Hyper-node Relational Graph Attention Network for Multi-modal Knowledge Graph Completion
ACM Transactions on Multimedia Computing, Communications, and Applications(2023)
摘要
Knowledge graphs often suffer from incompleteness, and knowledge graph completion (KGC) aims at inferring the missing triplets through knowledge graph embedding from known factual triplets. However, most existing knowledge graph embedding methods only use the relational information of knowledge graph and treat the entities and relations as IDs with simple embedding layer, ignoring the multi-modal information among triplets, such as text descriptions, images, etc. In this work, we propose a novel network to incorporate different modal information with graph structure information for more precise representation of multi-modal knowledge graph, termed as hyper-node relational graph attention (HRGAT) network. In HRGAT, we use low-rank multi-modal fusion to model the intra-modality and inter-modality dynamics, which transforms the original knowledge graph to a hyper-node graph. Then, relational graph attention (RGAT) network is used, which contains relation-specific attention and entity-relation fusion operation to capture the graph structure information. Finally, we aggregate the updated multi-modal information and graph structure information to generate the final embeddings of knowledge graph to achieve KGC. By exploring multi-modal information and graph structure information, HRGAT embraces faster convergence speed and achieves the state-of-the-art for KGC on the standard datasets. Implementation code is available at https://github.com/broliang/HRGAT.
更多查看译文
关键词
Multi-modal knowledge graph,knowledge graph completion,relational graph attention network,low-rank multi-modal fusion
AI 理解论文
溯源树
样例
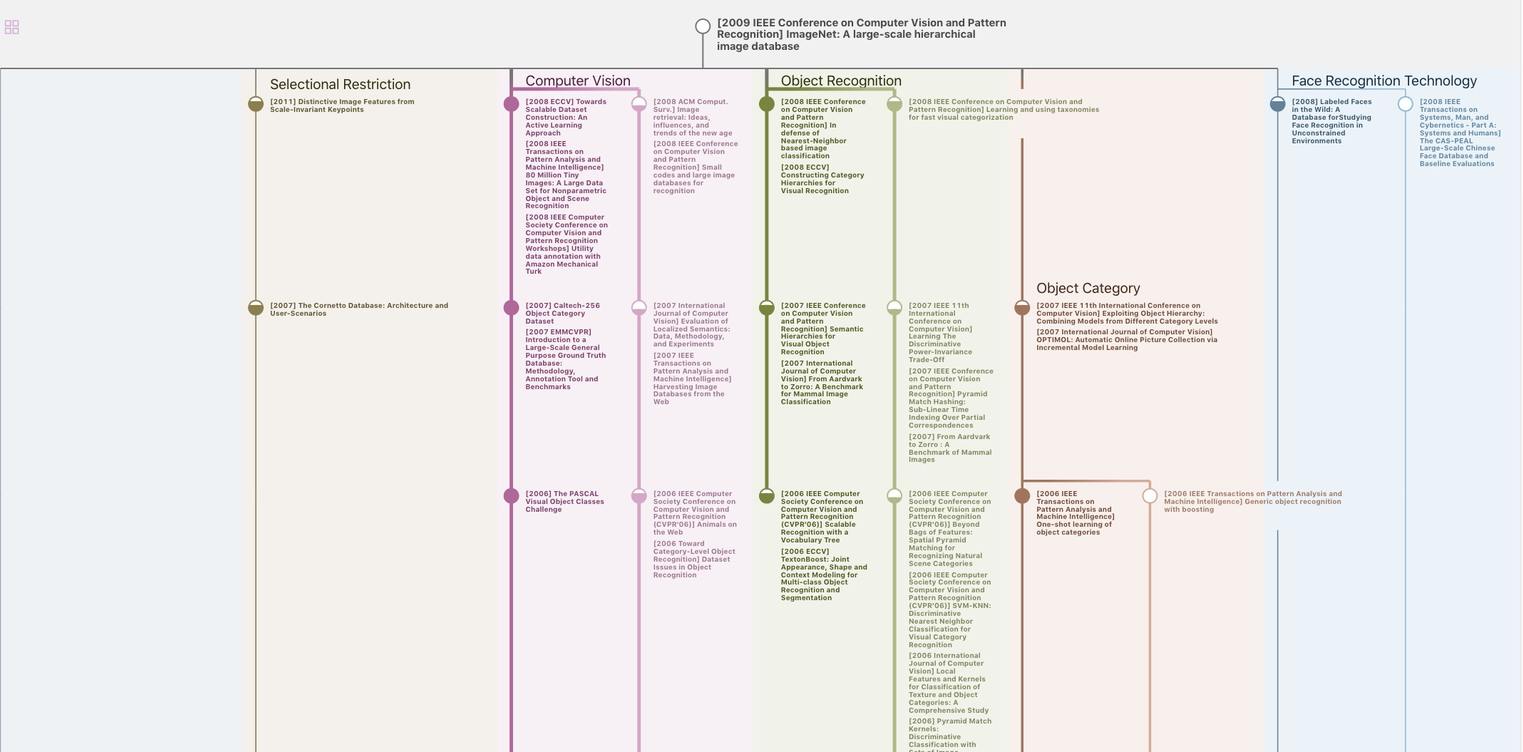
生成溯源树,研究论文发展脉络
Chat Paper
正在生成论文摘要