Frequency-aware Camouflaged Object Detection
ACM Transactions on Multimedia Computing, Communications, and Applications(2023)
摘要
Camouflaged object detection (COD) is important as it has various potential applications. Unlike salient object detection (SOD), which tries to identify visually salient objects, COD tries to detect objects that are visually very similar to the surrounding background. We observe that recent COD methods try to fuse features from different levels using some context aggregation strategies originally developed for SOD. Such an approach, however, may not be appropriate for COD as these existing context aggregation strategies are good at detecting distinctive objects while weakening the features from less discriminative objects. To address this problem, we propose in this article to exploit frequency learning to suppress the confusing high-frequency texture information, to help separate camouflaged objects from their surrounding background, and a frequency-based method, called FBNet, for camouflaged object detection. Specifically, we design a frequency-aware context aggregation (FACA) module to suppress high-frequency information and aggregate multi-scale features from a frequency perspective, an adaptive frequency attention (AFA) module to enhance the features of the learned important frequency components, and a gradient-weighted loss function to guide the proposed method to pay more attention to contour details. Experimental results show that our model outperforms relevant state-of-the-art methods.
更多查看译文
关键词
Camouflaged object detection,frequency learning
AI 理解论文
溯源树
样例
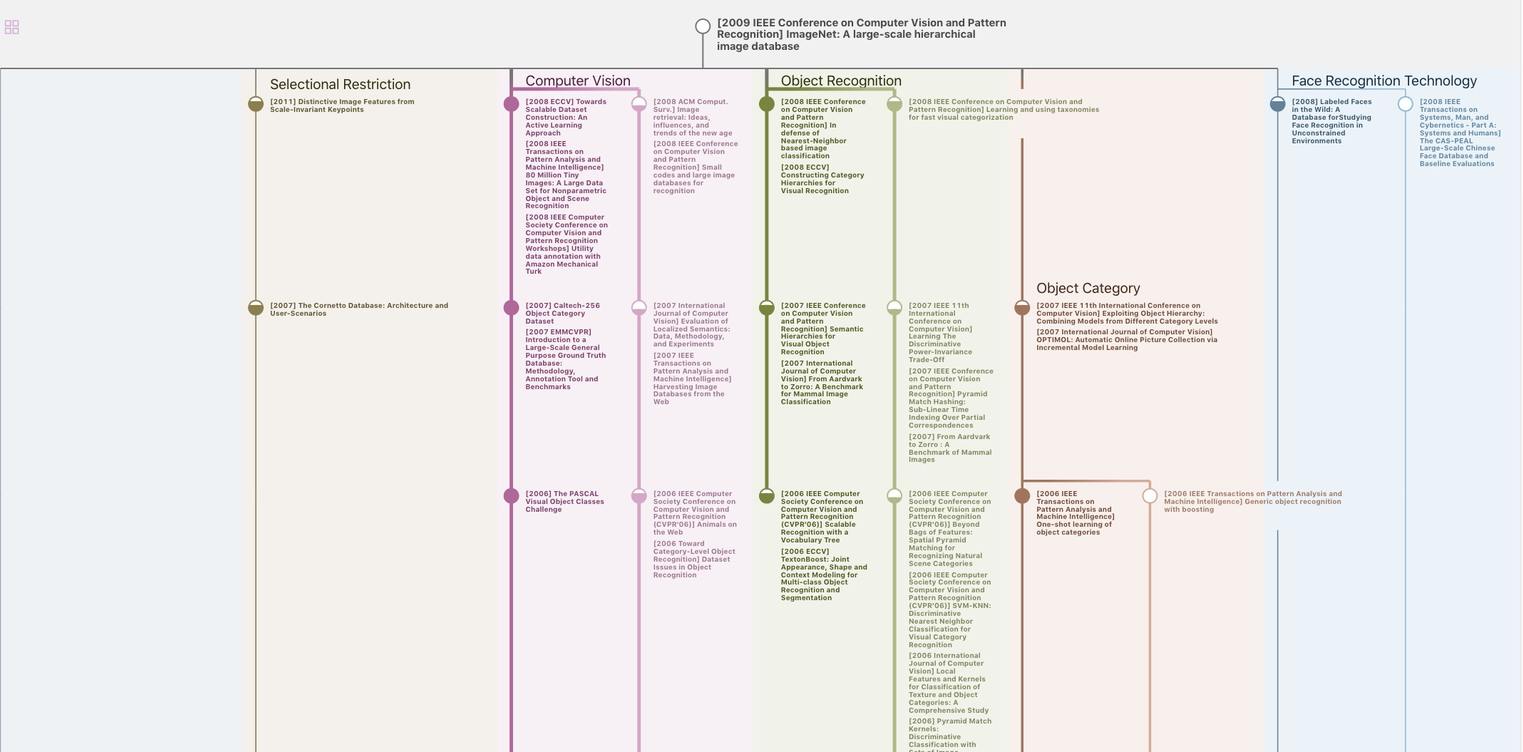
生成溯源树,研究论文发展脉络
Chat Paper
正在生成论文摘要