MEQA: Manifold embedding quality assessment via anisotropic scaling and Kolmogorov-Smirnov test
Pattern Recognition(2023)
摘要
Manifold learning methods unfold the manifold structures and embed them in a lower-dimensional space. The quality of such an embedding should be measured both qualitatively and quantitatively. The pro-posed manifold embedding quality assessment (MEQA) method does so by taking into account of local and global structure preservation as both are important traits of an embedding. To measure the local structure preservation MEQA uses two transformations. Initially anisotropic scaling, rotation and trans-lation are incorporated to measure the closeness between the original and the embedded data points. In the next stage, rigid transformation is incorporated to quantify the previous transformation which in -volved anisotropic scaling. For quantifying the global structure preservation, the Kolmogorov-Smirnov test is applied in a distributed manner over each dimension and averaged over them. To establish the superi-ority of MEQA we conducted several studies over standard synthetic and real-life datasets across separate existing feature extraction techniques.(c) 2023 Published by Elsevier Ltd.
更多查看译文
关键词
Manifold learning,Anisotropic scaling,Gradient descent,Global scaling,Singular value decomposition,Kolmogorov-Smirnov test
AI 理解论文
溯源树
样例
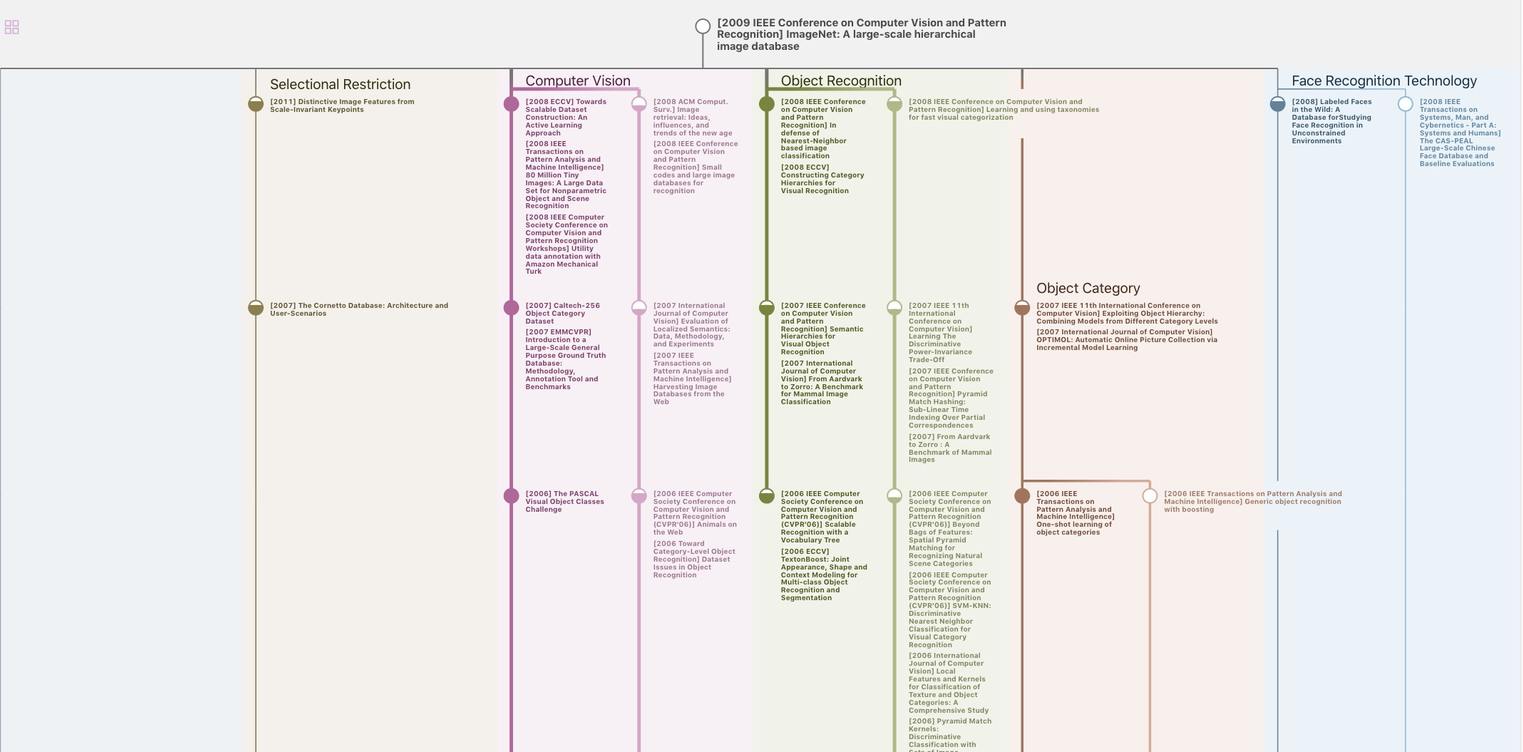
生成溯源树,研究论文发展脉络
Chat Paper
正在生成论文摘要