Bivariate quantitative Bayesian LASSO for detecting association of rare haplotypes with two correlated continuous phenotypes
Frontiers in genetics(2023)
摘要
In genetic association studies, the multivariate analysis of correlated phenotypes offers statistical and biological advantages compared to analyzing one phenotype at a time. The joint analysis utilizes additional information contained in the correlation and avoids multiple testing. It also provides an opportunity to investigate and understand shared genetic mechanisms of multiple phenotypes. Bivariate logistic Bayesian LASSO (LBL) was proposed earlier to detect rare haplotypes associated with two binary phenotypes or one binary and one continuous phenotype jointly. There is currently no haplotype association test available that can handle multiple continuous phenotypes. In this study, by employing the framework of bivariate LBL, we propose bivariate quantitative Bayesian LASSO (QBL) to detect rare haplotypes associated with two continuous phenotypes. Bivariate QBL removes unassociated haplotypes by regularizing the regression coefficients and utilizing a latent variable to model correlation between two phenotypes. We carry out extensive simulations to investigate the performance of bivariate QBL and compare it with that of a standard (univariate) haplotype association test, Haplo.score (applied twice to two phenotypes individually). Bivariate QBL performs better than Haplo.score in all simulations with varying degrees of power gain. We analyze Genetic Analysis Workshop 19 exome sequencing data on systolic and diastolic blood pressures and detect several rare haplotypes associated with the two phenotypes.
更多查看译文
关键词
Genetic Analysis Workshop 19,Markov chain Monte Carlo (MCMC),diastolic blood pressure (DBP),regularization,systolic blood pressure (SBP)
AI 理解论文
溯源树
样例
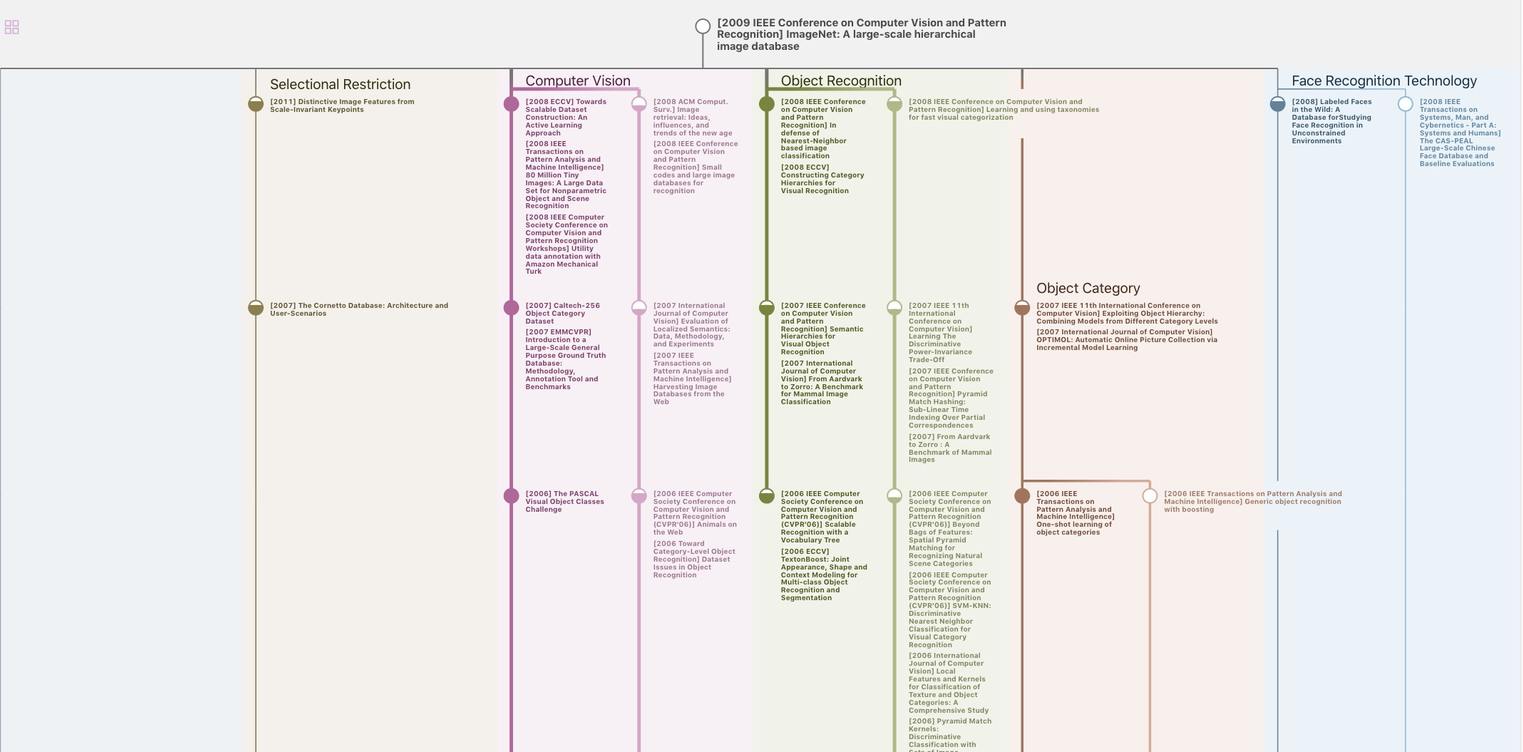
生成溯源树,研究论文发展脉络
Chat Paper
正在生成论文摘要