Impact Ionization Coefficient Prediction of a Lateral Power Device Using Deep Neural Network.
Micromachines(2023)
摘要
Nowadays, the impact ionization coefficient in the avalanche breakdown theory is obtained using 1-D PN junctions or SBDs, and is considered to be a constant determined by the material itself only. In this paper, the impact ionization coefficient of silicon in a 2D lateral power device is found to be no longer a constant, but instead a function of the 2D coupling effects. The impact ionization coefficient of silicon that considers the 2D depletion effects in real-world devices is proposed and extracted in this paper. The extracted impact ionization coefficient indicates that the conventional empirical impact ionization in the Fulop equation is not suitable for the analysis of 2D lateral power devices. The veracity of the proposed impact ionization coefficient is validated by the simulations obtained from TCAD tools. Considering the complexity of direct modeling, a new prediction method using deep neural networks is proposed. The prediction method demonstrates 97.67% accuracy for breakdown location prediction and less than 6% average error for the impact ionization coefficient prediction compared with the TCAD simulation.
更多查看译文
关键词
avalanche breakdown,deep neural networks (DNN),impact ionization coefficient,lateral power device
AI 理解论文
溯源树
样例
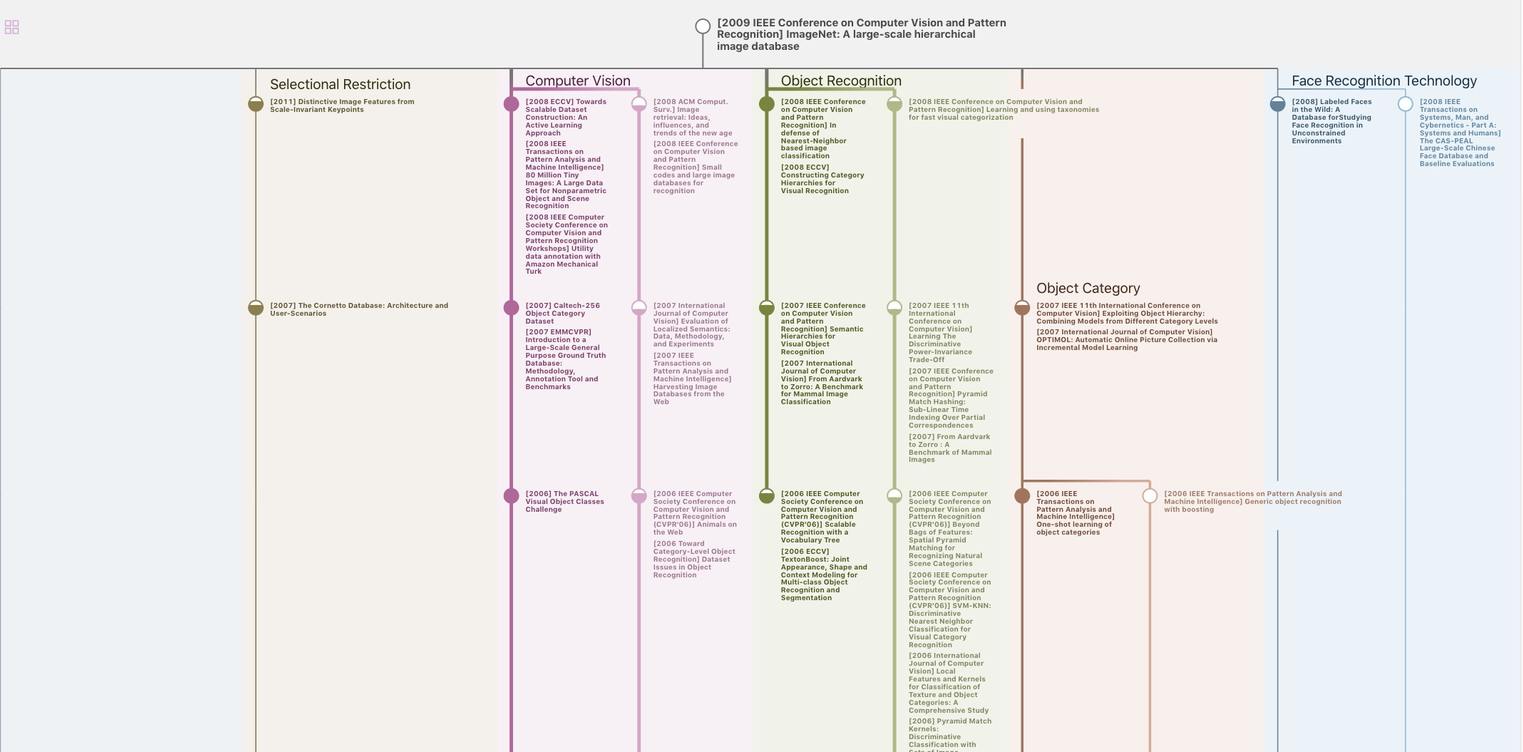
生成溯源树,研究论文发展脉络
Chat Paper
正在生成论文摘要