Sequential training of GANs against GAN-classifiers reveals correlated "knowledge gaps" present among independently trained GAN instances
CoRR(2023)
摘要
Modern Generative Adversarial Networks (GANs) generate realistic images remarkably well. Previous work has demonstrated the feasibility of "GAN-classifiers" that are distinct from the co-trained discriminator, and operate on images generated from a frozen GAN. That such classifiers work at all affirms the existence of "knowledge gaps" (out-of-distribution artifacts across samples) present in GAN training. We iteratively train GAN-classifiers and train GANs that "fool" the classifiers (in an attempt to fill the knowledge gaps), and examine the effect on GAN training dynamics, output quality, and GAN-classifier generalization. We investigate two settings, a small DCGAN architecture trained on low dimensional images (MNIST), and StyleGAN2, a SOTA GAN architecture trained on high dimensional images (FFHQ). We find that the DCGAN is unable to effectively fool a heldout GAN-classifier without compromising the output quality. However, the StyleGAN2 can fool held-out classifiers with no change in output quality, and this effect persists over multiple rounds of GAN/classifier training which appears to reveal an ordering over optima in the generator parameter space. Finally, we study different classifier architectures and show that the architecture of the GAN-classifier has a strong influence on the set of its learned artifacts.
更多查看译文
关键词
gans,knowledge gaps,sequential training,gan-classifiers
AI 理解论文
溯源树
样例
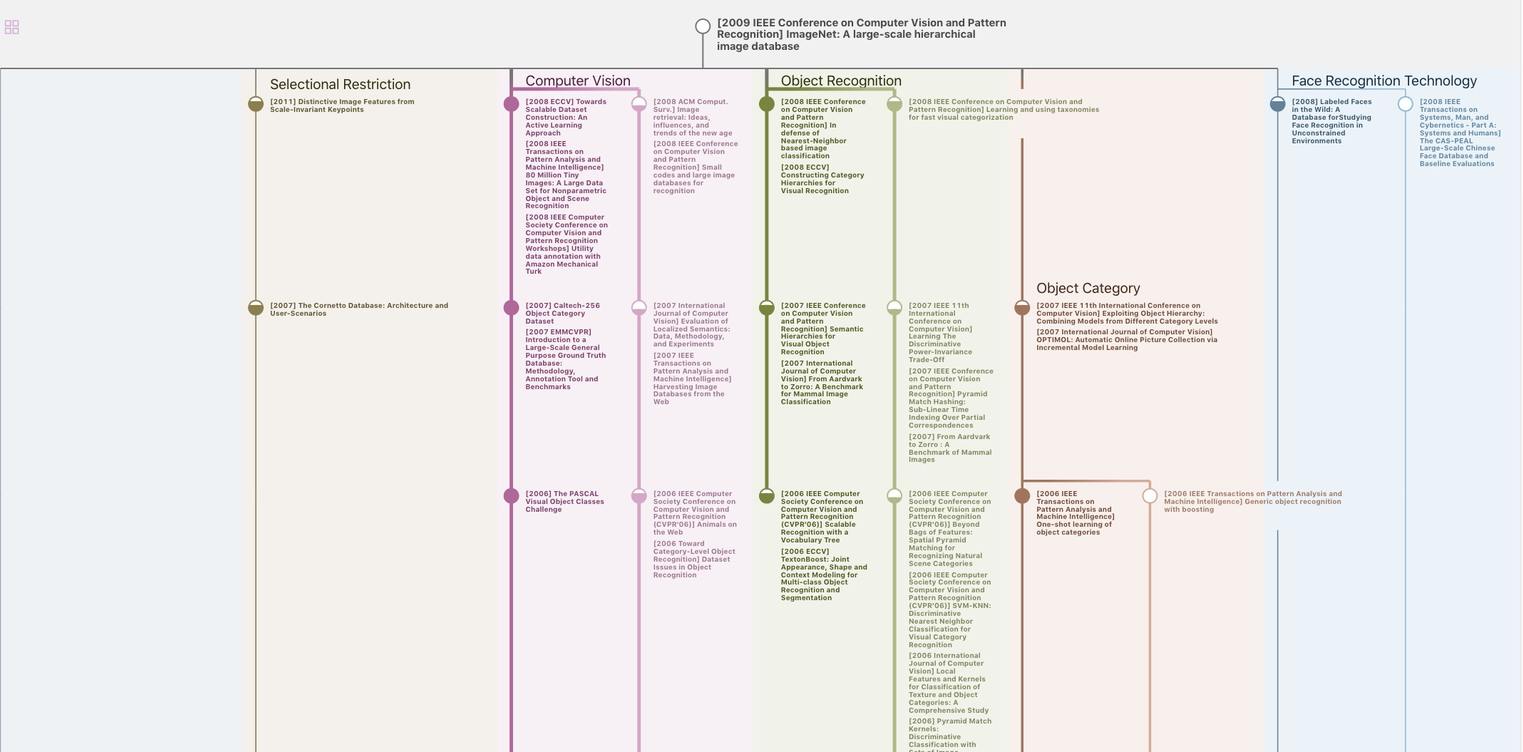
生成溯源树,研究论文发展脉络
Chat Paper
正在生成论文摘要