Explicit Attention-Enhanced Fusion for RGB-Thermal Perception Tasks
CoRR(2023)
摘要
Recently, RGB-Thermal based perception has shown significant advances. Thermal information provides useful clues when visual cameras suffer from poor lighting conditions, such as low light and fog. However, how to effectively fuse RGB images and thermal data remains an open challenge. Previous works involve naive fusion strategies such as merging them at the input, concatenating multi-modality features inside models, or applying attention to each data modality. These fusion strategies are straightforward yet insufficient. In this letter, we propose a novel fusion method named Explicit Attention-Enhanced Fusion (EAEF) that fully takes advantage of each type of data. Specifically, we consider the following cases: i) both RGB data and thermal data, ii) only one of the types of data, and iii) none of them generate discriminative features. EAEF uses one branch to enhance feature extraction for i) and iii) and the other branch to remedy insufficient representations for ii). The outputs of two branches are fused to form complementary features. As a result, the proposed fusion method outperforms state-of-the-art by 1.6% in mIoU on semantic segmentation, 3.1% in MAE on salient object detection, 2.3% in mAP on object detection, and 8.1% in MAE on crowd counting.
更多查看译文
关键词
Feature extraction,Task analysis,Data mining,Fuses,Semantic segmentation,Decoding,Object detection,Multi-modality data fusion,RGB-thermal fusion,RGB-thermal perception
AI 理解论文
溯源树
样例
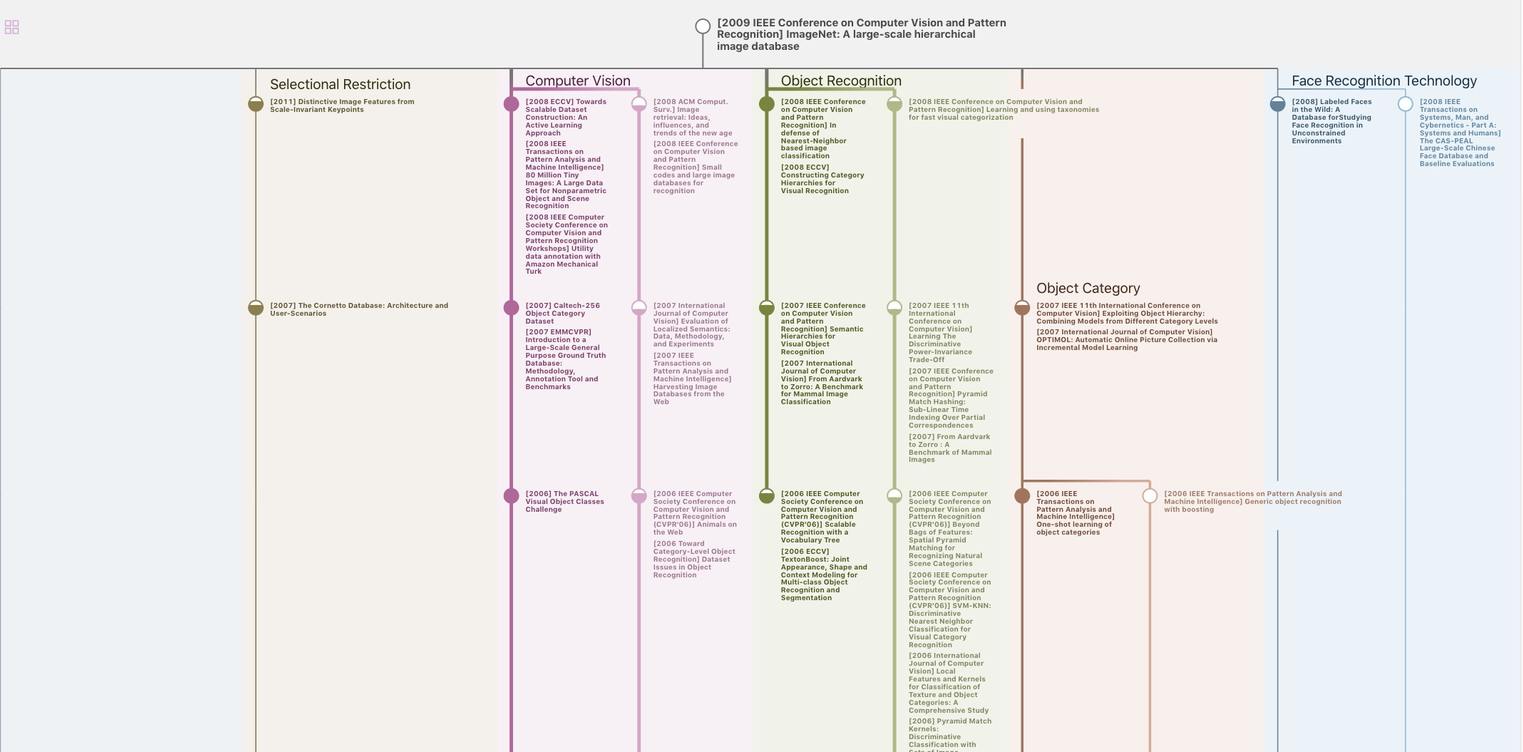
生成溯源树,研究论文发展脉络
Chat Paper
正在生成论文摘要