Learning transcriptional and regulatory dynamics driving cancer cell plasticity using neural ODE-based optimal transport
biorxiv(2023)
摘要
While single-cell technologies have allowed scientists to characterize cell states that emerge during cancer progression through temporal sampling, connecting these samples over time and inferring gene-gene relationships that promote cancer plasticity remains a challenge. To address these challenges, we developed TrajectoryNet, a neural ordinary differential equation network that learns continuous dynamics via interpolation of population flows between sampled timepoints. By running causality analysis on the output of TrajectoryNet, we compute rich and complex gene-gene networks that drive pathogenic trajectories forward. Applying this pipeline to scRNAseq data generated from in vitro models of breast cancer, we identify and validate a refined CD44 hi EPCAM+CAV1 + marker profile that improves the identification and isolation of cancer stem cells (CSCs) from bulk cell populations. Studying the cell plasticity trajectories emerging from this population, we identify comprehensive temporal regulatory networks that drive cell fate decisions between an epithelial-to-mesenchymal (EMT) trajectory, and a mesenchymal-to-epithelial (MET) trajectory. Through these studies, we identify and validate estrogen related receptor alpha as a critical mediator of CSC plasticity. We further apply TrajectoryNet to an in vivo xenograft model and demonstrate it’s ability to elucidate trajectories governing primary tumor metastasis to the lung, identifying a dominant EMT trajectory that includes elements of our newly-defined temporal EMT regulatory network. Demonstrated here in cancer, the TrajectoryNet pipeline is a transformative approach to uncovering temporal molecular programs operating in dynamic cell systems from static single-cell data.
### Competing Interest Statement
The authors have declared no competing interest.
更多查看译文
AI 理解论文
溯源树
样例
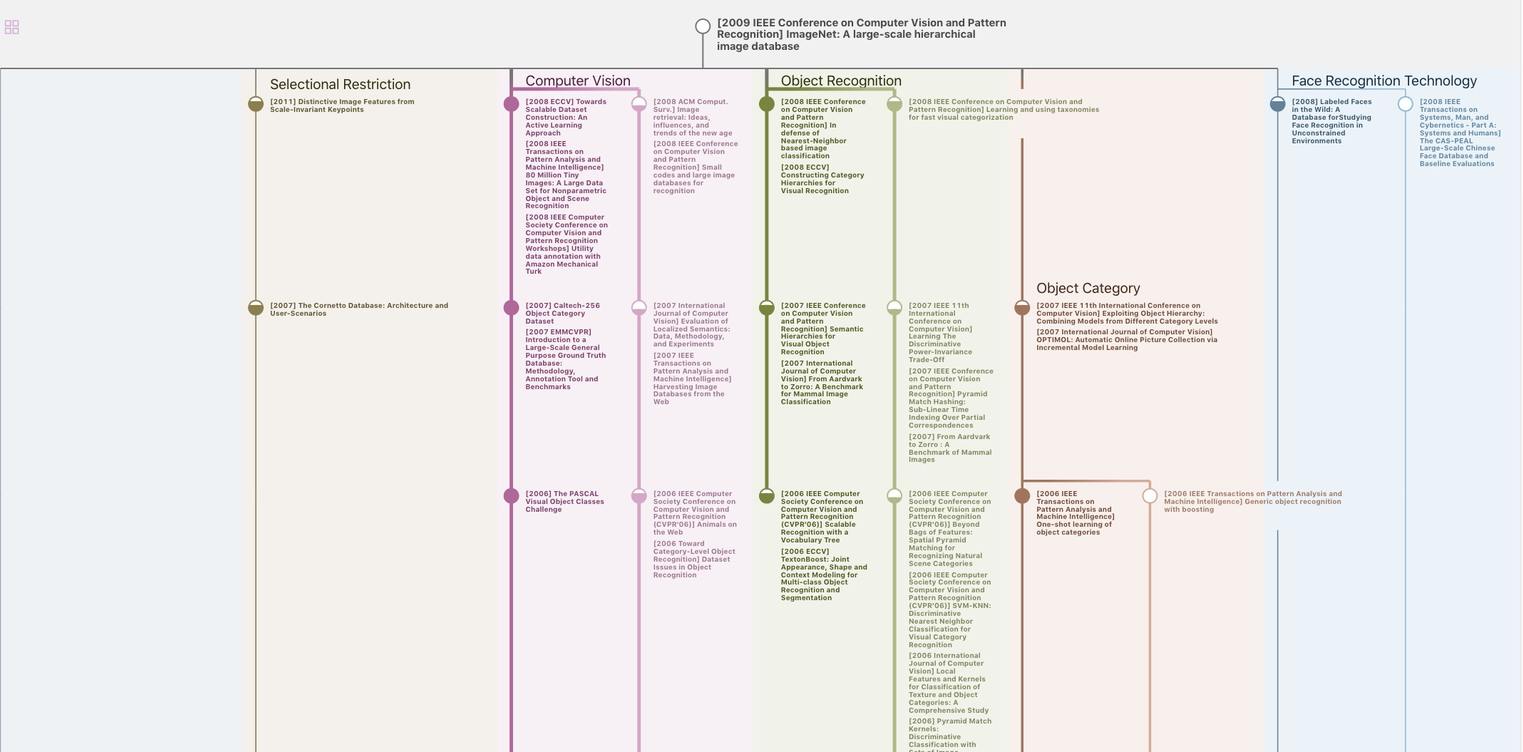
生成溯源树,研究论文发展脉络
Chat Paper
正在生成论文摘要