Exploring the Relationship of Microstructure and Conductivity in Metal Halide Perovskites via Active Learning-Driven Automated Scanning Probe Microscopy.
The journal of physical chemistry letters(2023)
摘要
Electronic transport and hysteresis in metal halide perovskites (MHPs) are key to the applications in photovoltaics, light emitting devices, and light and chemical sensors. These phenomena are strongly affected by the materials microstructure including grain boundaries, ferroic domain walls, and secondary phase inclusions. Here, we demonstrate an active machine learning framework for "driving" an automated scanning probe microscope (SPM) to discover the microstructures responsible for specific aspects of transport behavior in MHPs. In our setup, the microscope can discover the microstructural elements that maximize the onset of conduction, hysteresis, or any other characteristic that can be derived from a set of current-voltage spectra. This approach opens new opportunities for exploring the origins of materials functionality in complex materials by SPM and can be integrated with other characterization techniques either before (prior knowledge) or after (identification of locations of interest for detail studies) functional probing.
更多查看译文
关键词
metal halide perovskites,conductivity,microstructure,scanning,learning-driven
AI 理解论文
溯源树
样例
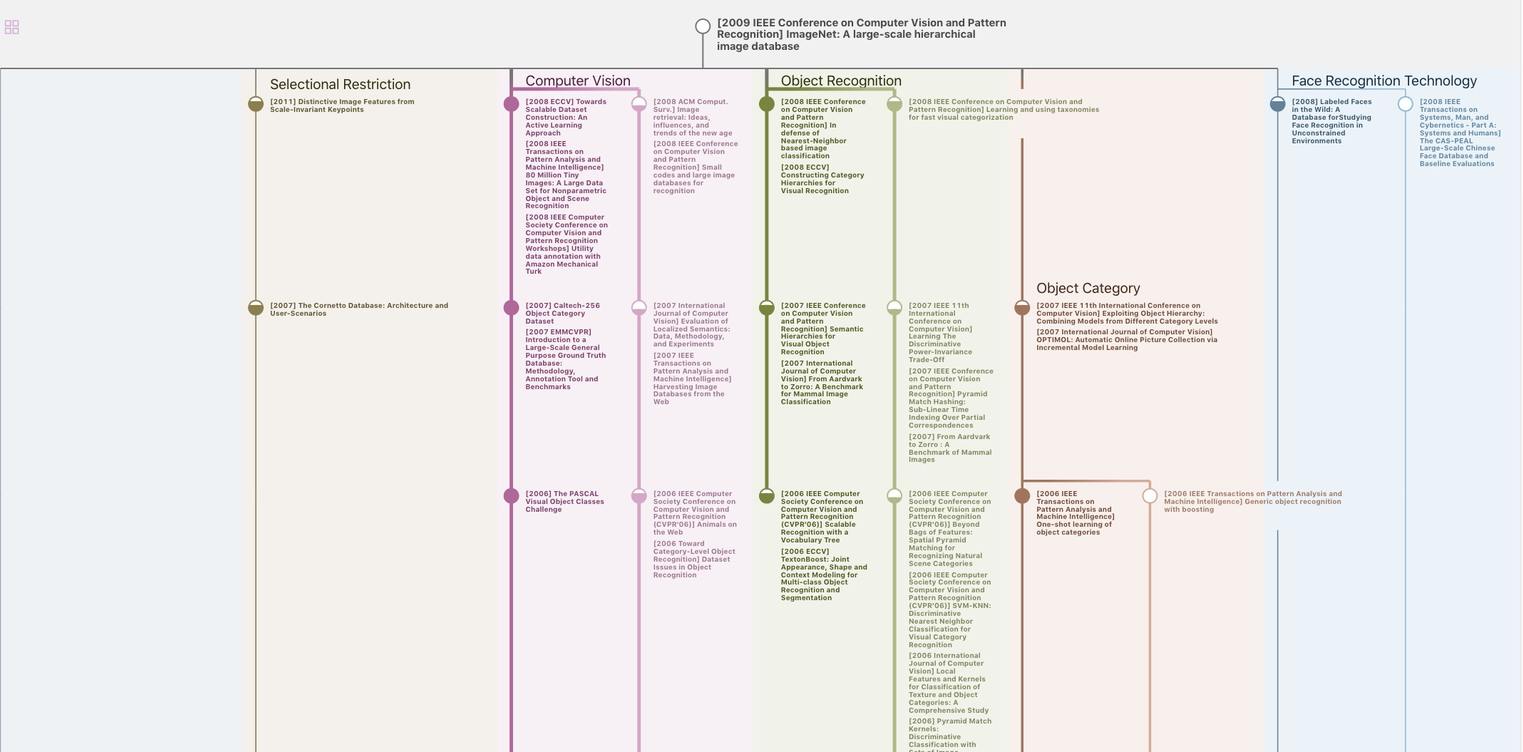
生成溯源树,研究论文发展脉络
Chat Paper
正在生成论文摘要