Toward reliable calcification detection: calibration of uncertainty in object detection from coronary optical coherence tomography images.
Journal of biomedical optics(2023)
摘要
Significance:Optical coherence tomography (OCT) has become increasingly essential in assisting the treatment of coronary artery disease (CAD). However, unidentified calcified regions within a narrowed artery could impair the outcome of the treatment. Fast and objective identification is paramount to automatically procuring accurate readings on calcifications within the artery.
Aim:We aim to rapidly identify calcification in coronary OCT images using a bounding box and reduce the prediction bias in automated prediction models.
Approach:We first adopt a deep learning-based object detection model to rapidly draw the calcified region from coronary OCT images using a bounding box. We measure the uncertainty of predictions based on the expected calibration errors, thus assessing the certainty level of detection results. To calibrate confidence scores of predictions, we implement dependent logistic calibration using each detection result's confidence and center coordinates.
Results:We implemented an object detection module to draw the boundary of the calcified region at a rate of 140 frames per second. With the calibrated confidence score of each prediction, we lower the uncertainty of predictions in calcification detection and eliminate the estimation bias from various object detection methods. The calibrated confidence of prediction results in a confidence error of ∼ 0.13 , suggesting that the confidence calibration on calcification detection could provide a more trustworthy result.
Conclusions:Given the rapid detection and effective calibration of the proposed work, we expect that it can assist in clinical evaluation of treating the CAD during the imaging-guided procedure.
更多查看译文
关键词
calibration,coronary artery disease,deep learning,optical coherence tomography
AI 理解论文
溯源树
样例
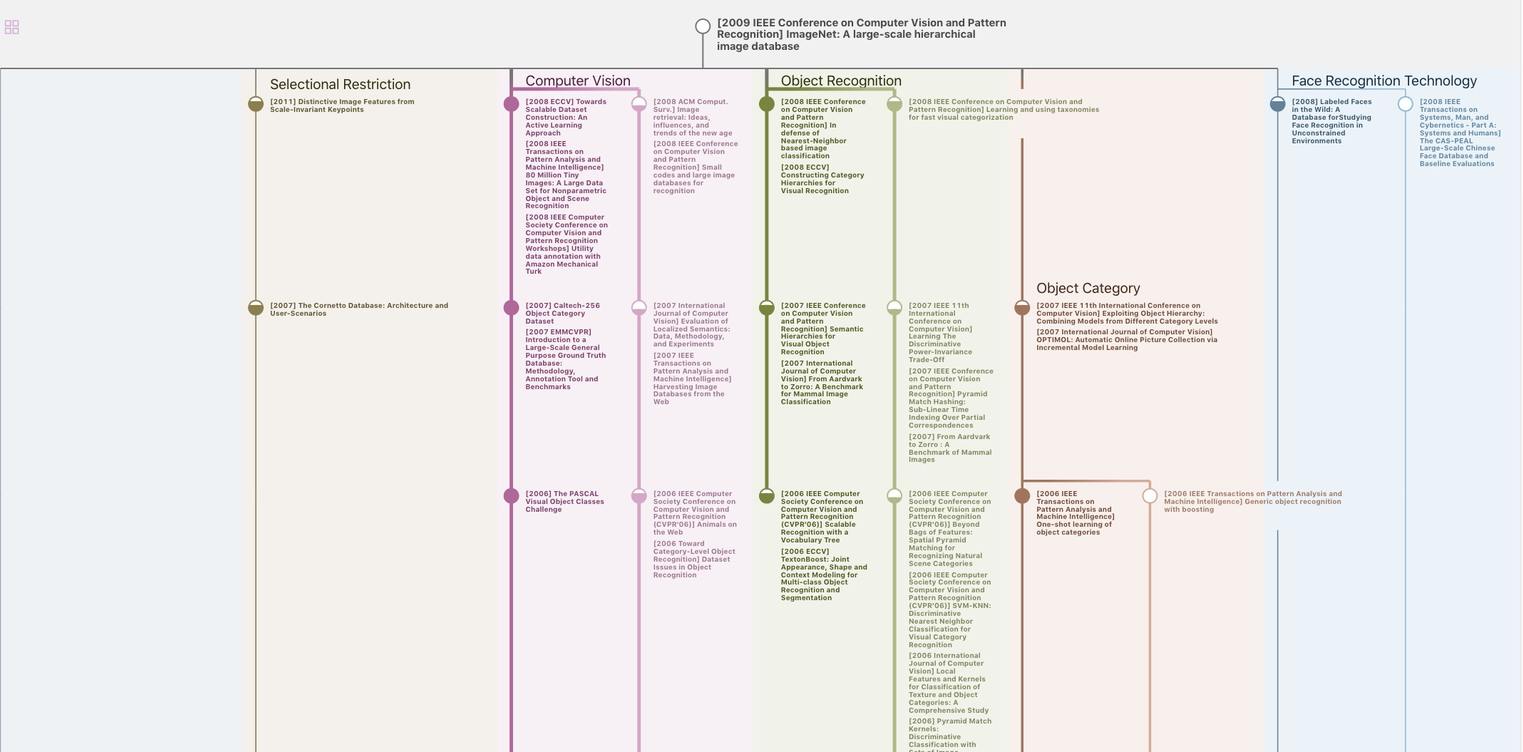
生成溯源树,研究论文发展脉络
Chat Paper
正在生成论文摘要