Target-driven machine learning-enabled virtual screening (TAME-VS) platform for early-stage hit identification.
Frontiers in molecular biosciences(2023)
摘要
High-throughput screening (HTS) methods enable the empirical evaluation of a large scale of compounds and can be augmented by virtual screening (VS) techniques to save time and money by using potential active compounds for experimental testing. Structure-based and ligand-based virtual screening approaches have been extensively studied and applied in drug discovery practice with proven outcomes in advancing candidate molecules. However, the experimental data required for VS are expensive, and hit identification in an effective and efficient manner is particularly challenging during early-stage drug discovery for novel protein targets. Herein, we present our TArget-driven Machine learning-Enabled VS (TAME-VS) platform, which leverages existing chemical databases of bioactive molecules to modularly facilitate hit finding. Our methodology enables bespoke hit identification campaigns through a user-defined protein target. The input target ID is used to perform a homology-based target expansion, followed by compound retrieval from a large compilation of molecules with experimentally validated activity. Compounds are subsequently vectorized and adopted for machine learning (ML) model training. These machine learning models are deployed to perform model-based inferential virtual screening, and compounds are nominated based on predicted activity. Our platform was retrospectively validated across ten diverse protein targets and demonstrated clear predictive power. The implemented methodology provides a flexible and efficient approach that is accessible to a wide range of users. The TAME-VS platform is publicly available at https://github.com/bymgood/Target-driven-ML-enabled-VS to facilitate early-stage hit identification.
更多查看译文
关键词
AIDD,drug discovery,hit identification,machine learning,virtual screening
AI 理解论文
溯源树
样例
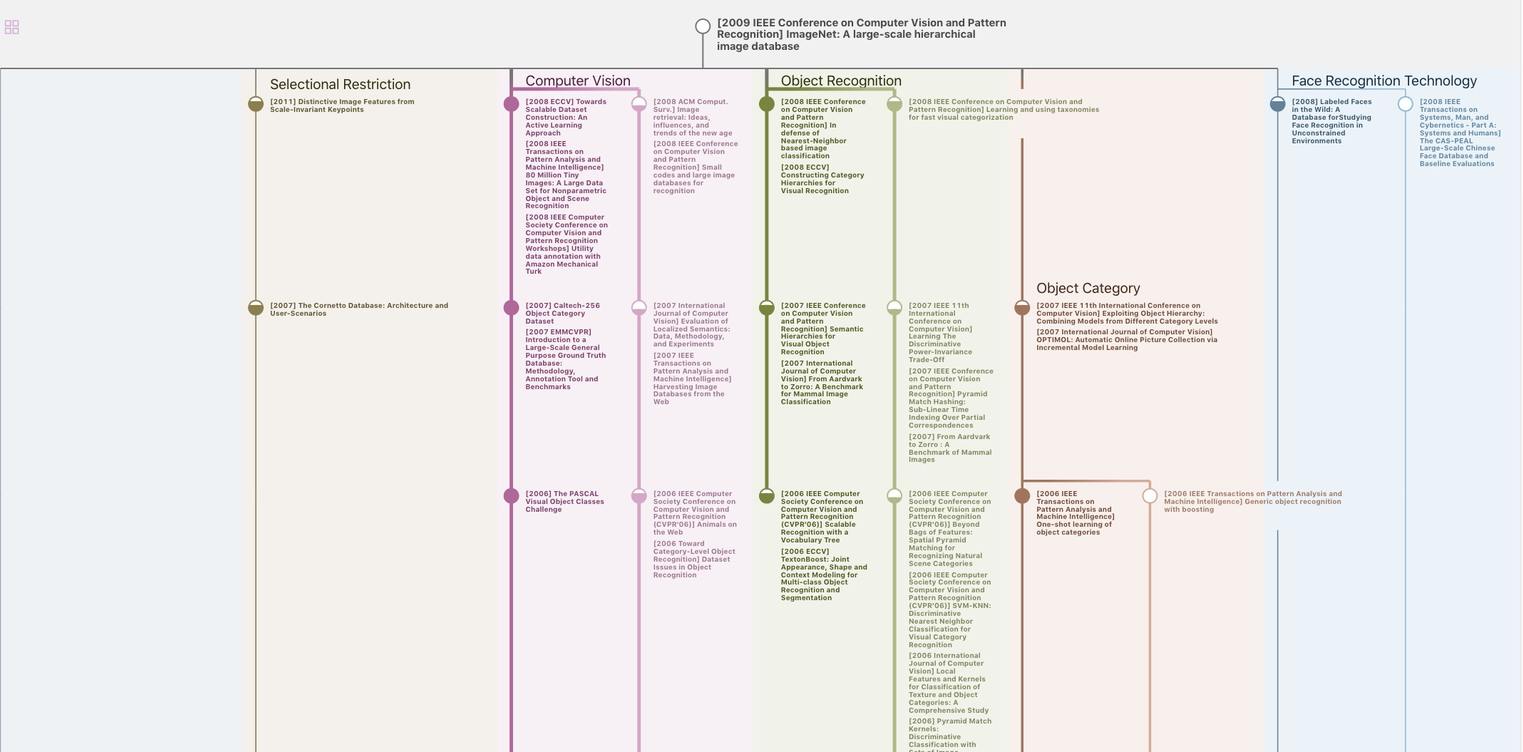
生成溯源树,研究论文发展脉络
Chat Paper
正在生成论文摘要