Clustering-based Active Learning Classification towards Data Stream
ACM Transactions on Intelligent Systems and Technology(2023)
摘要
Many practical applications, such as social media and monitoring system, will constantly generate streaming data, which has problems of instability, lack of labels and multiclass imbalance. In order to solve these problems, a cluster-based active learning method is proposed to achieve data stream classification. Firstly, a label query strategy combining marginal threshold matrix is proposed, which selects difficult to classify or potential concept drift samples for marking, to solve the problem of high cost label and unbalanced data. Secondly, dynamic maintenance of a group of micro clusters, by adjusting the weight of micro clusters in the model, correctly reflects the current data distribution, and finally, uses the buffer to store new micro clusters to participate in the update of the model, to adapt to the new data environment. Experimental results on three real data sets and three synthetic data sets show that compared with the classical data stream classification algorithm, it is less affected by concept drift and has higher classification accuracy than the online semi-supervised learning algorithm ADSM. The average accuracy of the six datasets increased by 5.56%, 2.32%, 1.77%, 1.83%, 3.78%, and 2.04%, respectively. The model processes data streams online and improves classification performance with less memory consumption.
更多查看译文
关键词
Active learning,data stream classification,concept drift,multi-class imbalance
AI 理解论文
溯源树
样例
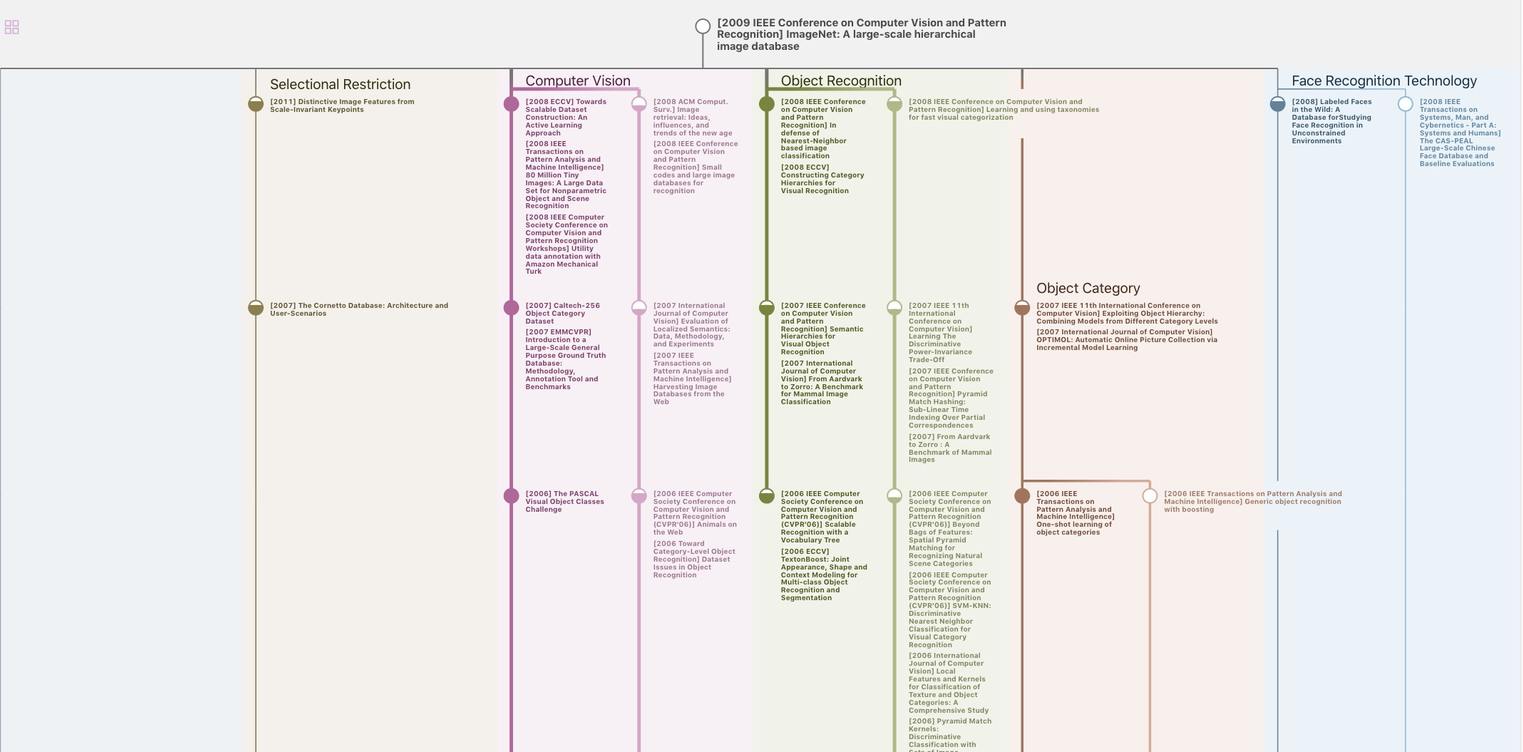
生成溯源树,研究论文发展脉络
Chat Paper
正在生成论文摘要