Sequence-aware Knowledge Distillation for a Lightweight Event Representation
ACM Transactions on Information Systems(2023)
摘要
Event representation targets to model the event-reasoning process as a machine-readable format. Previous studies on event representation mostly concentrate on a sole modeling perspective and have not well investigated the scenario-level knowledge, which can cause information loss. To cope with this dilemma, we propose a unified fine-tuning architecture-based approach (UniFA-S) that integrates all levels of trainings, including the scenario-level knowledge. However, another challenge for existing models is the ever-increasing computation overheads, restricting the deployment ability on limited resources devices. Hence, in this article, we aim to compress the cumbersome model UniFA-S into a lighter and easy-to-deploy one without much performance damage. To this end, we propose a sequence-aware knowledge distillation model (SaKD) that employs a dynamic self-distillation on the decouple-compress-couple framework for compressing UniFA-S, which cannot only realize the model compression, but also retain the integrity of individual components. We also design two fitting strategies to address the less-supervised issue at the distillation stage. Comprehensive experiments on representation-and-inference ability-based tasks validate the effectiveness of SaKD. Compared to UniFA-S, SaKD realizes a more portable event representation model at the cost of only 1.0% performance drop in terms of accuracy or Spearman's correlation, which is far less than other knowledge distillation models.
更多查看译文
关键词
Event representation,knowledge distillation,event similarity,event prediction
AI 理解论文
溯源树
样例
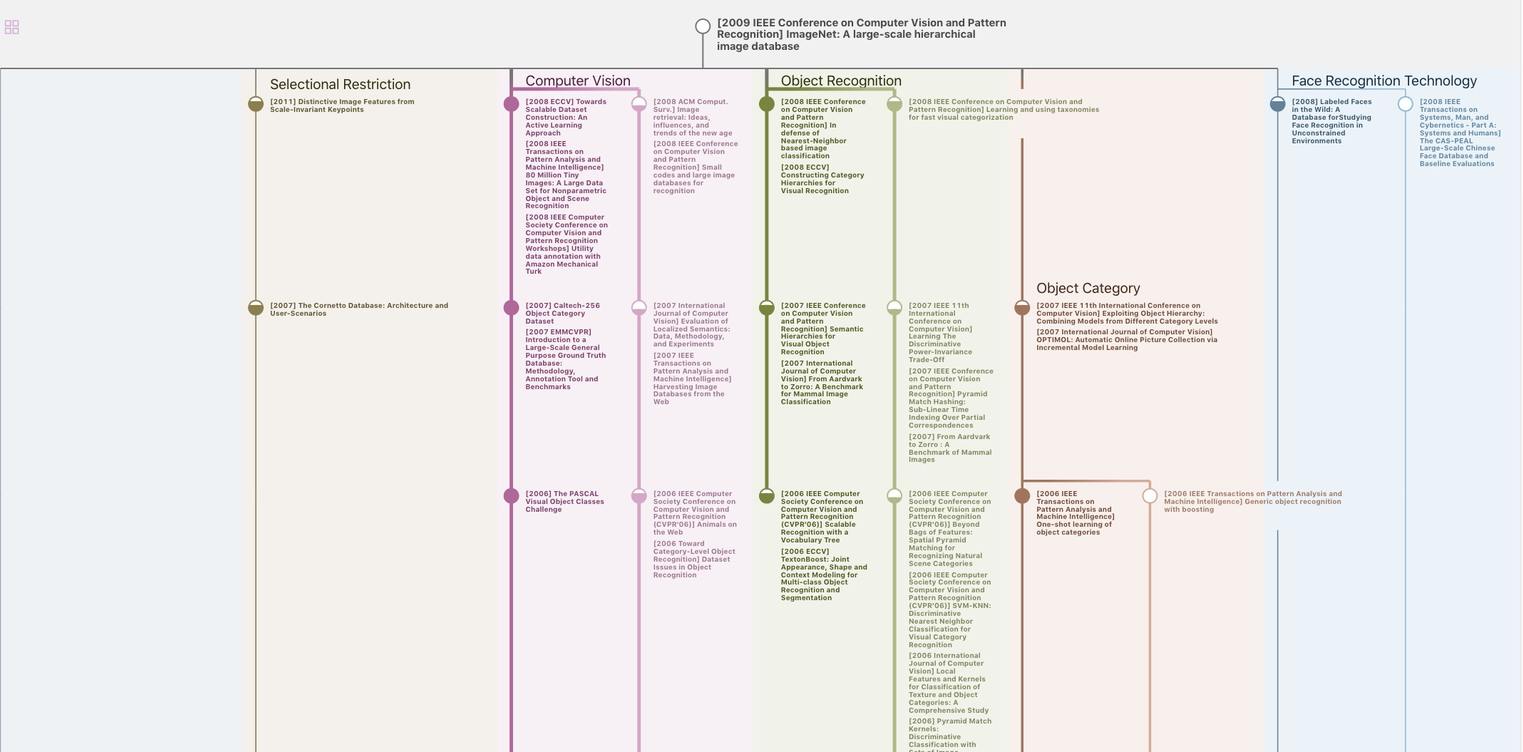
生成溯源树,研究论文发展脉络
Chat Paper
正在生成论文摘要