Convergence of Momentum-Based Heavy Ball Method with Batch Updating And/or Approximate Gradients
2023 NINTH INDIAN CONTROL CONFERENCE, ICC(2023)
摘要
In this paper, we study the well-known "Heavy Ball" method for convex and nonconvex optimization introduced by Polyak in 1964, and establish its convergence under a variety of situations. Traditionally, most algorithms use "full-coordinate update," that is, at each step, every component of the argument is updated. However, when the dimension of the argument is very high, it is more efficient to update some but not all components of the argument at each iteration. We refer to this as "batch updating" in this paper. When gradient-based algorithms are used together with batch updating, in principle it is sufficient to compute only those components of the gradient for which the argument is to be updated. However, if a method such as backpropagation is used to compute these components, computing only some components of gradient does not offer much savings over computing the entire gradient. Therefore, to achieve a noticeable reduction in CPU usage at each step, one can use first-order differences to approximate the gradient. The resulting estimates are biased, and also have unbounded variance. Thus some delicate analysis is required. In this paper, we establish the almost sure convergence of the iterations to the stationary point(s) of the objective function under suitable conditions when either noisy of approximate gradients are used.
更多查看译文
AI 理解论文
溯源树
样例
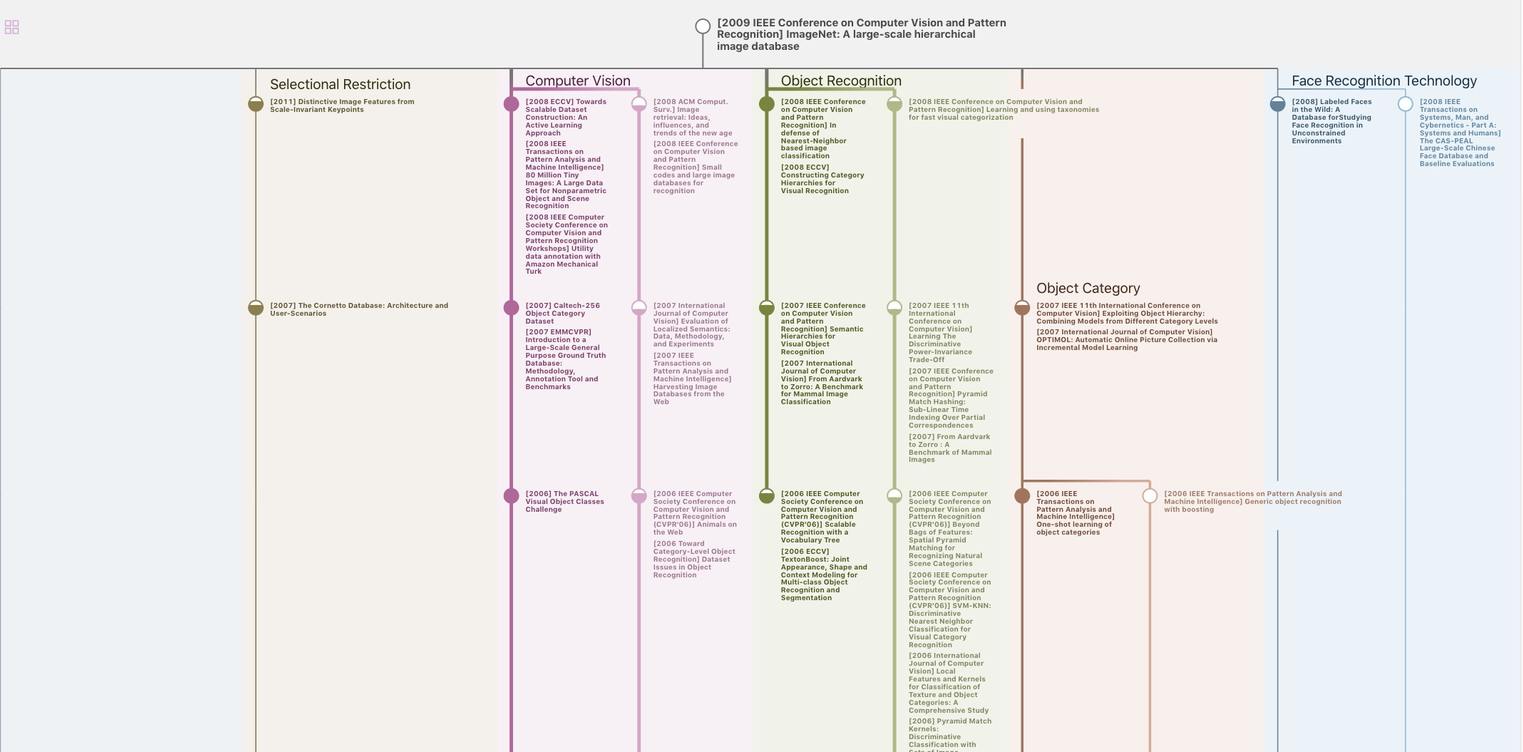
生成溯源树,研究论文发展脉络
Chat Paper
正在生成论文摘要