AROMA: Evaluating Deep Learning Systems for Stealthy Integrity Attacks on Multi-tenant Accelerators
ACM Journal on Emerging Technologies in Computing Systems(2023)
摘要
Multi-tenant applications have been proliferating in recent years, supported by the emergence of computingas-service paradigms. Unfortunately, multi-tenancy induces new security vulnerabilities due to spatial or temporal co-location of applications with possibly malicious intent. In this article, we consider a special class of stealthy integrity attacks on multi-tenant deep learning accelerators. One interesting conclusion is that it is possible to perform targeted integrity attacks on kernel weights of deep learning systems such that it remains functional but mis-labels specific categories of input data through standard RowHammer attacks by only changing 0.0009% of the total weights. We develop an automated framework, AROMA, to evaluate the impact of multi-tenancy on security of deep learning accelerators against integrity attacks on memory systems. We present extensive evaluations on AroMa to demonstrate its effectiveness.
更多查看译文
关键词
Integrity attack,neural networks,multi-tenant device,evaluation tool
AI 理解论文
溯源树
样例
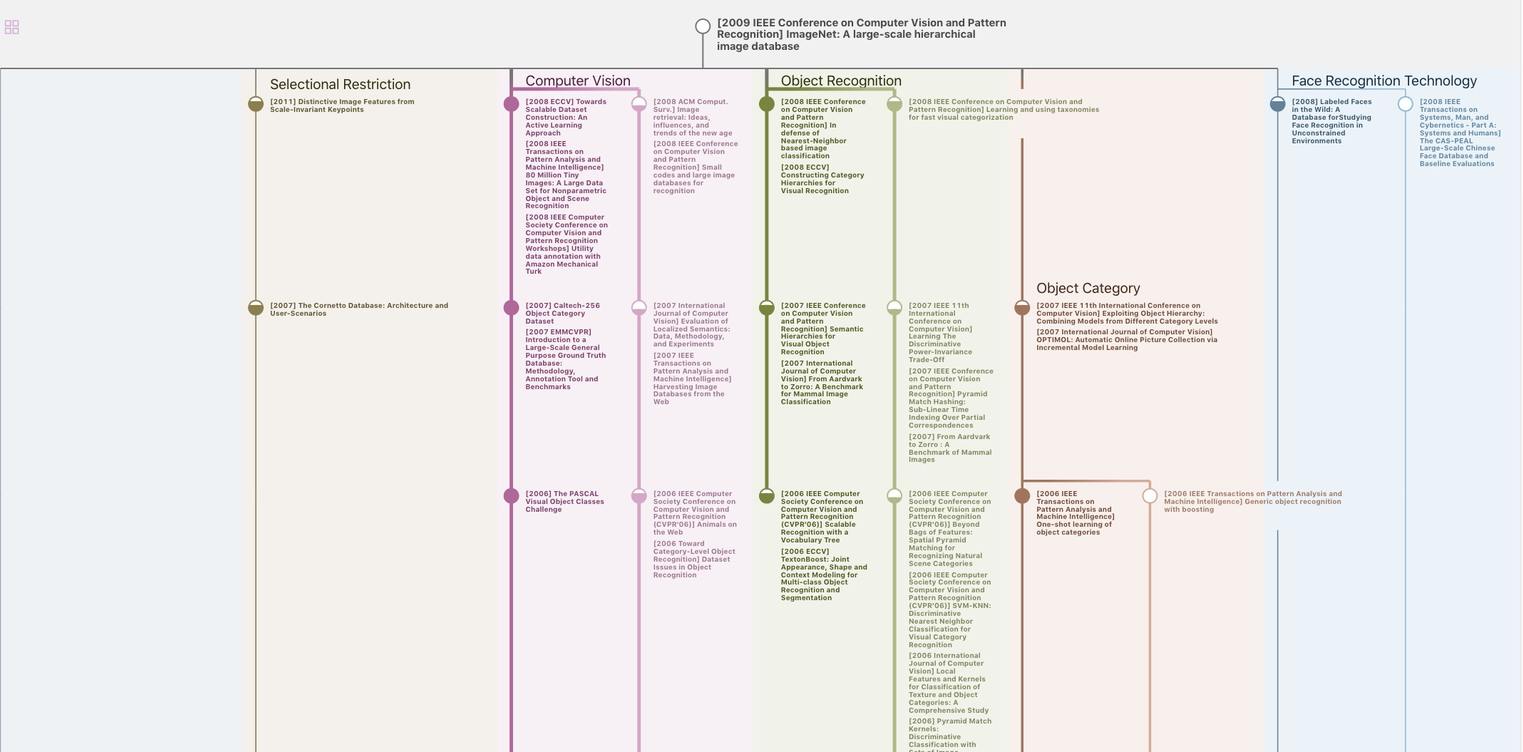
生成溯源树,研究论文发展脉络
Chat Paper
正在生成论文摘要