Physical Deep Reinforcement Learning Towards Safety Guarantee
CoRR(2023)
摘要
Deep reinforcement learning (DRL) has achieved tremendous success in many complex decision-making tasks of autonomous systems with high-dimensional state and/or action spaces. However, the safety and stability still remain major concerns that hinder the applications of DRL to safety-critical autonomous systems. To address the concerns, we proposed the Phy-DRL: a physical deep reinforcement learning framework. The Phy-DRL is novel in two architectural designs: i) Lyapunov-like reward, and ii) residual control (i.e., integration of physics-model-based control and data-driven control). The concurrent physical reward and residual control empower the Phy-DRL the (mathematically) provable safety and stability guarantees. Through experiments on the inverted pendulum, we show that the Phy-DRL features guaranteed safety and stability and enhanced robustness, while offering remarkably accelerated training and enlarged reward.
更多查看译文
关键词
deep reinforcement learning,safety guarantee,physical
AI 理解论文
溯源树
样例
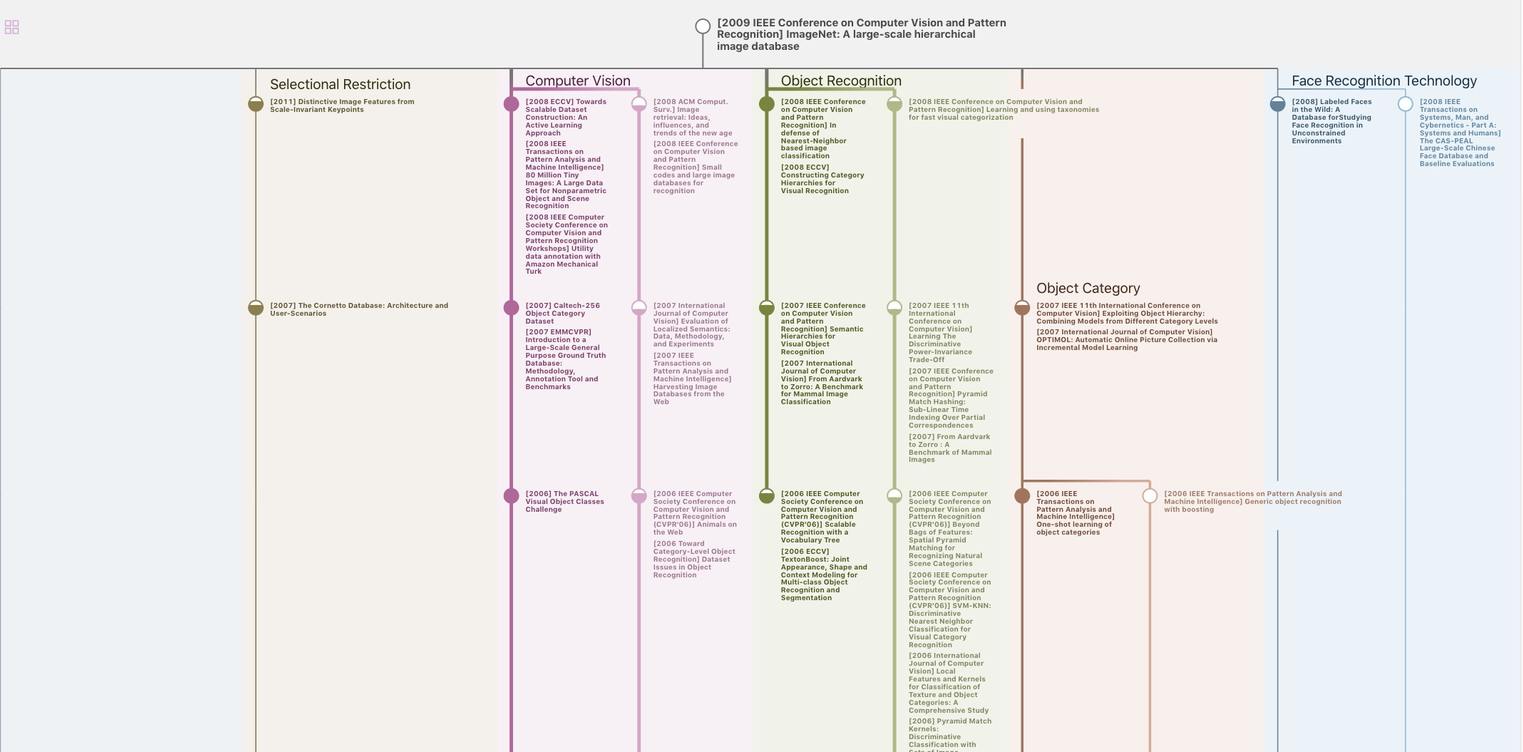
生成溯源树,研究论文发展脉络
Chat Paper
正在生成论文摘要