A time-varying ensemble model for ship motion prediction based on feature selection and clustering methods
Ocean Engineering(2023)
摘要
Effective ship motion prediction helps to avoid ship navigation hazards in time. However, there is insufficient attention to potentially valuable information in the multi-factor ship-related data space and the time-varying characteristics of ship motion. In this paper, a time-varying ensemble model based on feature selection and clustering methods is proposed to improve the performance of real-time ship motion prediction. The ensemble model consists of three modules that progressively improve the validity of the model. In Module I, the non-dominated sorting genetic algorithm-II (NSGA-II) algorithm is adopted for feature selection of the original multi-factor data to filter out the feature factors that are useful for ship motion prediction. In Module II, the self-organizing map (SOM) algorithm is applied to cluster the multi-factor data after feature selection to reorganize samples with similar attributes into a limited number of clusters. Then, an ensemble learning model is constructed for each cluster using multiple Elman neural networks and Adaboost techniques. In Module III, a time-varying prediction framework is proposed for real-time prediction of ship motion by combining the ensemble model corresponding to each cluster. An empirical study was conducted using three multifactorial datasets collected from a ship in the South China Sea in December 2020. The results show that the proposed model can be a competitive technique for solving complex multi-factor ship motion predictions and has the potential to be applied to real-time ship motion warning systems.
更多查看译文
关键词
Ship motion prediction,Time-varying ensemble model,Feature selection and clustering methods,Adaboost technique
AI 理解论文
溯源树
样例
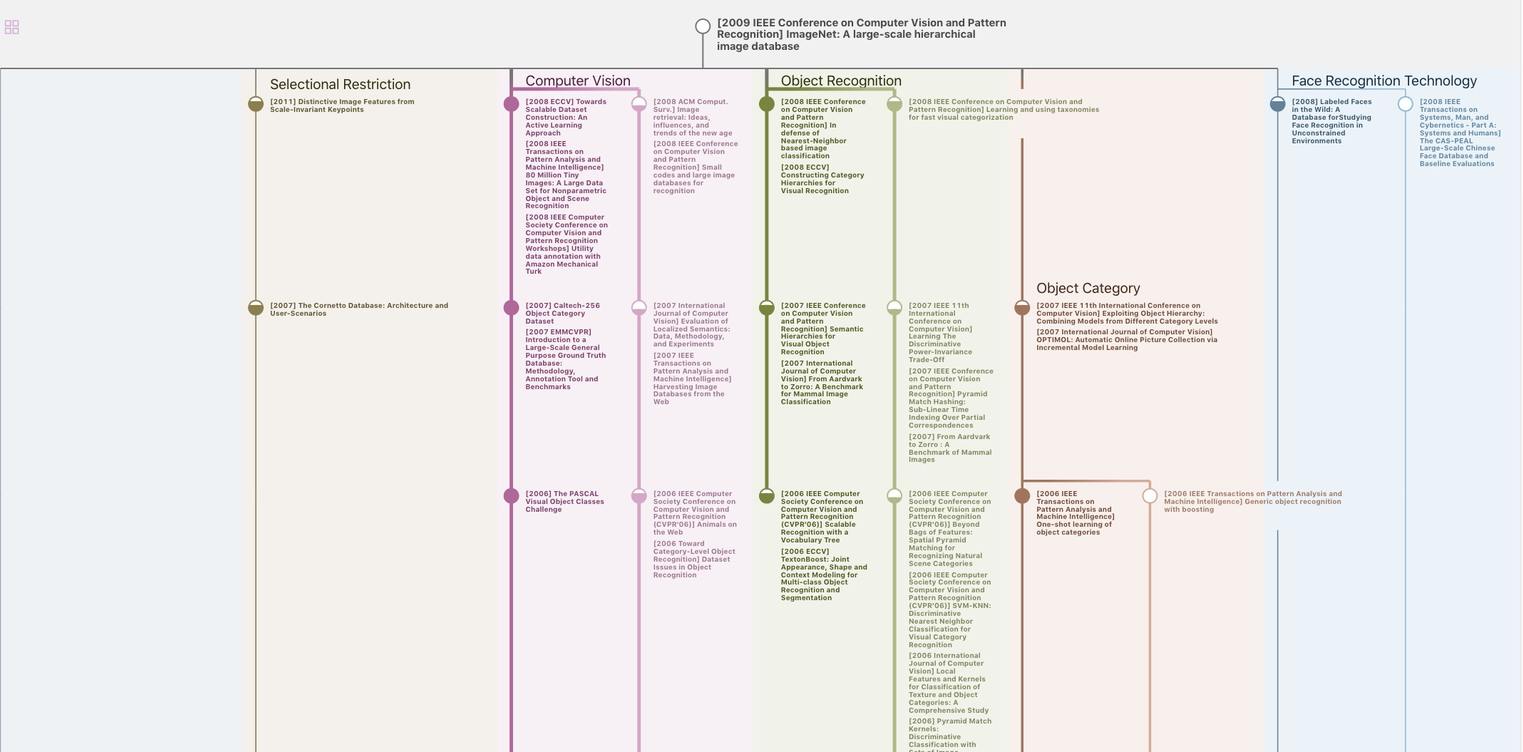
生成溯源树,研究论文发展脉络
Chat Paper
正在生成论文摘要