Composite Attention Residual U-Net for Rib Fracture Detection.
Entropy(2023)
摘要
Computed tomography (CT) images play a vital role in diagnosing rib fractures and determining the severity of chest trauma. However, quickly and accurately identifying rib fractures in a large number of CT images is an arduous task for radiologists. We propose a U-net-based detection method designed to extract rib fracture features at the pixel level to find rib fractures rapidly and precisely. Two modules are applied to the segmentation network-a combined attention module (CAM) and a hybrid dense dilated convolution module (HDDC). The features of the same layer of the encoder and the decoder are fused through CAM, strengthening the local features of the subtle fracture area and increasing the edge features. HDDC is used between the encoder and decoder to obtain sufficient semantic information. Experiments show that on the public dataset, the model test brings the effects of Recall (81.71%), F1 (81.86%), and Dice (53.28%). Experienced radiologists reach lower false positives for each scan, whereas they have underperforming neural network models in terms of detection sensitivities with a long time diagnosis. With the aid of our model, radiologists can achieve higher detection sensitivities than computer-only or human-only diagnosis.
更多查看译文
关键词
CT,U-net,deep learning,rib fractures
AI 理解论文
溯源树
样例
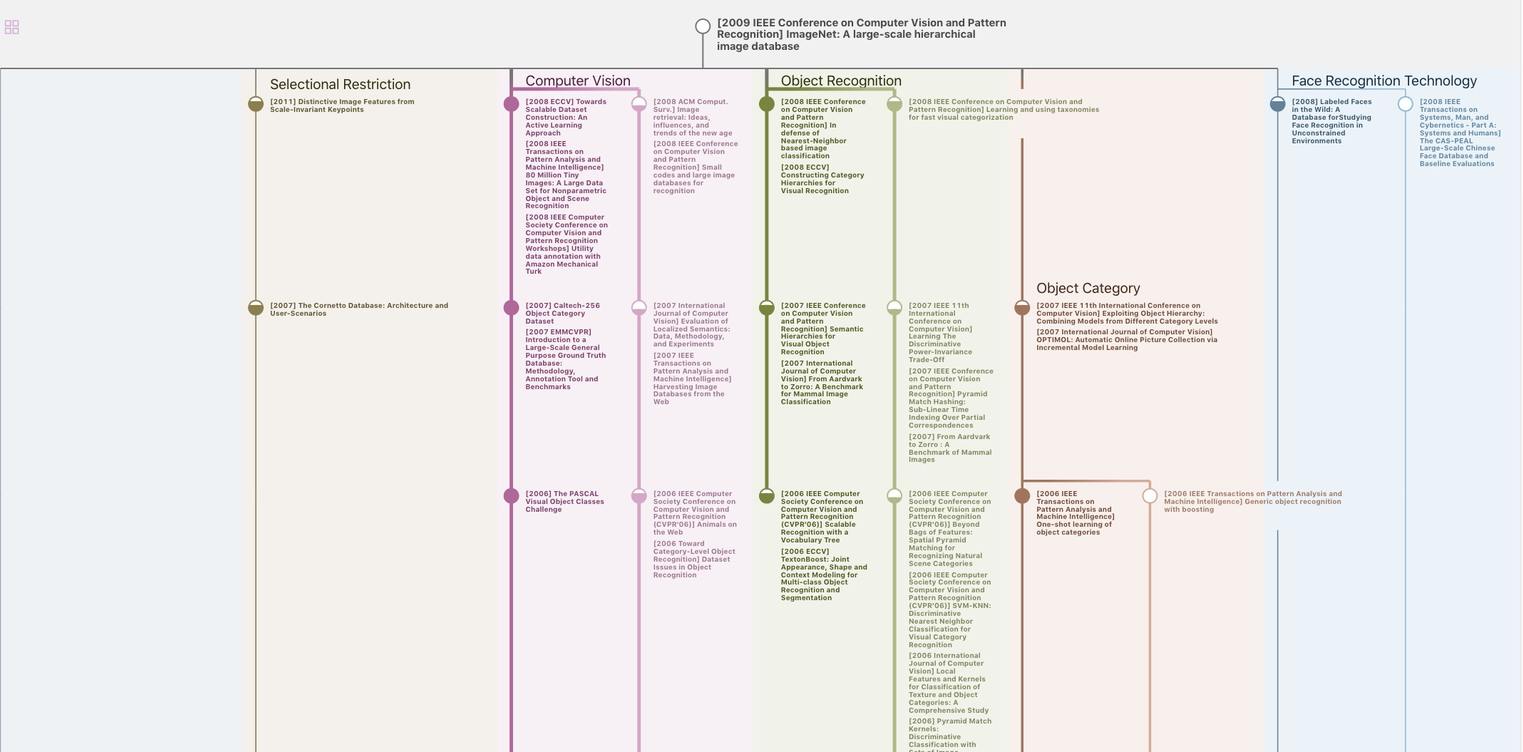
生成溯源树,研究论文发展脉络
Chat Paper
正在生成论文摘要