A novel method for real-time ATR system of AUV based on Attention-MobileNetV3 network and pixel correction algorithm
Ocean Engineering(2023)
摘要
This paper proposes an Automatic Target Recognition (ATR) system based on the Autonomous Underwater Vehicle (AUV) equipped with side scan sonar for real-time detection and positioning of underwater targets. The system introduces a pre-processing algorithm for the original Side Scan Sonar (SSS) image, which performs pixel-level correction of the image according to the slant range setting of the SSS sensor and the height from the seabed to eliminate the influence of target geometric distortion on the subsequent recognition algorithm and improve the recognition accuracy. At the same time, the attitude angle information of the AUV is obtained to correct the target position, making each pixel of the pre-processed image has underwater positioning (latitude and longitude) information. Since SSS images are seriously disturbed by noise, in order to accurately extract target features in real time, on the basis of selecting the lightweight network MobileNetV3, we introduce attention mechanism and integrated learning to meet the requirements of underwater target recognition accuracy and real-time performance. The multi-classifier ensemble prediction results further reduce the mis-identification rate of submarine targets. The ATR system fuses the prediction results and the submarine target position and sends it to the AUV main control system to guide the AUV how to navigate and plan path at the subsequent. The experimental results show that, we validate that the proposed method matches the state of the art in segmentation accuracy, the ATR system proposed in this paper can be embedded in the underwater unmanned platform, and achieve accurate underwater target detection and positioning, The real-time recognition speed of each picture is about 0.058 s.
更多查看译文
关键词
AUV,ATR system,Side Scan sonar,Pixel correction,Real time recognition,Underwater target location
AI 理解论文
溯源树
样例
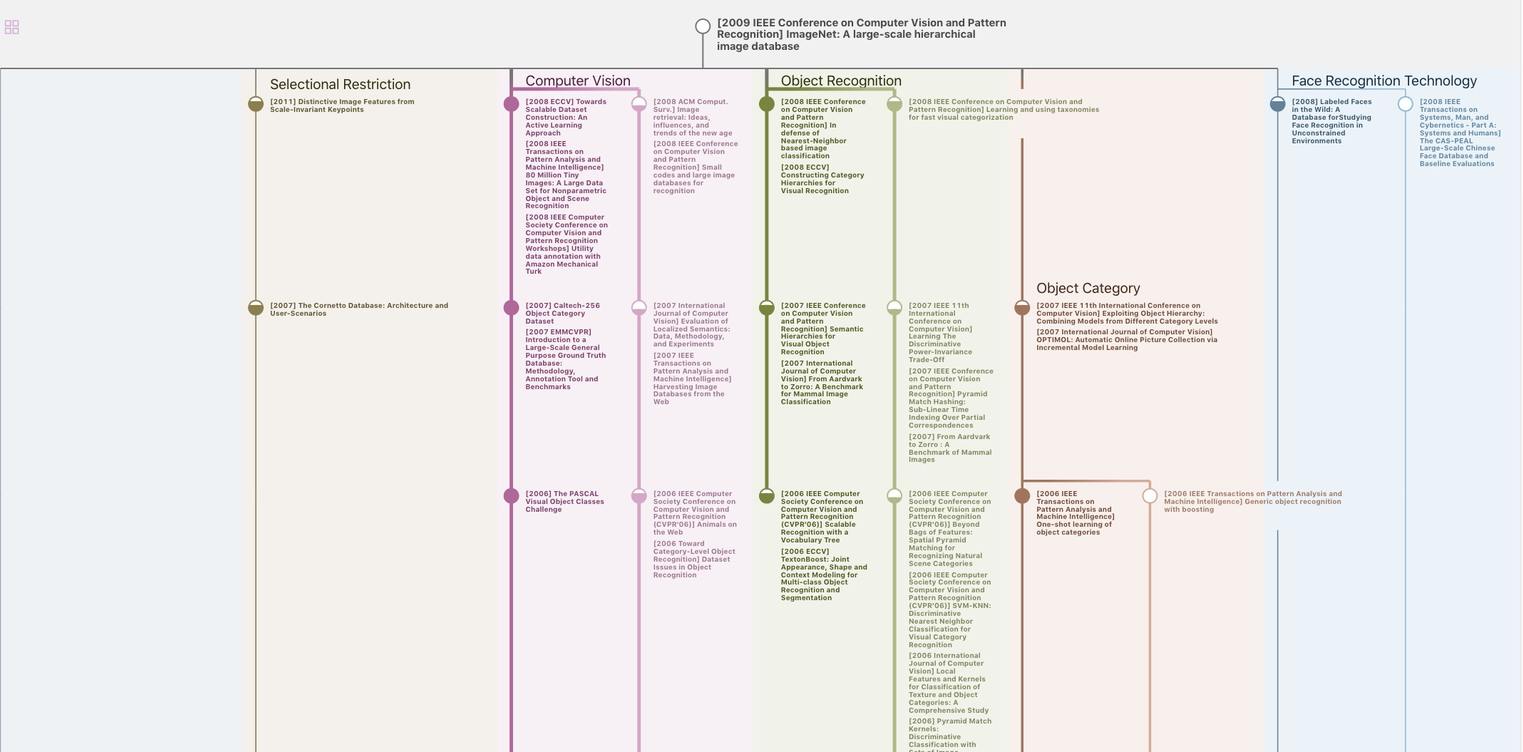
生成溯源树,研究论文发展脉络
Chat Paper
正在生成论文摘要