Understanding the Cognitive Influences of Interpretability Features on How Users Scrutinize Machine-Predicted Categories
CHIIR '23: Proceedings of the 2023 Conference on Human Information Interaction and Retrieval(2023)
摘要
The goal of interpretable machine learning (ML) is to design tools and visualizations to help users scrutinize a system’s predictions. Prior studies have mostly employed quantitative methods to investigate the effects of specific tools/visualizations on outcomes related to objective performance—a human’s ability to correctly agree or disagree with the system—and subjective perceptions of the system. Few studies have employed qualitative methods to investigate how and why specific tools/visualizations influence performance, perceptions, and behaviors. We report on a lab study (N = 30) that investigated the influences of two interpretability features: confidence values and sentence highlighting. Participants judged whether medical articles belong to a predicted medical topic and were exposed to two interface conditions—one with and one without interpretability features. We investigate the effects of our interpretability features on participants’ performance and perceptions. Additionally, we report on a qualitative analysis of participants’ responses during an exit interview. Specifically, we report on how our interpretability features impacted different cognitive activities that participants engaged with during the task—reading, learning, and decision making. We also describe ways in which the interpretability features introduced challenges and sometimes led participants to make mistakes. Insights gained from our results point to future directions for interpretable ML research.
更多查看译文
AI 理解论文
溯源树
样例
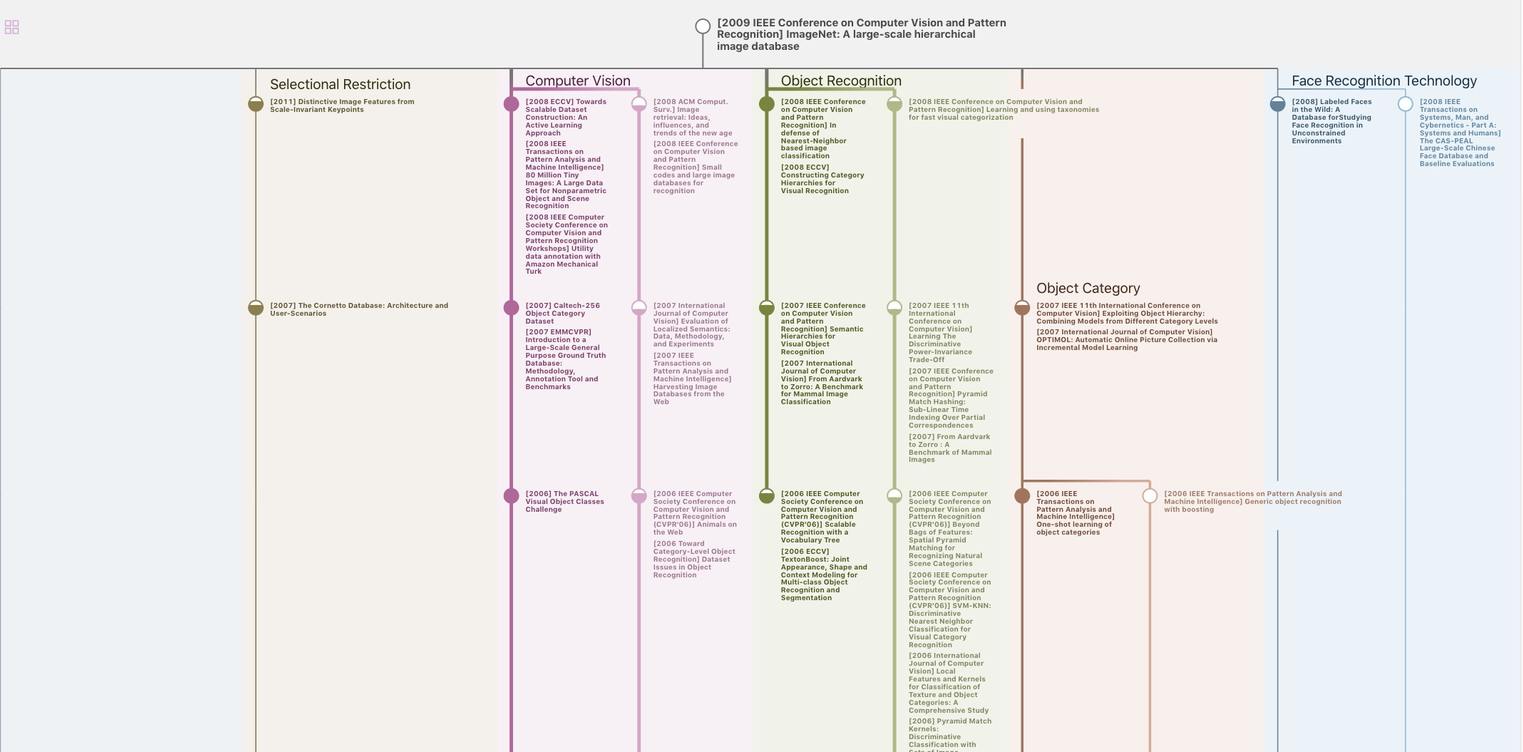
生成溯源树,研究论文发展脉络
Chat Paper
正在生成论文摘要