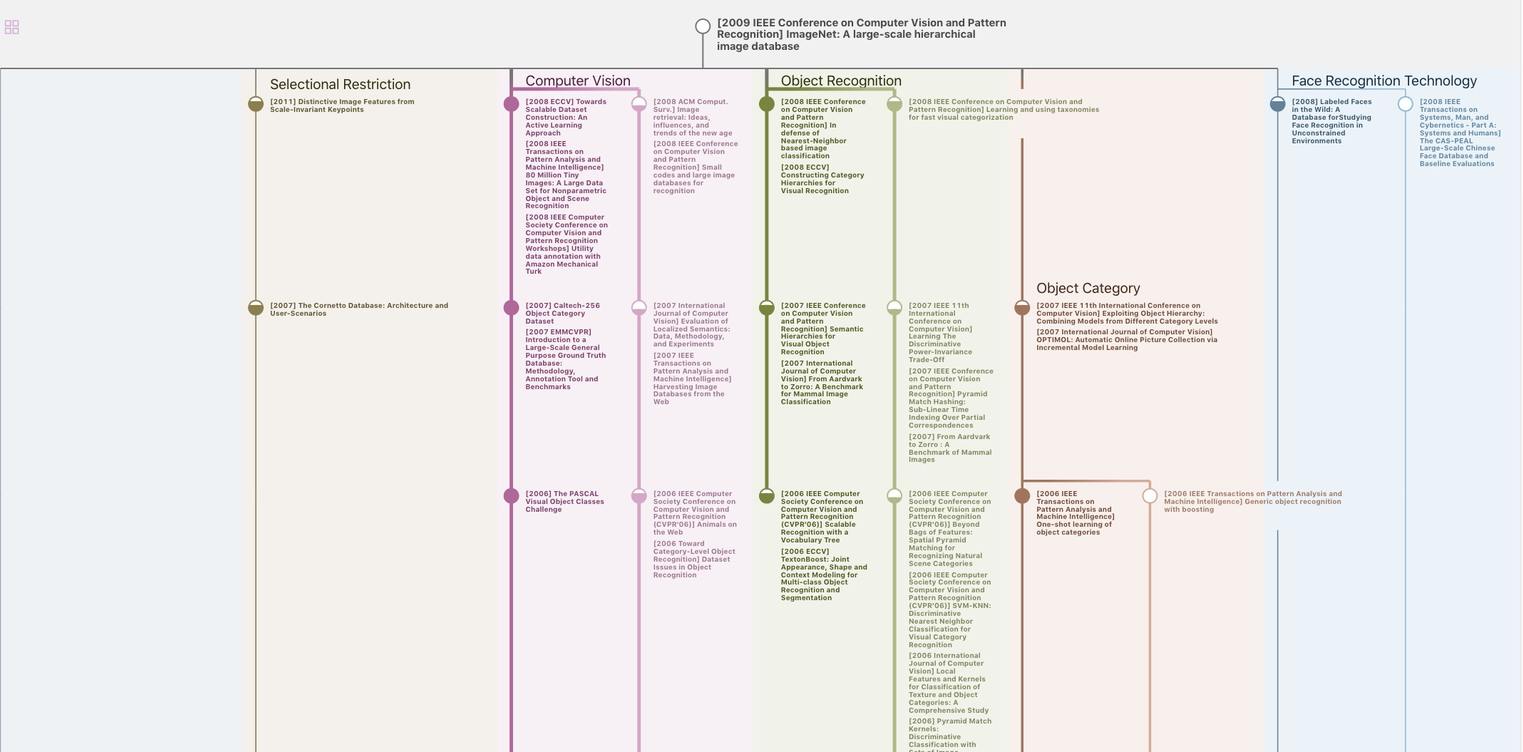
生成溯源树,研究论文发展脉络
Chat Paper
正在生成论文摘要