Federated End-to-End Unrolled Models for Magnetic Resonance Image Reconstruction
Bioengineering(2023)
摘要
Image reconstruction is the process of recovering an image from raw, under-sampled signal measurements, and is a critical step in diagnostic medical imaging, such as magnetic resonance imaging (MRI). Recently, data-driven methods have led to improved image quality in MRI reconstruction using a limited number of measurements, but these methods typically rely on the existence of a large, centralized database of fully sampled scans for training. In this work, we investigate federated learning for MRI reconstruction using end-to-end unrolled deep learning models as a means of training global models across multiple clients (data sites), while keeping individual scans local. We empirically identify a low-data regime across a large number of heterogeneous scans, where a small number of training samples per client are available and non-collaborative models lead to performance drops. In this regime, we investigate the performance of adaptive federated optimization algorithms as a function of client data distribution and communication budget. Experimental results show that adaptive optimization algorithms are well suited for the federated learning of unrolled models, even in a limited-data regime (50 slices per data site), and that client-sided personalization can improve reconstruction quality for clients that did not participate in training.
更多查看译文
关键词
MRI reconstruction,federated learning,unrolled optimization,accelerated MRI
AI 理解论文
溯源树
样例
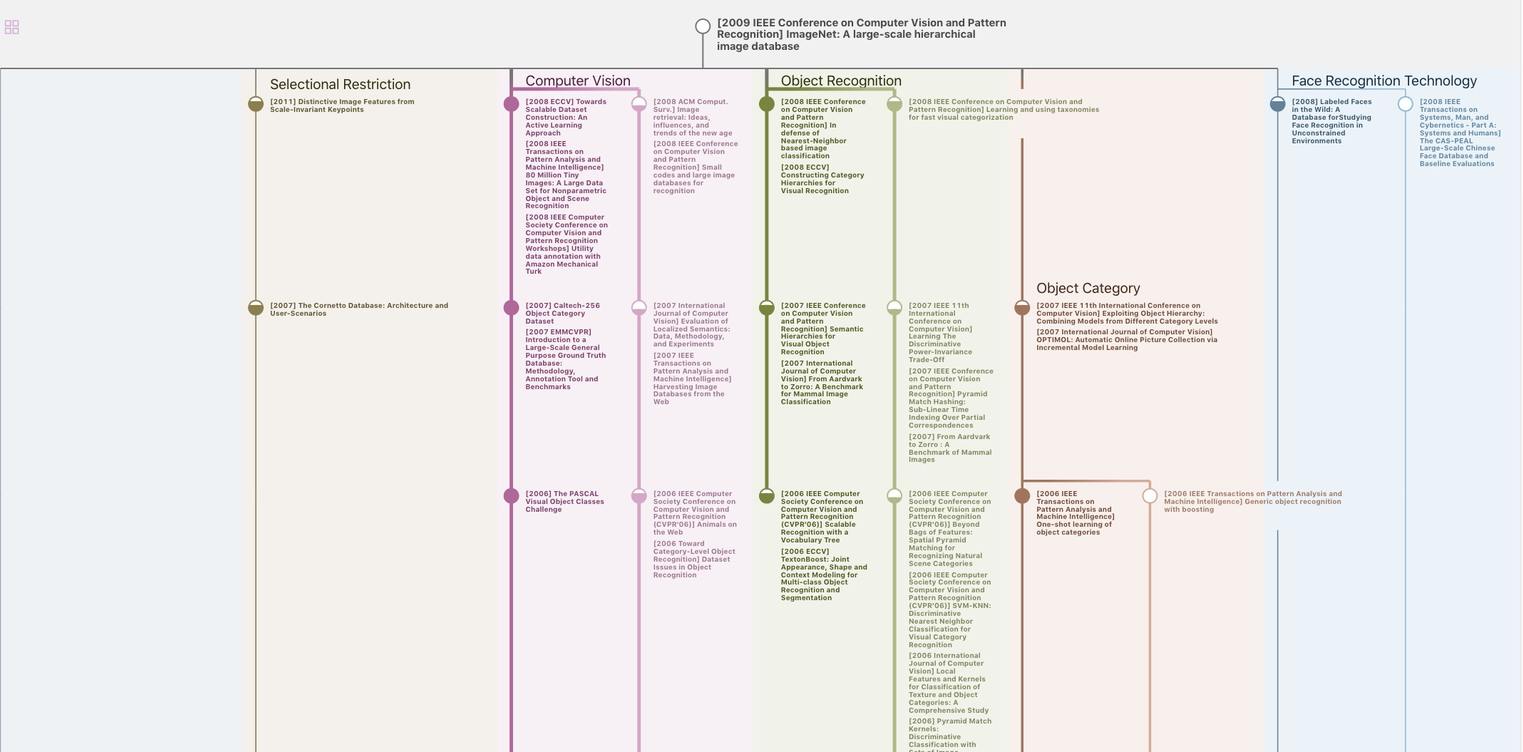
生成溯源树,研究论文发展脉络
Chat Paper
正在生成论文摘要