Boosting Few-Shot Text Classification via Distribution Estimation
CoRR(2023)
摘要
Distribution estimation has been demonstrated as one of the most effective approaches in dealing with few-shot image classification, as the low-level patterns and underlying representations can be easily transferred across different tasks in computer vision domain. However, directly applying this approach to few-shot text classification is challenging, since leveraging the statistics of known classes with sufficient samples to calibrate the distributions of novel classes may cause negative effects due to serious category difference in text domain. To alleviate this issue, we propose two simple yet effective strategies to estimate the distributions of the novel classes by utilizing unlabeled query samples, thus avoiding the potential negative transfer issue. Specifically, we first assume a class or sample follows the Gaussian distribution, and use the original support set and the nearest few query samples to estimate the corresponding mean and covariance. Then, we augment the labeled samples by sampling from the estimated distribution, which can provide sufficient supervision for training the classification model. Extensive experiments on eight few-shot text classification datasets show that the proposed method outperforms state-of-the-art baselines significantly.
更多查看译文
关键词
classification,distribution estimation,text,few-shot
AI 理解论文
溯源树
样例
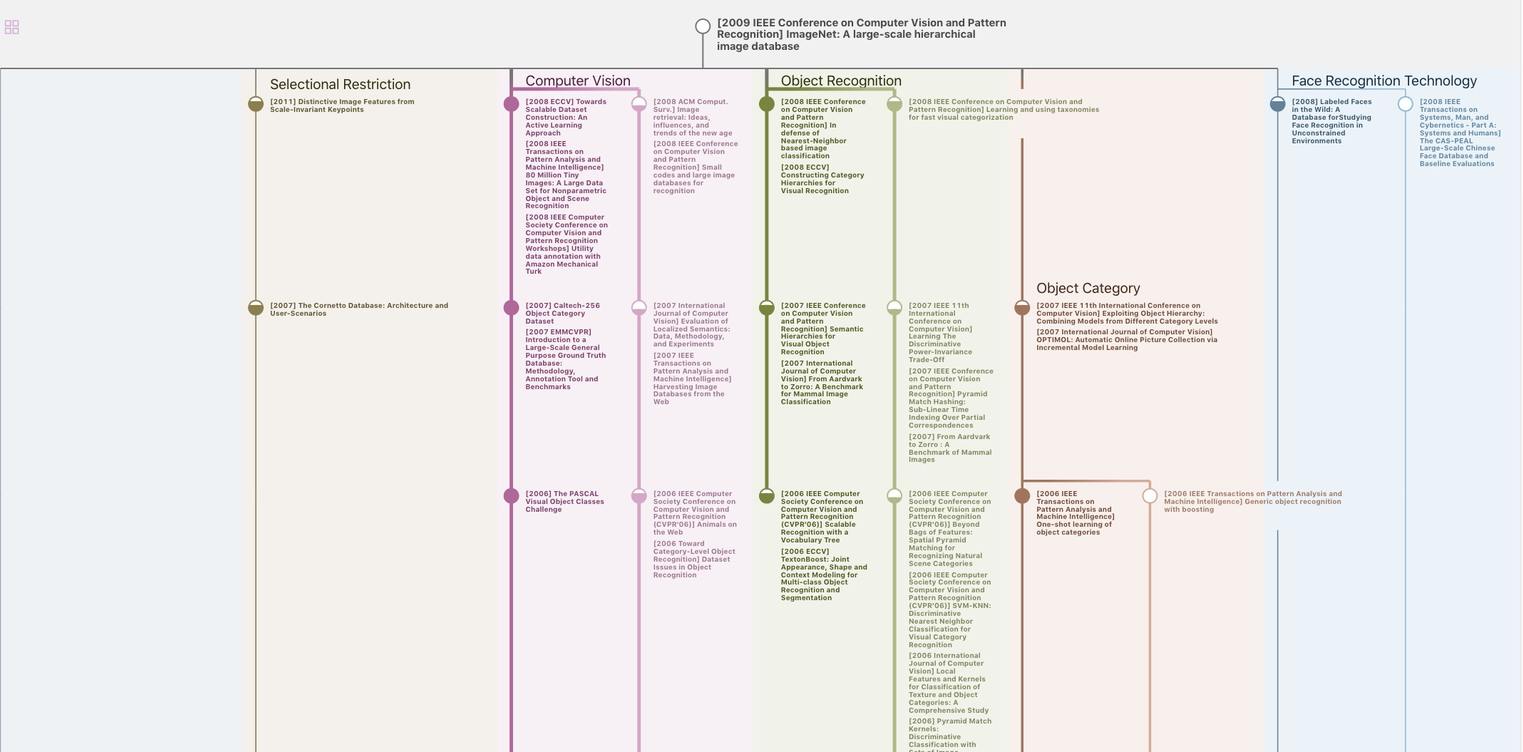
生成溯源树,研究论文发展脉络
Chat Paper
正在生成论文摘要