Domain Word Extension Using Curriculum Learning.
Sensors (Basel, Switzerland)(2023)
摘要
Self-supervised learning models, such as BERT, have improved the performance of various tasks in natural language processing. Although the effect is reduced in the out-of-domain field and not the the trained domain thus representing a limitation, it is difficult to train a new language model for a specific domain since it is both time-consuming and requires large amounts of data. We propose a method to quickly and effectively apply the pre-trained language models trained in the general domain to a specific domain's vocabulary without re-training. An extended vocabulary list is obtained by extracting a meaningful wordpiece from the training data of the downstream task. We introduce curriculum learning, training the models with two successive updates, to adapt the embedding value of the new vocabulary. It is convenient to apply because all training of the models for downstream tasks are performed in one run. To confirm the effectiveness of the proposed method, we conducted experiments on AIDA-SC, AIDA-FC, and KLUE-TC, which are Korean classification tasks, and subsequently achieved stable performance improvement.
更多查看译文
关键词
curriculum learning,pre-trained models,token expansion
AI 理解论文
溯源树
样例
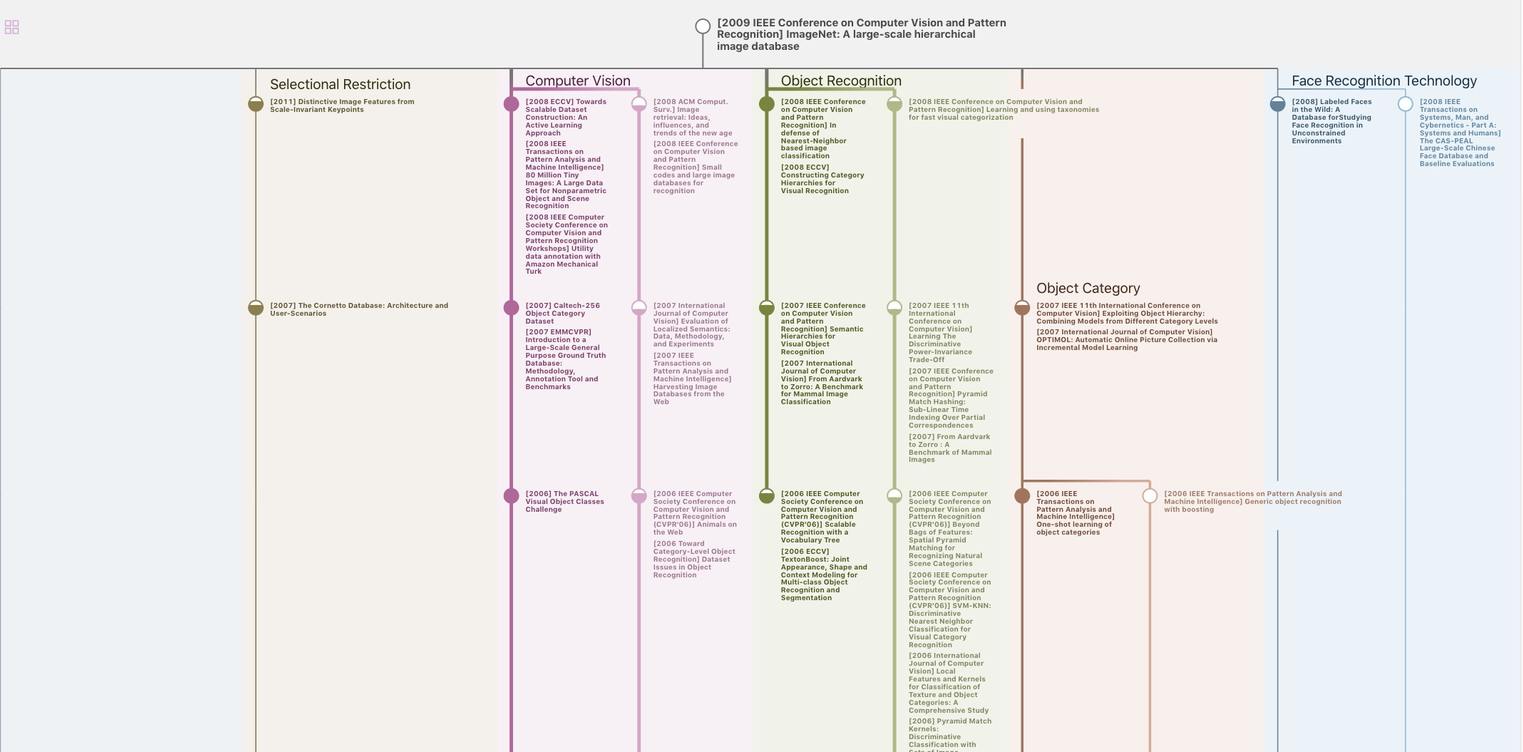
生成溯源树,研究论文发展脉络
Chat Paper
正在生成论文摘要