Rapid detection of serological biomarkers in gallbladder carcinoma using fourier transform infrared spectroscopy combined with machine learning.
Talanta(2023)
摘要
Gallbladder cancer (GBC) is the most common malignant tumour of the biliary tract. GBC is difficult to diagnose and treat at an early stage because of the lack of effective serum markers and typical symptoms, resulting in low survival rates. This study aimed to investigate the applicability of dried serum Fourier-transform infrared (FTIR) spectroscopy combined with machine learning algorithms to correctly differentiate patients with GBC from patients with gallbladder disease (GBD), cholangiocarcinoma (CCA), hepatocellular carcinoma (HCC) and healthy individuals. The differentiation between healthy individuals and GBC serum was better using principal component analysis (PCA) and linear discriminant analysis (LDA) for six spectral regions, especially in the protein (1710-1475 cm) and combined (1710-1475 + 1354-980 cm) region. However, the PCA-LDA model poorly differentiated GBC from GBD, CCA, and HCC in serum spectra. We evaluated the PCA- LDA, PCA-support vector machine (SVM), and radial basis kernel function support vector machine (RBF-SVM) models for GBC diagnosis and found that the RBF-SVM model performed the best, with 88.24-95% accuracy, 95.83% sensitivity, and 78.38-94.44% specificity in the 1710-1475 + 1354-980 cm region. This study demonstrated that serum FTIR spectroscopy combined with the RBF-SVM algorithm has great clinical potential for GBC screening.
更多查看译文
关键词
Cholangiocarcinoma,Fourier transform infrared spectroscopy,Gallbladder carcinoma,Hepatocellular carcinoma,Machine learning,Serum
AI 理解论文
溯源树
样例
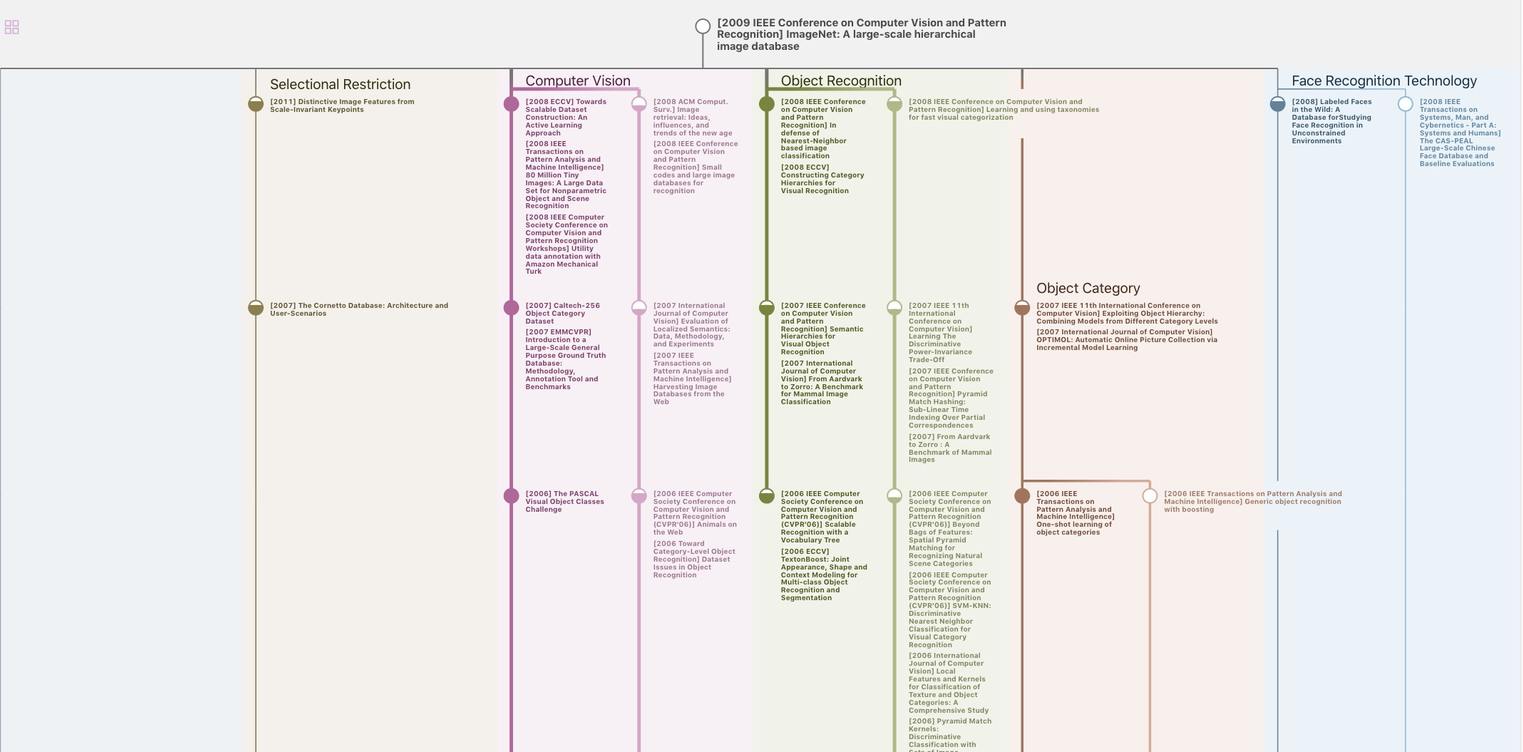
生成溯源树,研究论文发展脉络
Chat Paper
正在生成论文摘要