Unsupervised anomaly detection using graph neural networks integrated with physical-statistical feature fusion and local-global learning
RENEWABLE ENERGY(2023)
摘要
Efficient and feasible anomaly detection scheme that could utilize data collected by supervisory-control-anddata-acquisition (SCADA) system is essential for wind turbines, which could greatly increase the amount of available condition monitoring data, and improve the power generation efficiency and reduce maintenance costs. While it is also very challenging as condition estimation with such massive field data is difficult to tackle, especially the SCADA data is not labeled in most cases. This work presents an unsupervised anomaly detection framework for wind turbines incorporating physical-statistical feature fusion and graph neural networks (GNNs), realizing dimensionality reduction, temporal dependence extraction, and latent nonlinear correlation capture of high-dimensional data. Firstly, graphical modeling for SCADA data of wind turbines is presented. Secondly, the physical-statistical feature fusion is implemented via local-global mutual information maximization. Finally, anomaly detection is realized with an energy-based method to learn patterns in the updated nodes' feature matrix. The results show that i) the features designed by physical information can reasonably represent equipment's state and reduce the information-redundancy, ii) the time-series based graph structure can effectively express the dataset structure information and extract temporal dependence, iii) and the anomaly detection model can fully use the physical-statistical information and local-global information, which outperforms comparison methods.
更多查看译文
关键词
Wind turbine,Anomaly detection,Feature fusion,Supervisory control and data acquisition,Graph neural networks
AI 理解论文
溯源树
样例
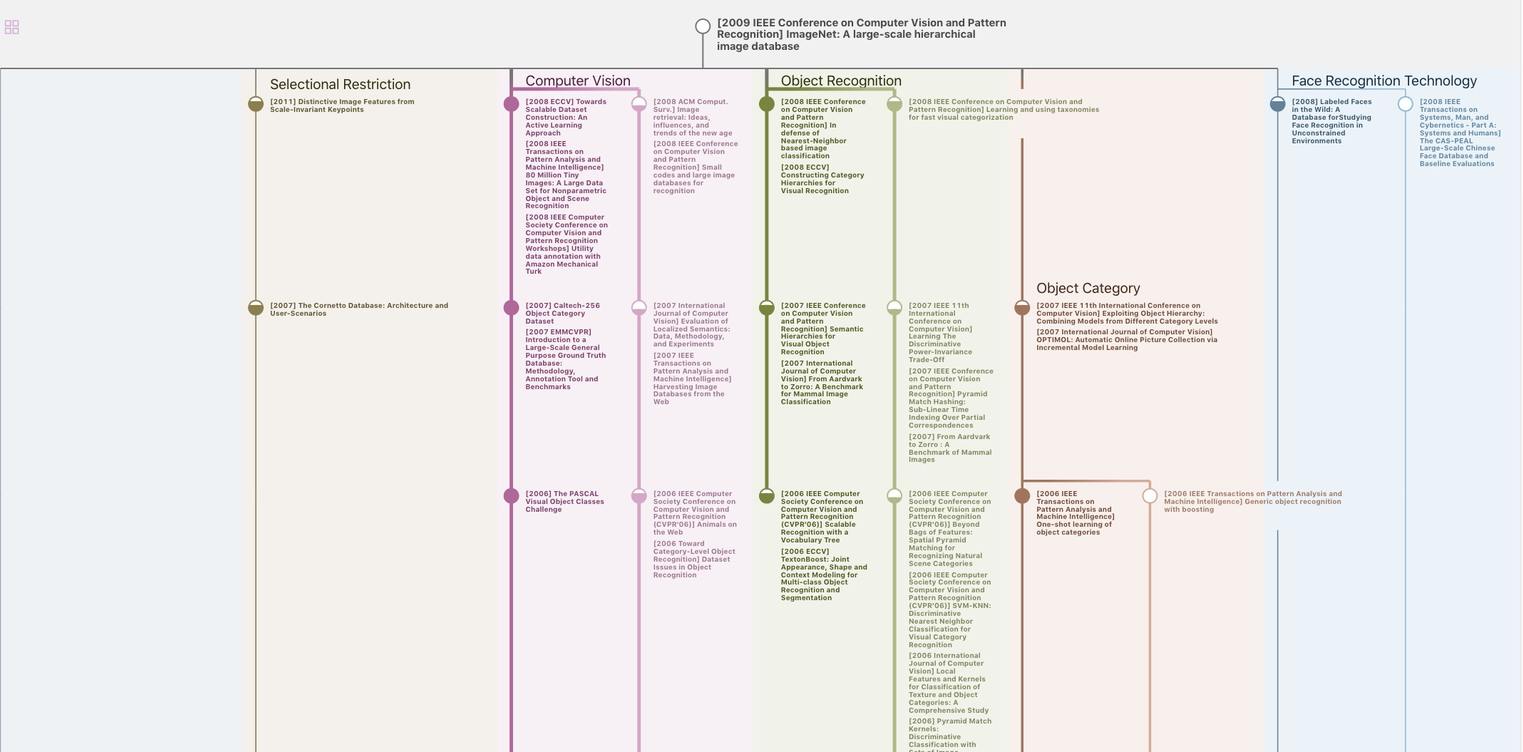
生成溯源树,研究论文发展脉络
Chat Paper
正在生成论文摘要